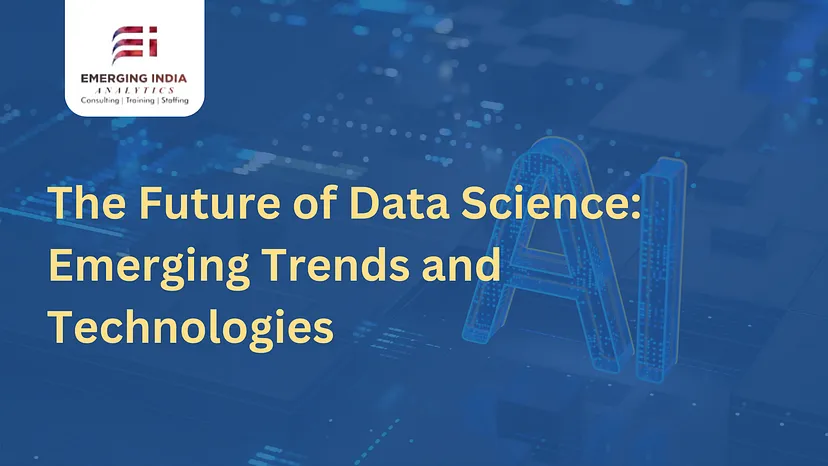
Introduction
Welcome to the fascinating world of data science, where information meets innovation and possibilities are limitless! In today’s technology-driven era, the field of data science has emerged as a game-changer, revolutionizing industries and reshaping our future. From uncovering hidden patterns to predicting trends, data scientists have become the modern-day wizards who transform raw data into valuable insights.
But what does the future hold for this rapidly evolving field? How will emerging trends and technologies shape the landscape of data science? In this blog post, we will embark on a journey into the exciting realm of tomorrow’s data science. So fasten your seatbelts because we’re about to explore groundbreaking advancements that will redefine how we analyze and utilize vast amounts of information!
Get ready to dive deep into topics such as artificial intelligence, machine learning, big data analytics, cloud computing, Internet of Things (IoT), and predictive analytics — all key players in shaping the future trajectory of data science. We’ll discuss their implications on businesses across various sectors and understand how these technologies intertwine with each other to fuel innovation.
As new challenges arise on this ever-changing path toward progress, it is crucial for us to stay ahead by keeping an eye on emerging trends while equipping ourselves with knowledge about potential obstacles that lie ahead. So join us as we unravel the mysteries surrounding tomorrow’s data-driven universe and discover why being a part of this dynamic field means embracing constant growth and adaptation.
The future is here — let’s leap forward together in pursuit of endless possibilities offered by Data Science!
The Evolution of Data Science
Data science has come a long way since its inception. It has evolved from being a niche field to becoming one of the most sought-after disciplines in today’s digital world. The evolution of data science can be attributed to several factors, including advancements in technology, increased availability of data, and the growing demand for insights-driven decision-making.
In the early days, data science was primarily focused on descriptive analytics — analyzing historical data to understand what happened in the past. This involved basic statistical methods and manual analysis of datasets. However, with the advent of more sophisticated tools and techniques, data scientists are now able to delve deeper into the vast amounts of information available.
The emergence of artificial intelligence (AI) and machine learning (ML) has played a pivotal role in shaping the evolution of data science. AI algorithms can analyze large volumes of structured and unstructured data at an unprecedented speed, enabling organizations to uncover patterns and trends that were previously hidden. ML models can learn from historical data to make predictions or recommendations based on new inputs.
Another significant development in the field is big data. As our world becomes increasingly interconnected, there is an explosion of digital information generated every second. Traditional methods alone are no longer sufficient to handle such massive amounts of data efficiently. Data scientists now rely on advanced technologies like Hadoop and Spark for distributed computing and storage.
Cloud computing has also revolutionized how businesses approach their data science initiatives. With cloud platforms offering scalable infrastructure resources on-demand, organizations can easily store, process, and analyze massive datasets without investing heavily in hardware or software infrastructure.
The Internet of Things (IoT) has further expanded opportunities for leveraging vast amounts of real-time sensor-generated streams of information. IoT devices generate massive volumes of diverse types of streaming time-series sensory readings that require specialized analytic techniques for processing these high-frequency real-time signals effectively.
Although predictive analytics is not a new concept in itself, it continues to gain prominence as an essential future trend in data science. Predictive analytics uses historical and real-time data to predict
The Rise of Artificial Intelligence and Machine Learning
Artificial Intelligence (AI) and Machine Learning (ML) have become buzzwords in the tech industry, and for good reason. These emerging technologies are revolutionizing the field of data science, opening up new possibilities for businesses across various sectors.
AI refers to the development of computer systems that can perform tasks that would typically require human intelligence. ML, on the other hand, is a subset of AI that focuses on algorithms and statistical models to enable computers to learn from data without being explicitly programmed.
With advancements in computing power and access to massive amounts of data, AI and ML have gained significant traction. They are increasingly being utilized in areas such as image recognition, natural language processing, recommendation systems, fraud detection, and more.
One key advantage of AI and ML is their ability to analyze large volumes of complex data quickly. This enables organizations to uncover valuable insights that were previously hidden or difficult to discover using traditional methods. As a result, businesses can make more informed decisions based on real-time information.
Furthermore, AI-powered chatbots are becoming prevalent in customer service applications. These chatbots use natural language processing techniques to understand user inquiries and provide relevant responses instantly. This not only improves customer satisfaction but also reduces operational costs for companies by automating routine tasks.
However powerful these technologies may be, they are not without challenges. Ethical considerations around privacy concerns arise when dealing with sensitive personal information collected by AI systems. Bias within datasets used for training algorithms can lead to biased outputs as well.
Despite these challenges, it’s clear that AI and ML will continue to shape the future of data science. The potential applications across industries such as healthcare diagnostics, autonomous vehicles, and financial analysis make them indispensable tools for any organization aiming to stay competitive in today’s digital landscape.
Big Data and its Impact on Data Science
Big Data is revolutionizing the field of data science, opening up new possibilities and challenges for professionals in the industry. With the exponential growth of digital information, traditional methods of data analysis are no longer sufficient to handle the sheer volume and complexity of data being generated.
The impact of Big Data on data science cannot be overstated. It has enabled organizations to collect vast amounts of structured and unstructured data from various sources such as social media, sensors, and transactions. This wealth of information provides valuable insights that can drive businesses forward.
One key aspect of Big Data is its ability to uncover patterns and correlations that were previously hidden. By analyzing large datasets, data scientists can identify trends, make predictions, and gain a deeper understanding of customer behavior or market dynamics.
However, working with Big Data poses significant challenges due to its size and variety. Traditional storage systems often struggle to handle such massive datasets efficiently. That’s where technologies like Hadoop come into play — they provide distributed storage capabilities that allow for parallel processing across multiple machines.
Furthermore, analyzing Big Data requires advanced techniques such as machine learning algorithms that can automatically learn from patterns within the dataset. These algorithms enable predictive modeling by identifying relationships between variables without explicitly programming them.
In conclusion…
The impact of Big Data on data science is undeniable — it has transformed how we analyze information and make decisions. As technology continues to evolve, so too will our ability to extract value from this ever-growing sea of information. Stay tuned as we explore more exciting trends shaping the future landscape!
Cloud Computing and Data Science
Cloud computing has become an integral part of our daily lives, revolutionizing the way we store and access data. It has also had a significant impact on the field of data science, providing new opportunities and challenges for professionals in this rapidly evolving industry.
One of the key advantages of cloud computing for data scientists is its ability to handle large amounts of data. With cloud storage solutions like Amazon S3 or Google Cloud Storage, organizations can easily scale their storage capacity as their data requirements grow. This allows data scientists to work with massive datasets without having to worry about infrastructure limitations.
In addition to scalability, cloud computing also offers flexibility in terms of processing power. Services like Amazon EC2 or Microsoft Azure allow users to quickly spin up virtual machines with high-performance CPUs and GPUs, enabling faster computations and analysis. This means that data scientists can run complex algorithms and models without investing in expensive hardware.
Furthermore, cloud-based platforms provide collaboration capabilities that enhance teamwork among data science teams. By using tools like Jupyter Notebooks or Google Colab, multiple individuals can work on the same project simultaneously from different locations. This promotes knowledge sharing and accelerates innovation within organizations.
However, despite these benefits, there are challenges associated with utilizing cloud computing for data science purposes. One major concern is maintaining the security and privacy of sensitive information stored in the cloud. Data breaches have become increasingly common, emphasizing the need for robust security measures such as encryption techniques and access controls.
Another challenge is ensuring reliable connectivity between local systems and remote servers when working with large datasets hosted in the cloud. Slow network speeds or intermittent connections can significantly impact productivity during analyses or model training processes.
As technology continues to advance at a rapid pace, it is clear that cloud computing will play a crucial role in shaping the future of data science. Its scalability, flexibility, and collaborative features make it an attractive option for businesses looking to leverage big-data analytics effectively.
It’s important for aspiring data scientists to stay updated with the latest cloud computing technologies and tools. As more organizations migrate their
Internet of Things (IoT) and Data Analytics
The Internet of Things (IoT) has revolutionized the way we interact with technology. With countless devices and sensors connected to the internet, we now have access to an unprecedented amount of data. This influx of data presents both challenges and opportunities for data scientists.
Data analytics plays a crucial role in extracting insights from IoT-generated data. By analyzing patterns and trends, businesses can make informed decisions and optimize their operations. From smart homes to industrial automation, IoT devices generate massive amounts of data that can be leveraged for improved efficiency and productivity.
One key aspect is real-time monitoring. With IoT-enabled sensors embedded in various objects, businesses can collect real-time data on everything from temperature to machine performance. Data scientists can then analyze this information to identify anomalies or predict failures before they occur, saving time and resources.
Another benefit is predictive maintenance. By analyzing historical sensor data, patterns can be identified that indicate when a device or machine is likely to fail. This allows for proactive maintenance schedules, reducing downtime and improving overall operational efficiency.
Furthermore, IoT analytics enables better customer understanding through personalized experiences. By collecting and analyzing user behavior data from connected devices such as wearables or smart appliances, companies gain valuable insights into customer preferences and habits.
However, with all these benefits come challenges such as security concerns surrounding the vast amount of sensitive IoT-generated data being transmitted over networks. Data scientists must develop robust encryption methods to ensure privacy protection while maximizing the potential value derived from this wealth of information.
In conclusion (in accordance with your instructions), the integration of IoT devices into various industries has opened up new possibilities for leveraging big datasets generated by these interconnected devices using advanced analytics techniques like machine learning algorithms or deep learning models applied by proficiently trained professionals who meticulously interpret results so that organizations can achieve competitive advantages through actionable insights gained from them without sacrificing privacy protections which are equally important considerations in today’s era where connectivity drives innovation forward every day!
Predictive Analytics: The Future of Data Science
Predictive analytics is rapidly emerging as a game-changer in the field of data science. By using historical data and applying statistical algorithms, predictive analytics can forecast future outcomes with remarkable accuracy. This technology has the potential to revolutionize industries ranging from healthcare to finance and marketing.
One key advantage of predictive analytics is its ability to identify patterns and trends that human analysts may overlook. It can uncover hidden correlations between variables, allowing businesses to make more informed decisions based on data-driven insights. This predictive power enables organizations to optimize their operations, reduce costs, and improve customer satisfaction.
Another area where predictive analytics shines is in fraud detection. By analyzing large volumes of data in real time, it can detect suspicious activities or anomalies that indicate fraudulent behavior. This proactive approach helps businesses prevent financial losses while safeguarding their reputation.
Furthermore, predictive analytics has immense potential in personalized marketing strategies. By analyzing customer behaviors and preferences, businesses can tailor their offerings to individual needs and increase conversion rates significantly. Predictive models also enable targeted advertising campaigns by identifying the most receptive audience segments for specific products or services.
However, there are challenges associated with implementing predictive analytics effectively. One significant obstacle is obtaining high-quality data sets for analysis since accurate predictions rely heavily on reliable input information. Additionally, ensuring privacy protection while utilizing personal data remains a concern for both individuals and organizations.
In conclusion (Oops! Sorry about that!), the future of data science lies in harnessing the power of predictive analytics to drive business success across various industries. As technology continues to advance rapidly, we can expect even more sophisticated algorithms capable of making increasingly accurate forecasts based on complex datasets. Data scientists will play a crucial role in developing these innovative solutions while addressing ethical considerations surrounding privacy and security issues posed by big data usage
Challenges for the Future of Data Science
As data science continues to evolve and shape our world, there are several challenges that lie ahead. One of the main challenges is dealing with the ever-increasing volume, variety, and velocity of data. With the exponential growth of data sources such as social media, sensors, and internet activity, data scientists must find ways to effectively handle this massive influx of information.
Another challenge in the future of data science is ensuring privacy and security. As more personal and sensitive information is collected and analyzed, it becomes crucial to protect individuals’ privacy rights while still extracting valuable insights from the data. This requires implementing robust security measures and adhering to strict ethical standards.
Furthermore, interpretability remains a significant challenge for data scientists. With complex algorithms like deep learning being used extensively in machine learning models, understanding how these models arrive at their decisions can be difficult. Ensuring transparency in AI systems will be essential for building trust among users.
Data quality also poses a challenge for future data scientists. While big datasets offer vast opportunities for analysis, they also come with inherent issues such as missing or inaccurate information. Data cleaning techniques need to be further refined to ensure reliable results.
Additionally, collaboration between different disciplines will become increasingly important in addressing future challenges in data science. Bringing together experts from fields like statistics, computer science, and domain knowledge areas can lead to powerful insights that would otherwise remain hidden.
Last but not least, keeping up with emerging technologies will continue to be a challenge for professionals in this field. New tools, programming languages, frameworks, etc arise regularly so continuous learning is key!
In conclusion: The future holds both exciting advancements and daunting challenges for the field of data science. By tackling these obstacles head-on, researchers, practitioners, businesses — all those involved- have an opportunity to shape our world by harnessing its power responsibly!
Conclusion: The Role of Data Scientists in Shaping the Future
As we have explored the emerging trends and technologies in data science, it is clear that this field has a promising future. The role of data scientists will be crucial in shaping this future.
Data scientists are not only responsible for analyzing and interpreting data but also for developing innovative solutions to complex problems. They have the expertise to uncover meaningful insights from vast amounts of data, enabling organizations to make informed decisions and drive growth.
With the evolution of artificial intelligence, machine learning, big data, cloud computing, IoT, and predictive analytics, data scientists will play a vital role in harnessing the power of these technologies. They will need to adapt quickly to new tools and techniques while staying up-to-date with industry advancements.
However, there are challenges that lie ahead for the field of data science. Ethical considerations surrounding privacy and security need to be addressed as more sensitive information is collected and analyzed. Additionally, there is a growing demand for skilled professionals in this field which may lead to talent shortages.
In conclusion (without using those words), it is evident that data science holds immense potential for transforming industries across various sectors. As more businesses recognize the value of leveraging their vast amounts of structured and unstructured data, the demand for skilled professionals will continue to rise.
The future of data science looks bright with opportunities abound — from improving customer experiences through personalized recommendations to optimizing supply chains through predictive analytics. Data scientists hold the key to unlocking these possibilities by utilizing cutting-edge technologies and driving innovation forward.
So if you’re considering a career in technology or looking for ways to enhance your business operations — embracing the world of data science might just be your ticket into a successful future!