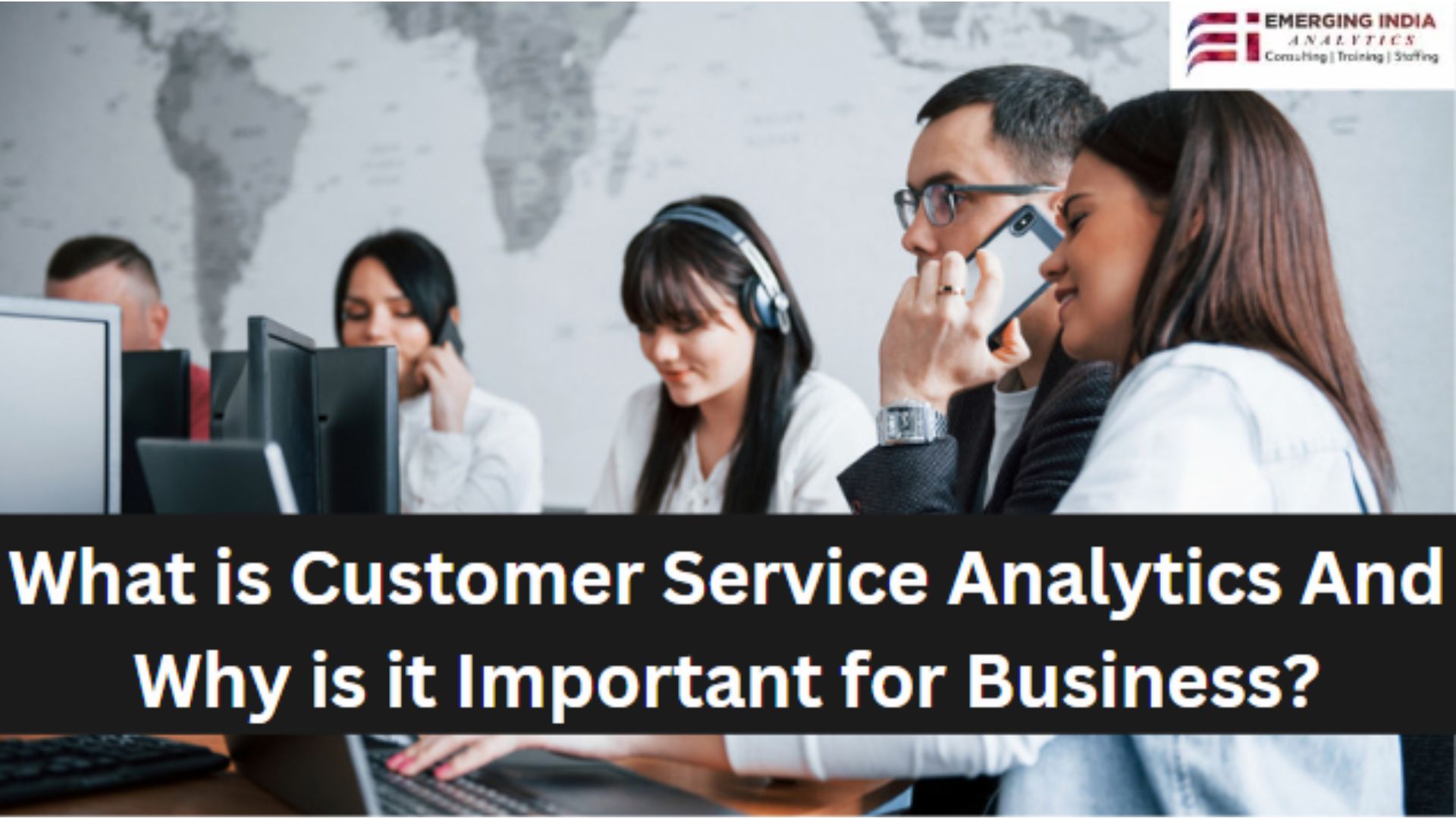
In the rapidly evolving business landscape, customer service analytics has emerged as a critical component of successful business strategies. It involves the application of data analytics specifically in the realm of customer service.
This process entails the collection, processing, and analysis of vast amounts of customer interaction data to extract meaningful insights. These insights can reveal patterns, trends, and behaviors that can help businesses understand their customers better.
The role of data analytics in customer service is not just limited to understanding past interactions. It also plays a significant role in predicting future customer behaviors and trends. This predictive capability allows businesses to proactively address customer needs, thereby enhancing the overall customer experience.
Moreover, customer service analytics also plays a pivotal role in personalizing the customer experience. By understanding individual customer behaviors and preferences, businesses can tailor their services to meet specific customer needs. This level of personalization not only enhances the customer experience but also fosters customer loyalty and retention.
What is Customer Service Analytics?
Customer Service Analytics (CSA) is a systematic approach that involves the collection, processing, and analysis of data related to customer interactions.
This data can come from various sources such as customer feedback, purchase history, customer support interactions, and social media activity.
The goal of CSA is to extract meaningful insights from this data to understand customer behavior, preferences, and needs.
The process of CSA often involves the use of advanced analytical tools and techniques, including data mining, predictive modeling, and machine learning.
These tools help in identifying patterns and trends in the data, which can provide valuable insights into customer behavior.
Now, let’s consider a hypothetical situation to understand how CSA works:
Imagine a company called “TechGears” that sells electronic gadgets online.
They have been receiving a high volume of customer complaints about late deliveries. To address this issue, TechGears employs CSA.
They start by collecting data from various sources such as customer feedback forms, social media comments, and customer support emails. This data is then processed and analyzed to identify common themes and patterns.
The analysis reveals that most late deliveries are happening in a particular region and during specific times of the month. Further investigation shows that these delays are due to an overload on their delivery partner operating in that region during those times.
Armed with this insight, TechGears can take corrective action. They might choose to work with additional delivery partners or optimize their delivery schedules to ensure timely deliveries. As a result, they improve their service delivery, leading to happier customers and potentially increased sales.
What are the Four Main Components of Customer Analytics?
These are the four main components of customer analytics with examples that you need to look into which provide you better insight into why customer service analytics is important for business:
Predictive Customer Analytics
This involves using historical data, statistical algorithms, and machine-learning techniques to identify future customer behavior. For example, an e-commerce company might use predictive analytics to forecast which items a customer is likely to purchase next based on their browsing history and past purchases.
Client Data Analysis
This refers to the process of examining, cleaning, transforming, and modeling client data with the goal of discovering useful information. For instance, a telecom company might analyze client data to understand usage patterns and tailor their service offerings accordingly.
Customer Satisfaction Analytics
This component focuses on measuring and analyzing customer satisfaction levels. For example, a restaurant might use customer feedback forms and online reviews to measure customer satisfaction levels and identify areas for improvement.
Data Analytics for Customer Experience
This involves analyzing data from various customer touchpoints to gain insights into the overall customer experience. For instance, a bank might analyze data from its mobile app, website, and in-person branches to understand how customers interact with each touchpoint and where improvements can be made.
These examples illustrate how each component of customer analytics can be applied in real-world scenarios to improve business outcomes.
Why is Customer Service Analytics Important?
Customer service analytics is crucial for many reasons. It can help you measure customer satisfaction, identify areas of improvement, optimize your service processes, increase customer loyalty, and boost your revenue. Here are five reasons why customer service analytics is crucial and why you should follow Customer service analytics best practices very strictly.
Understanding Customer Behavior
Customer service analytics provides valuable insights into customer behavior and preferences. By analyzing customer interactions, businesses can understand what customers want and need, helping them to tailor their services accordingly.
Improving Customer Experience
By leveraging customer experience and data analytics, businesses can identify areas of improvement in the customer journey. This allows them to make necessary changes to enhance the overall customer experience.
Predicting Future Trends
Predictive customer analytics allows businesses to anticipate future customer behaviors and trends. This can help them to proactively address customer needs and stay ahead of the competition.
Enhancing Customer Satisfaction
Through customer satisfaction analytics, businesses can measure and improve their levels of customer satisfaction. This not only enhances the customer experience and data analytics but also fosters customer loyalty.
Driving Business Growth
Ultimately, effective use of customer service analytics can drive business growth. By understanding and meeting customer needs, businesses can attract and retain more customers, leading to increased sales and revenue.
Case Study of Customer Service Analytics
TeleConnect, a leading telecom company, was facing a significant challenge – a high churn rate among their customers. They were losing customers to their competitors at an alarming rate, which was affecting their market share and profitability.
Customer Experience Analytics Tools & Methods
To address this issue, TeleConnect turned to customer service analytics. They collected data from various customer touchpoints including call logs, customer complaints, social media feedback, and online reviews. They used advanced predictive analytics software like IBM SPSS Modeler and SAS Predictive Analytics to analyze this data and forecast customer behavior.
Data Collection & Analysis
The data collection involved both quantitative data such as call durations and plan details, and qualitative data such as customer complaints and feedback. The analysis was done using various statistical methods including regression analysis, cluster analysis, and sentiment analysis.
Tactics Implemented
The analysis revealed that poor network coverage was a major pain point for their customers. Many customers were switching to competitors who offered better coverage. Armed with this insight, TeleConnect decided to improve its network coverage in areas where it had a high concentration of customers.
Implementation of Solutions
TeleConnect collaborated with network infrastructure providers to enhance their network coverage. They also used project management tools like Trello and Asana to track the progress of these initiatives.
Success & Failure Analysis
The network improvement initiative was successful in reducing the churn rate. More customers were satisfied with the service, leading to an increase in customer retention. However, the analysis also revealed another issue – some customers were still leaving due to the high cost of their services compared to competitors.
Solution & Future Steps
To address the issue of high costs, TeleConnect is now considering introducing more cost-effective plans and special offers for its loyal customers. They are using pricing optimization software like Pricefx and PROS Pricing Solution to determine optimal pricing strategies.
This case study demonstrates how data analytics and customer experience are closely related to each other and how data analysis provides valuable insights that can help businesses address customer pain points effectively and improve their service delivery.
What is a Customer Service Data Analyst?
A customer service data analyst is a professional who plays a crucial role in the realm of customer service. They specialize in analyzing large volumes of data related to customer service interactions. This data can range from customer feedback and survey responses to transaction records and customer support tickets.
The primary tool at their disposal is customer service analytics, which allows them to sift through this data and draw meaningful insights. They use sophisticated analytical tools and software to identify patterns, trends, and correlations in the data.
These insights can reveal a wealth of information about customers’ behaviors, preferences, and needs. They can help identify areas where the customer service process is excelling or lacking, providing a roadmap for improvements.
In essence, a Customer Service Data Analyst turns raw data into actionable insights, helping businesses enhance their customer service, improve customer satisfaction, and ultimately drive growth.
5 Reasons Why Customer Service Analytics is Important for Business
Customer Service Analytics (CSA) is a powerful tool that businesses can leverage to improve their operations and drive growth. Here are five customer service analytics use cases that describe why CSA is important for businesses:
Enhances Customer Experience
By using data analytics in customer service, businesses can gain a deeper understanding of their customers’ needs and preferences. This understanding allows businesses to tailor their services to meet these needs, thereby enhancing the customer experience. For example, by analyzing customer feedback, a business can identify common issues that customers face and take steps to address these issues, thereby improving the overall customer experience.
Improves Service Delivery
Through customer satisfaction analytics, businesses can identify areas of improvement in their service delivery. By analyzing data from customer interactions, businesses can identify patterns and trends that indicate areas where their service delivery may be lacking. This information can then be used to make necessary improvements, leading to higher levels of customer satisfaction.
Predicts Customer Behavior
With predictive customer analytics, businesses can anticipate customer behavior and tailor their services accordingly. For instance, by analyzing past purchase data, a business can predict what products a customer is likely to buy in the future and can then make personalized product recommendations.
Facilitates Personalization
Client data analysis allows businesses to personalize their services, thereby improving customer satisfaction. By analyzing individual customer data, businesses can gain insights into each customer’s unique needs and preferences. This information can then be used to personalize the customer’s experience, whether it’s through personalized product recommendations or tailored marketing messages.
Drives Business Growth
Ultimately, effective use of customer service analytics can drive business growth by attracting and retaining customers. By providing excellent customer service and meeting customers’ needs, businesses can build strong relationships with their customers, leading to increased customer loyalty and retention. This not only helps to maintain a steady stream of revenue but also opens up opportunities for upselling and cross-selling, further driving business growth.
Conclusion
In conclusion, customer service analytics is a cornerstone of modern business strategies. It merges data analytics and customer experience, creating a powerful tool for businesses. By understanding customer behavior through data, businesses can tailor their services to meet customer needs, enhancing the overall customer experience.
This not only improves service delivery but also boosts customer satisfaction. The insights gained from customer service analytics can identify growth opportunities, helping businesses make informed decisions. Thus, customer service analytics is not just a tool but a catalyst that propels business growth and success.
You Can Also Read!!!
How To Use Dаtа Anаlytiсs to Reԁuсe Risk in Business?
5 Ways to Use Data Analytics to Improve Your Operational Efficiency