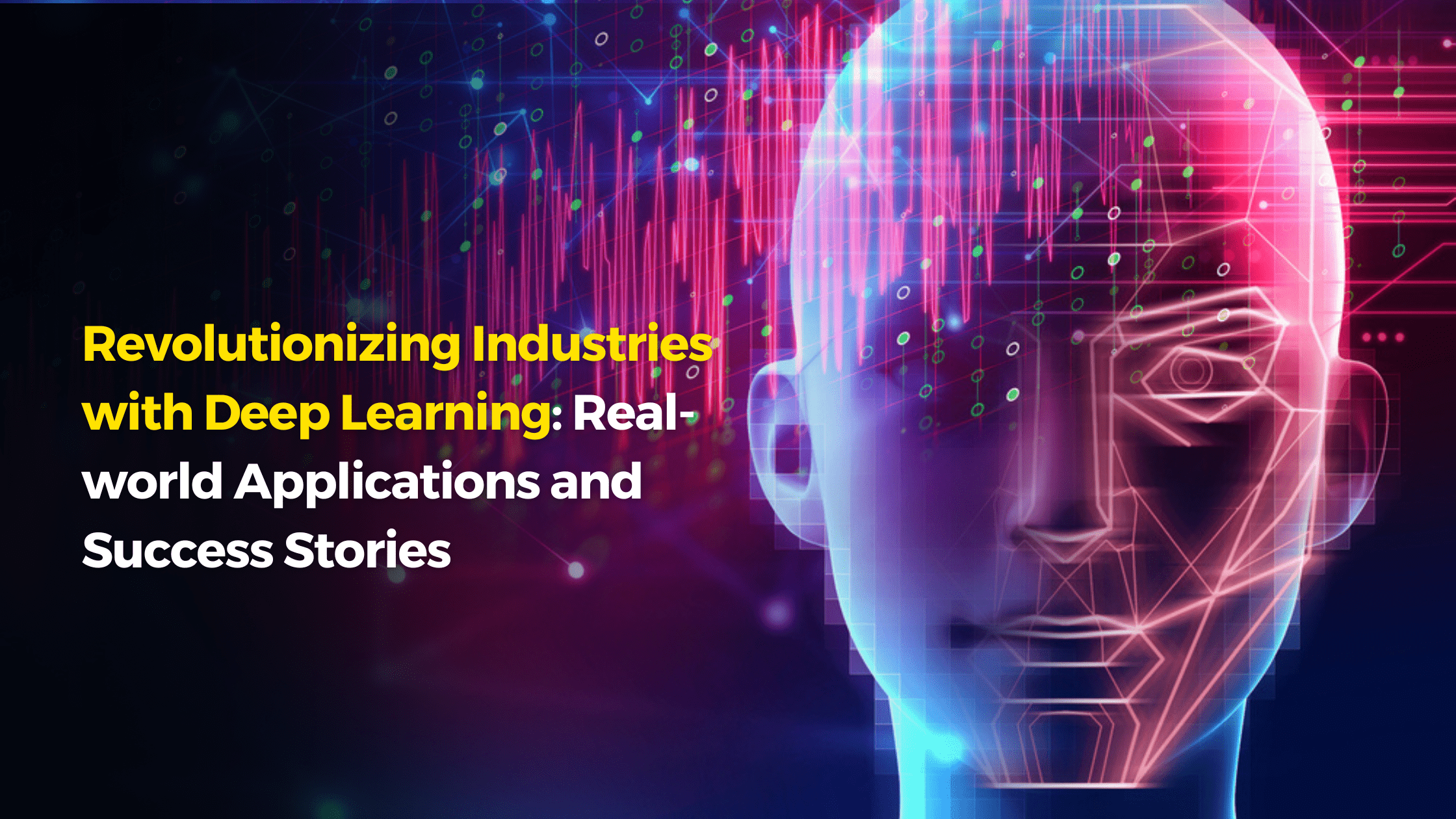
Welcome to a world where machines outperform humans in various tasks, revolutionizing industries one breakthrough at a time. Deep learning, the cutting-edge technology behind this remarkable achievement, has taken the world by storm with its ability to analyze vast amounts of data and make accurate predictions. From healthcare to finance, from manufacturing to transportation – deep learning is transforming every sector it touches. In this blog post, we will explore the real-world applications of deep learning and dive into inspiring success stories that showcase how this powerful technology is shaping our future. So buckle up and get ready for an exhilarating journey into the realms of artificial intelligence as we unveil the game-changing impact of deep learning on industries worldwide!
Introduction to Deep Learning
Deep learning is a subset of artificial intelligence that has been gaining widespread attention in recent years due to its ability to solve complex problems and make accurate predictions. It involves training neural networks, which are algorithms modeled after the human brain, with large amounts of data to recognize patterns and make decisions.
One of the key features that sets deep learning apart from traditional machine learning techniques is its use of multiple layers in the neural network. These layers allow for a hierarchical representation of data, where each layer learns more abstract features from the previous one. This enables deep learning models to handle highly complex and unstructured data such as images, speech, and natural language.
The concept of deep learning has been around since the 1950s, but it wasn’t until recently when advancements in computing power and access to big data made it possible to train these models effectively. Today, deep learning is being used in various industries such as healthcare, finance, retail, transportation, and many others. Let’s take a closer look at some real-world applications and success stories that showcase how deep learning is revolutionizing these industries.
1. Healthcare: Deep Learning for Medical Image Analysis
Medical imaging plays a crucial role in diagnosing diseases and planning treatments. However, interpreting medical images can be time-consuming for doctors and prone to human error. Deep learning has shown promising results in automating this process by accurately identifying abnormalities on medical scans such as X-rays, MRIs, CT scans, etc.
For instance, researchers at Stanford University developed an
Understanding the Basics of Neural Networks
Neural networks are a fundamental component of deep learning, which has revolutionized industries across the board with its powerful capabilities. These artificial neural networks (ANN) are models inspired by the structure and function of the human brain, designed to process large amounts of data and make complex decisions. In this section, we will delve deeper into the basics of neural networks and understand how they work.
The foundation of a neural network lies in its basic unit called a neuron. This is an interconnected node that receives input data from other neurons, performs calculations with them, and produces an output. Multiple neurons come together to form layers within a neural network. The first layer takes in raw data as input and passes it on to the next layer for further processing. Each subsequent layer becomes more specialized in understanding patterns within the data until it reaches the final output layer.
To train a neural network, we use a process called backpropagation where we feed labeled training data into the network multiple times. During each iteration, the network adjusts its internal parameters based on how well it performed compared to the expected output. This enables it to continuously improve its ability to recognize patterns in new data.
One key aspect of neural networks is their ability to learn through experience or past examples rather than relying solely on explicit programming instructions. This allows them to tackle complex problems that would be difficult or impossible for traditional computer programs.
There are different types of neural networks used for various purposes such as image recognition, natural language processing, time-series prediction, etc.
Real-world Applications of Deep Learning
Deep learning, a subfield of artificial intelligence, has gained immense popularity in recent years due to its ability to process large amounts of data and extract meaningful insights. This revolutionary technology has found numerous applications in various industries, transforming the way businesses operate and improving efficiency and accuracy in decision making.
In this section, we will explore some real-world applications of deep learning that have revolutionized industries across the globe.
1) Healthcare:
One of the most significant applications of deep learning is in the healthcare industry. With the help of advanced algorithms and neural networks, medical professionals can now accurately diagnose diseases and predict treatment outcomes. Deep learning models can analyze vast amounts of patient data such as medical records, lab results, imaging scans, and genetic information to identify patterns that may not be visible to human eyes. This has led to improved disease diagnosis rates and personalized treatment plans for patients.
For example, Google’s deep learning model was able to detect diabetic retinopathy (a leading cause of blindness) with 97% accuracy by analyzing retinal images. Another success story is IBM Watson Health’s use of deep learning for cancer treatment recommendations based on patient data analysis.
2) Finance:
The finance industry generates an enormous amount of data every day from stock market fluctuations to customer transactions. Deep learning techniques have enabled financial institutions to process this data quickly and accurately for tasks like fraud detection, risk assessment, investment predictions, and credit scoring. By identifying hidden patterns in financial data, deep learning models can make more informed decisions than traditional
Healthcare and Medicine
The healthcare and medicine industry is one of the most essential sectors in our society, as it is responsible for maintaining the health and well-being of individuals. With the constant advancements in technology, deep learning has emerged as a game-changing tool in this field. It has revolutionized the way medical professionals diagnose diseases, make treatment plans, and monitor patient progress.
One of the significant applications of deep learning in healthcare is medical imaging analysis. Traditional methods of analyzing medical images were time-consuming and prone to errors. However, with deep learning techniques such as convolutional neural networks (CNNs), radiologists can now quickly and accurately detect abnormalities in X-rays, MRI scans, CT scans, and other medical images. This has significantly improved diagnostic accuracy, leading to better treatment outcomes.
Another area where deep learning has made a considerable impact is drug discovery and development. The process of developing new drugs is time-consuming and expensive. However, with deep learning algorithms that can analyze vast amounts of data from various sources such as scientific literature, clinical trials, and chemical compounds databases; researchers can now identify potential drug candidates more efficiently. This not only speeds up the drug development process but also reduces costs significantly.
Deep learning has also played a crucial role in personalized medicine – an approach that takes into account an individual’s genetic makeup, lifestyle choices, environment factors when making treatment plans. With deep learning models trained on large datasets containing information about patients’ genetics and medical history; doctors can now predict which treatments will be most effective for specific patients
Finance and Banking
Finance and Banking is one of the industries that has been greatly impacted by the rise of Deep Learning technology. In this section, we will explore how financial institutions are utilizing Deep Learning to improve their processes, enhance customer experience, and drive business growth.
1. Fraud Detection:
Fraud detection has always been a major concern for banks and financial institutions. With the increase in online transactions and digital payments, fraudsters have found new ways to exploit vulnerabilities in the system. Deep Learning techniques such as anomaly detection, predictive modeling, and behavioral analysis are being used to identify fraudulent activities in real-time. This not only helps in preventing financial losses but also ensures a secure environment for customers.
2. Risk Management:
Traditional risk management models relied heavily on historical data and statistical analysis which were often limited in their ability to predict future trends accurately. By using Deep Learning algorithms, banks can analyze large volumes of structured and unstructured data from various sources including social media, news articles, market trends, etc., to detect patterns and make more accurate risk assessments. This enables them to mitigate potential risks and make better-informed decisions.
3. Personalized Customer Experience:
Personalization has become key in the highly competitive banking industry where customers expect tailored products and services based on their individual needs. With Deep Learning, banks can analyze customer behavior patterns from past transactions and interactions with the bank’s website or app to understand their preferences better. This information can then be used to offer personalized recommendations for financial products or services that best suit each customer
Retail and E-commerce
Retail and e-commerce have been greatly impacted by the advancements in deep learning technology. From improving customer experience to optimizing supply chain management, this powerful tool has revolutionized the way these industries operate.
One of the major applications of deep learning in retail and e-commerce is in personalized marketing and recommendation systems. Using deep learning algorithms, companies are able to analyze vast amounts of data on customer behavior, preferences, and purchase history to create personalized product recommendations. This not only improves the overall shopping experience for customers but also increases sales for businesses.
In addition to personalization, deep learning has also greatly improved inventory management for retailers. Traditional methods of forecasting demand and managing inventory can be time-consuming and often lead to inaccurate predictions. With deep learning techniques such as neural networks, retailers can now analyze a wide range of data points including historical sales data, current market trends, weather patterns, and social media activity to make more accurate demand forecasts. This helps businesses avoid overstocking or understocking products, ultimately leading to cost savings and increased efficiency.
Another significant impact of deep learning in retail is its ability to detect fraud and prevent losses for both online and brick-and-mortar stores. Deep learning algorithms can quickly analyze large volumes of transactions in real-time and identify suspicious activities such as fraudulent purchases or stolen credit card information. This enables businesses to take immediate action before any damage occurs.
Apart from these operational benefits, deep learning has also enhanced the overall customer experience with advanced chatbots powered by natural language processing (NLP).
Automotive Industry
The automotive industry has been at the forefront of technological advancements in recent years, with self-driving cars, electric vehicles, and advanced driver assistance systems becoming increasingly prevalent. One technology that has played a crucial role in these innovations is deep learning. Deep learning algorithms have revolutionized the automotive industry by enabling machines to learn from vast amounts of data and make complex decisions without explicit programming.
One of the most significant applications of deep learning in the automotive sector is autonomous driving. Companies like Tesla and Waymo have made considerable progress in developing self-driving vehicles that can navigate through traffic, recognize road signs and signals, and avoid obstacles using deep learning algorithms. These algorithms use a combination of sensors such as cameras, lidar, radar, and ultrasonic sensors to gather real-time data about their surroundings. This data is then fed into deep neural networks that process it to make decisions about steering, braking, accelerating, and other critical functions.
Deep learning has also transformed how engineers design cars. With traditional methods, designing a car’s shape could take months or even years. However, with generative adversarial networks (GANs), designers can quickly generate multiple designs based on specific criteria such as aerodynamics or aesthetics. GANs are trained on thousands of existing car designs to learn the underlying patterns and generate new designs that meet the desired specifications. This process not only saves time but also opens up possibilities for more creative and efficient vehicle designs.
Another area where deep learning has had a significant impact is in predictive maintenance for automobiles.
Education Sector
The education sector has always been at the forefront of embracing new technologies and innovative approaches to enhance learning outcomes. With the rise of deep learning, this trend has only accelerated as educational institutions around the world are leveraging its capabilities to revolutionize the way students learn and teachers teach.
One of the most significant applications of deep learning in education is personalized learning. By utilizing sophisticated algorithms and data analysis techniques, educators can now create tailored lesson plans and activities for each student based on their individual strengths, weaknesses, and learning style. This personalized approach not only improves engagement but also leads to better academic performance.
Another area where deep learning is making a significant impact is in language translation. With students from diverse backgrounds studying together, language barriers can often hinder effective communication and collaboration. However, with advancements in natural language processing (NLP), deep learning models can now accurately translate text from one language to another in real-time. This technology not only makes classrooms more inclusive but also prepares students for a globalized workforce.
Assessment and grading have always been essential components of the education system, but they are often time-consuming and prone to errors. Deep learning-powered assessment tools can automatically grade assignments and exams using advanced scoring algorithms, freeing up valuable time for teachers to focus on providing feedback and support to their students. These tools also offer real-time insights into student performance, allowing educators to identify areas that need improvement quickly.
Apart from improving traditional teaching methods, deep learning is also paving the way for innovative teaching tools such as virtual tutors or
Success Stories of Companies Utilizing Deep Learning
Deep learning, a subset of artificial intelligence (AI), has been making waves in various industries with its ability to analyze large amounts of data and identify patterns and relationships that were previously unattainable. By mimicking the way the human brain processes information, deep learning algorithms have proven to be incredibly powerful in solving complex problems and revolutionizing industries. In this section, we will explore some success stories of companies that have successfully implemented deep learning technology in their operations.
1. Google: Image Recognition
Google has been at the forefront of using deep learning to improve its products and services. One notable application is its image recognition technology which is used in Google Photos and Google Lens. Using deep learning algorithms, Google can accurately identify objects, people, and even text within images uploaded by users. This has greatly improved user experience by making it easier to search for specific photos or translate foreign languages through the camera lens.
2. Netflix: Personalized Recommendations
With millions of subscribers worldwide, Netflix generates an immense amount of data on user preferences, watching habits, and ratings. To provide personalized recommendations for each user based on this data, Netflix utilizes deep learning algorithms to analyze viewing history and make predictions about what they might enjoy watching next. This has significantly increased customer satisfaction and retention rates for the streaming giant.
3. Walmart: Inventory Management
With over 11,000 stores worldwide, managing inventory efficiently is crucial for Walmart’s operations. In order to optimize their supply chain management process, Walmart turned to deep learning technology
Google’s use of DeepMind in its services
Google is known for its innovative use of technology to improve their services and products. One of the most significant advancements that Google has made in recent years is the incorporation of Deep Learning through their partnership with DeepMind, an artificial intelligence company that Google acquired in 2014.
DeepMind’s cutting-edge technologies have revolutionized how Google operates and delivers its services to users worldwide. By using advanced machine learning algorithms and neural networks, DeepMind has helped enhance various aspects of Google’s services, such as search engine results, voice recognition, image processing, and more.
One notable example of DeepMind’s integration into Google’s services is the improvement of the Google Translate app. With Deep Learning algorithms, the app can now translate between languages more accurately and efficiently than ever before. This application uses neural networks to analyze patterns in different languages’ grammatical structures to provide accurate translations in real-time.
Another revolutionary innovation that DeepMind brought to Google was the development of AlphaGo, an AI program capable of playing Go – a complex board game with trillions of possible moves. In 2016, AlphaGo famously defeated Lee Sedol – one of the world’s best Go players – in a five-game match. This achievement demonstrated how powerful Deep Learning can be when applied correctly.
Furthermore, DeepMind has also helped improve user experience on YouTube by implementing algorithms that recommend videos based on a user’s viewing history and preferences. These suggestions help users discover new content they may enjoy while keeping them engaged on the platform for longer periods
Amazon’s use of deep learning for product recommendations
Amazon is a pioneer in the use of deep learning for product recommendations. The company has been utilizing this cutting-edge technology to provide highly personalized and relevant product suggestions to its customers. With over 197 million active users worldwide, Amazon’s recommendations have become an integral part of its customer experience and have contributed significantly to the company’s success.
So, how does Amazon use deep learning for product recommendations? Let’s dive into the details.
1. Understanding Customer Behavior: At the core of Amazon’s recommendation system lies deep learning models that are trained on vast amounts of data collected from its customers. These models analyze a variety of factors such as purchase history, browsing behavior, search queries, and even mouse movements to understand each customer’s preferences and interests. This allows Amazon to create a comprehensive profile for each user, enabling them to make accurate predictions about what products they are most likely to be interested in purchasing.
2. Collaborative Filtering: Another crucial aspect of Amazon’s recommendation system is collaborative filtering, which involves analyzing patterns among different users’ behaviors. By analyzing their interactions with products and purchases, deep learning algorithms can identify similar interests among various user groups and recommend products accordingly. This approach not only helps in generating more precise recommendations but also enables cross-selling by suggesting complementary products that users may not have considered before.
3. Natural Language Processing: In recent years, Amazon has also incorporated natural language processing (NLP) techniques into its recommendation engine using deep learning algorithms. NLP allows computers to understand human language better and
Tesla’s self-driving cars powered by deep learning algorithms
Tesla’s self-driving cars have been making headlines since their introduction, promising to revolutionize the automotive industry. One of the key components that makes this possible is deep learning algorithms. These powerful algorithms are at the core of Tesla’s autonomous driving technology, allowing the vehicles to make decisions based on real-time data and environmental conditions.
At its most basic level, deep learning involves training a neural network with large amounts of data to recognize patterns and make predictions. This technology has proven to be incredibly effective in many industries, but it is particularly well-suited for self-driving cars.
The first step in creating a self-driving car powered by deep learning algorithms is collecting vast amounts of data. Tesla’s fleet of vehicles captures massive amounts of information every day through cameras, sensors, radar, and other sources. This data includes images, video footage, audio recordings, and more – all providing valuable insights about how humans interact with their environment while driving.
Once this data has been collected, it is fed into a neural network that has been specifically designed for autonomous driving tasks. The network then trains itself by analyzing patterns in the data and adjusting its weights accordingly – much like how our brains learn new things through experience.
As the neural network continues to train on more and more data, it becomes increasingly accurate at detecting different objects such as cars, pedestrians, traffic signals, and road signs. It also learns how these objects move within their surroundings and can predict potential outcomes based on previous experiences.
One of the most significant advantages of using
–
The use of deep learning has revolutionized various industries, bringing about unprecedented levels of efficiency and innovation. In this section of the blog, we will explore some real-world applications and success stories that demonstrate how deep learning is transforming different sectors.
1. Healthcare:
Deep learning has made a significant impact in the healthcare industry by providing accurate diagnosis and treatment solutions. For instance, Google’s DeepMind project uses deep learning algorithms to detect eye diseases like diabetic retinopathy with an accuracy level comparable to human doctors. This technology can help save time and improve patient outcomes by detecting diseases at an early stage.
In addition, deep learning algorithms are also being used for medical image analysis, such as MRI scans and X-rays, to assist doctors in making more precise diagnoses. This not only reduces the chances of misdiagnosis but also improves the speed of diagnosis, allowing for faster treatment.
2. Automotive Industry:
The automotive industry has also embraced the power of deep learning to enhance their products’ performance and safety features. With the rise of self-driving cars, companies like Tesla, Waymo, and Uber have heavily invested in deep learning technologies to develop advanced driver assistance systems (ADAS).
Deep learning algorithms enable vehicles to recognize traffic signs, pedestrians, other vehicles on the road, and make decisions based on that information in real-time. This technology has shown promising results in reducing accidents caused by human error.
3. Retail:
Retail businesses are leveraging deep learning for various tasks such as inventory management, customer service chatbots,