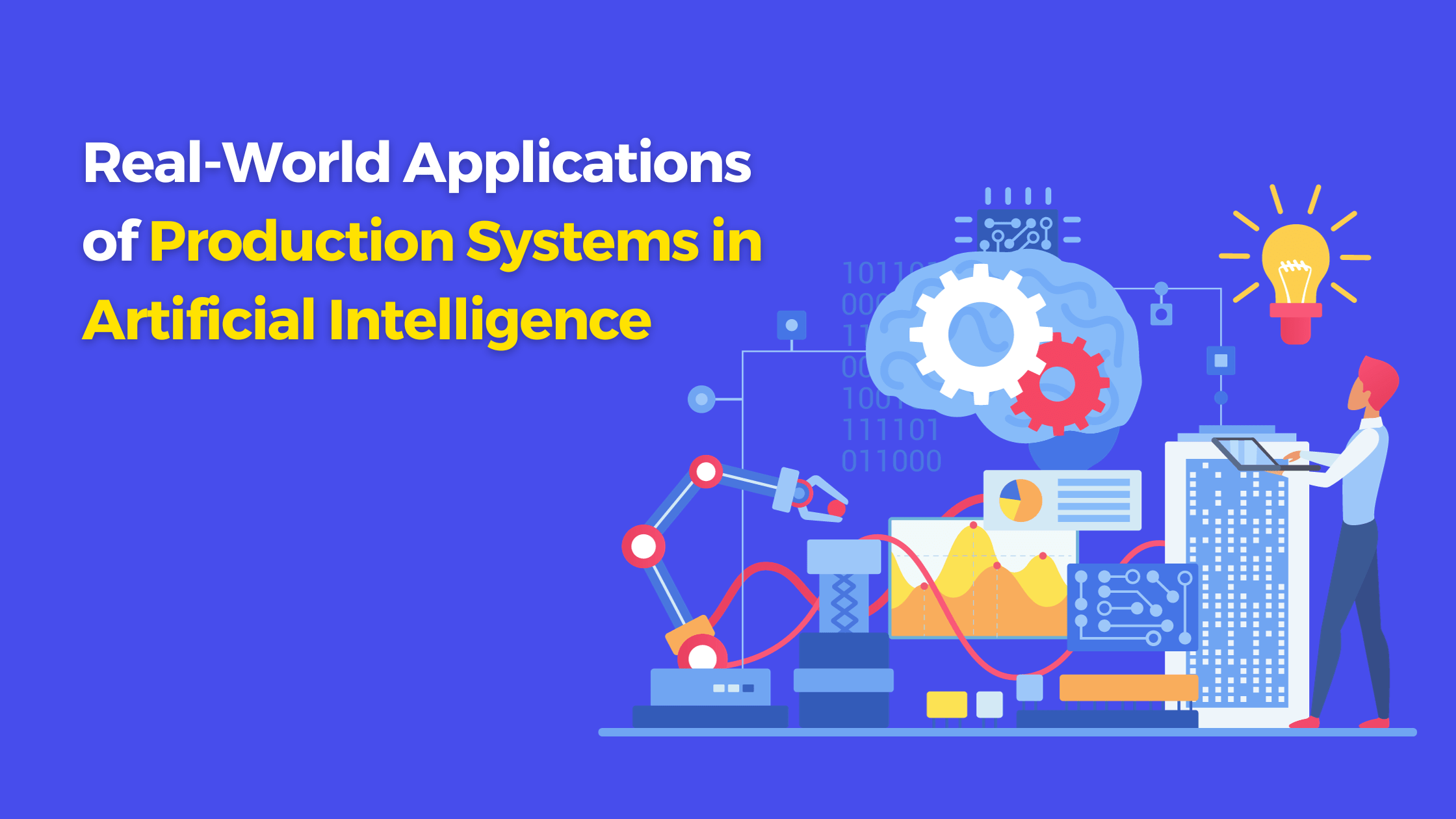
Are you curious about how artificial intelligence is transforming various industries? Wondering how production systems work in the realm of AI and what real-world applications they have? Look no further! In this blog post, we will delve into the exciting world of production systems in artificial intelligence to uncover their practical uses. From streamlining manufacturing processes to enhancing customer experiences, join us on a journey where cutting-edge technology meets real-life impact. Brace yourself for insightful examples and fascinating insights that will leave you hungry for more knowledge on the subject. Let’s dive right in!
Introduction to Production Systems in Artificial Intelligence
Production systems are a type of knowledge-based system that have been widely used in the field of artificial intelligence (AI) since their development in the 1960s. They are rule-based systems designed to simulate human problem-solving and reasoning processes, making them an ideal tool for solving complex problems and decision-making tasks.
In this section, we will provide a comprehensive overview of production systems, including their definition, components, and how they work. We will also discuss the key features that make them useful for real-world applications in artificial intelligence.
Definition of Production Systems
A production system is an AI software architecture that stores knowledge as rules within a knowledge base. These rules consist of condition-action statements that define how the system should behave or react when presented with certain conditions or situations. In other words, they represent a set of instructions that guide the system’s behavior and decision-making process.
Components of Production Systems
1. Knowledge Base:
The knowledge base is the central component of a production system where all the rules and facts are stored. It contains both declarative knowledge (information about objects, events, and relationships) and procedural knowledge (how to perform specific tasks). The system uses this information to make decisions by matching it with incoming data or queries.
2. Working Memory:
The working memory is responsible for storing temporary data during problem-solving processes. It acts as a buffer between the knowledge base and external environment by holding relevant information needed by production rules to make decisions.
Understanding the Components of a Production System
Production systems are an integral part of Artificial Intelligence (AI) and are widely used in real-world applications. These systems are designed to mimic the decision-making processes of human experts by utilizing rules, knowledge, and data to achieve a specific goal. In this section, we will delve deeper into the components that make up a production system and how they work together to drive intelligent decision-making.
1. Knowledge Base:
The knowledge base is the foundation of a production system and contains all the information or rules necessary for making decisions. It can be represented in various forms such as if-then rules, frames, semantic networks, or ontologies. This knowledge is acquired from human experts or extracted from data using techniques like machine learning or natural language processing. The accuracy and completeness of the knowledge base greatly impact the performance of the production system.
2. Working Memory:
Working memory acts as a temporary storage unit for information during problem-solving in a production system. It holds relevant facts about the current state of the problem being solved and provides input to rule-based reasoning mechanisms. Working memory is constantly updated based on new inputs from sensors, user interactions, or other external sources.
3. Inference Engine:
The inference engine is responsible for applying logical reasoning on the knowledge base stored in working memory to generate new conclusions or actions based on predefined rules. It uses pattern-matching algorithms to match conditions specified in if-then rules against facts stored in working memory and triggers corresponding actions when a match is found.
4. Production Rules:
Real-World Examples of Production Systems in AI
Production systems in artificial intelligence (AI) have been widely used in various industries and fields, showcasing their versatility and effectiveness in solving complex problems. In this section, we will discuss some real-world examples of production systems in AI, demonstrating how they have been applied to improve processes, decision-making, and overall performance.
1. Healthcare: One of the most promising applications of production systems in AI is in the healthcare industry. With a vast amount of patient data available, it can be challenging for doctors to make accurate diagnoses and treatment plans. Production systems help by analyzing patient data and providing recommendations based on previous cases or medical guidelines. For example, IBM’s Watson supercomputer uses a production system to assist doctors with cancer treatment decisions. It analyzes patient data, medical literature, and treatment options to provide personalized recommendations for each patient.
2. Manufacturing: In manufacturing industries where efficiency is crucial for success, production systems are being utilized to optimize processes and minimize downtime. For instance, General Motors uses a hybrid production system called “Intelligent Control System” (ICS), which combines rule-based reasoning with neural networks to predict machine failures before they happen. This allows them to schedule maintenance proactively and avoid costly breakdowns.
3. Finance: The finance sector has also seen the benefits of using production systems in AI to handle large amounts of financial data efficiently. Banks use these systems for fraud detection by identifying patterns that indicate fraudulent activities or transactions and raising red flags for further investigation. Additionally, investment firms utilize them for portfolio management
– Robotics and Automation
Robotics and automation have become integral components of modern production systems, revolutionizing the way we manufacture goods. In this section, we will delve into how robotics and automation are utilized in real-world applications of artificial intelligence (AI) within production systems.
Robots have been used in manufacturing for decades, but recent advancements in AI have greatly increased their capabilities. One of the main benefits of using robots in production is their ability to perform repetitive tasks with high precision and consistency, thereby reducing human error. This has led to significant improvements in efficiency and productivity.
In addition to traditional industrial robots, collaborative robots or “cobots” have emerged as a prominent technology in production systems. These are designed to work alongside humans rather than replace them. Cobots use advanced sensors and AI algorithms to adapt to changing environments and interact safely with humans, making them ideal for tasks that require human supervision or dexterity.
One example of the application of robotics and AI is in automated material handling systems. These systems use robots equipped with vision sensors to identify objects on a conveyor belt and sort them accordingly. The robots can also communicate with each other through a centralized control system, allowing for efficient coordination and optimization of the production process.
Another common application is robotic assembly lines, where robots equipped with cameras and sensors can autonomously assemble parts without human intervention. This reduces labor costs while increasing accuracy and speed.
In addition to physical tasks, AI-powered software bots are increasingly being used for automating administrative tasks such as data entry, invoice processing,
– Personalized Recommender Systems
Personalized Recommender Systems, also known as recommendation engines, are a type of production system that uses artificial intelligence (AI) to provide personalized recommendations to users. These systems have become increasingly popular in recent years due to their ability to enhance user experience and drive customer engagement for businesses.
One of the main applications of personalized recommender systems is in the field of e-commerce. With the rise of online shopping, it has become crucial for businesses to offer personalized recommendations to their customers in order to increase sales and retain loyal customers. These recommendation engines use AI algorithms and techniques such as collaborative filtering and content-based filtering to analyze customer data, including browsing history, purchase history, and preferences, in order to suggest relevant products or services that match their interests.
Another area where personalized recommender systems are widely used is in the entertainment industry. Streaming platforms like Netflix and Spotify rely heavily on these systems to provide customized viewing and listening experiences for their users. By analyzing user behavior such as watch or listen history, ratings, and reviews, these recommendation engines can make accurate predictions about what types of movies or music a particular user would enjoy. This not only enhances user satisfaction but also helps these companies with content curation and retention strategies.
Moreover, personalized recommender systems are also being utilized in healthcare for patient treatment recommendations. With access to vast amounts of medical data such as patient records, symptoms, diagnoses, medications, etc., AI-powered recommendation engines can assist doctors in making more effective treatment decisions by suggesting potential diagnosis options or medication
– Virtual Assistants and Chatbots
Virtual Assistants and Chatbots have become increasingly popular in recent years, thanks to the advancements in artificial intelligence technology. These intelligent systems are designed to interact with users in a conversational manner, providing them with personalized assistance and information.
One of the most well-known examples of virtual assistants is Apple’s Siri. This AI-powered assistant can perform tasks such as setting reminders, making phone calls, or searching for information on the internet, all through voice commands. Similarly, Amazon’s Alexa and Google Assistant also provide similar functions and have gained widespread adoption in homes around the world.
But virtual assistants are not just limited to personal use; they also have real-world applications in various industries. For example, many businesses now use chatbots on their websites or social media platforms to provide customers with quick responses and support. These chatbots use natural language processing (NLP) algorithms to understand customer queries and provide relevant answers or solutions.
In addition to customer service, virtual assistants and chatbots are also being used in healthcare settings. They can assist doctors by gathering patient data and helping with diagnosis based on medical records. This not only saves time but also reduces the risk of human error.
Another industry that has seen significant benefits from integrating virtual assistants is banking and finance. Customers can now easily check their account balance, make transactions or even get investment advice through chatbots without having to visit a physical bank branch.
Moreover, virtual assistants have also found their way into education systems as digital tutors for students. These AI-powered tutors can
– Fraud Detection and Prevention
Fraud detection and prevention is one of the most critical applications of production systems in artificial intelligence. As technology advances, so do fraudulent activities, making it increasingly challenging for businesses to safeguard their operations and protect their customers. In recent years, there has been a significant rise in fraud schemes across various industries, including finance, healthcare, e-commerce, and more.
Production systems powered by AI algorithms can play a vital role in detecting and preventing fraud in real-time. These systems are designed to analyze vast amounts of data quickly and accurately identify patterns or anomalies that may indicate fraudulent behavior. Let’s take a closer look at how production systems are being used for fraud detection and prevention:
1. Real-Time Fraud Detection: Production systems use advanced machine learning techniques such as anomaly detection, clustering, and predictive modeling to identify unusual patterns or behaviors that deviate from the norm. By monitoring transactions in real-time, these systems can detect suspicious activities as they occur and trigger alerts for further investigation.
2. Uncovering Hidden Patterns: Fraudsters are constantly evolving their tactics to avoid detection by traditional rule-based systems. However, production systems have the ability to learn from past data and uncover hidden patterns that humans may not be able to identify on their own. This allows them to stay one step ahead of fraudsters and flag potentially fraudulent behavior before it leads to financial losses.
3. Multi-Layered Fraud Prevention: With the help of AI-powered production systems, businesses can implement multi-layered fraud prevention strategies that incorporate multiple techniques such
Advantages and Limitations of Using Production Systems in AI
Advantages:
1. Increased Efficiency: One of the main advantages of using production systems in AI is the increased efficiency it brings to various tasks and processes. With the use of rule-based systems, production systems are able to automate repetitive tasks, freeing up human resources for more complex and creative work.
2. Scalability: Production systems in AI are designed to be highly scalable, which means they can handle large amounts of data and tasks without compromising on performance. As businesses continue to generate massive amounts of data, production systems provide a powerful solution for managing and analyzing that data efficiently.
3. Flexibility: Production systems offer a high degree of flexibility as they can be easily modified or expanded to accommodate new rules or changing requirements. This makes them ideal for use in dynamic environments where the conditions may change frequently.
4. Debugging and Maintenance: Another advantage of production systems is their ability to identify errors and bugs quickly, making it easier for developers to debug and maintain the system. This reduces downtime and improves overall system reliability.
5. Decision-making Support: Production systems can also serve as decision-making support tools by providing intelligent recommendations based on established rules and past experiences. This can help businesses make better decisions quickly, leading to improved performance and productivity.
Limitations:
1. Limited Domain Knowledge: Production systems are only as effective as the knowledge base they have been programmed with. They lack general intelligence capabilities which means they cannot handle tasks outside their specific domain.
2. Lack of Contextual Understanding: While production systems
Challenges and Future Possibilities
Challenges and Future Possibilities:
While production systems have proven to be incredibly useful in artificial intelligence, there are still several challenges that need to be addressed in order to fully utilize their potential. These challenges include scalability, explainability, and adaptability.
Scalability: One of the major challenges with using production systems in AI is their limited scalability. Production systems rely on a set of rules and conditions to make decisions, which can quickly become overwhelming when dealing with large amounts of data. This can result in slower processing times and lower efficiency. To overcome this challenge, researchers are exploring ways to optimize the rule-based system by incorporating machine learning techniques such as neural networks.
Explainability: Another important challenge is the lack of explainability in production systems. Unlike other AI algorithms such as deep learning or reinforcement learning which can provide insights into how they arrive at a decision, production systems often operate as black boxes. This makes it difficult for humans to understand and trust the decisions made by these systems. In recent years, efforts have been made to make production systems more transparent by developing methods for generating explanations for their actions.
Adaptability: As the world evolves at a rapid pace, so do our problems and needs. This poses a challenge for traditional production systems that require manual changes to their rule base whenever new knowledge or data is introduced. However, with advancements in technologies like natural language processing (NLP) and machine learning (ML), there is potential for production systems to become more adaptive through automated rule
Conclusion
Production systems are an essential component of artificial intelligence, with applications in various real-world scenarios. From manufacturing and supply chain management to healthcare and customer service, production systems have revolutionized the way we approach problem-solving and decision-making processes. As technology continues to advance, we can only expect these applications to become more prevalent and efficient. With further research and development, the potential for production systems in AI is limitless, making it an exciting field for innovation and progress.