POPULAR
immersive learning
Post Graduate Program
in Artificial Intelligence & Data Science
The IT industry is undergoing a seismic shift, and the demand for skilled data science and artificial intelligence (AI) professionals is skyrocketing. A post-graduate program in artificial intelligence artificial and data science positions you at the forefront of this transformation. Emerging India Analytics’ program equips you with the expertise to unlock a thriving career in this dynamic field, empowering you to solve complex challenges and drive innovation through the power of data and intelligent systems.
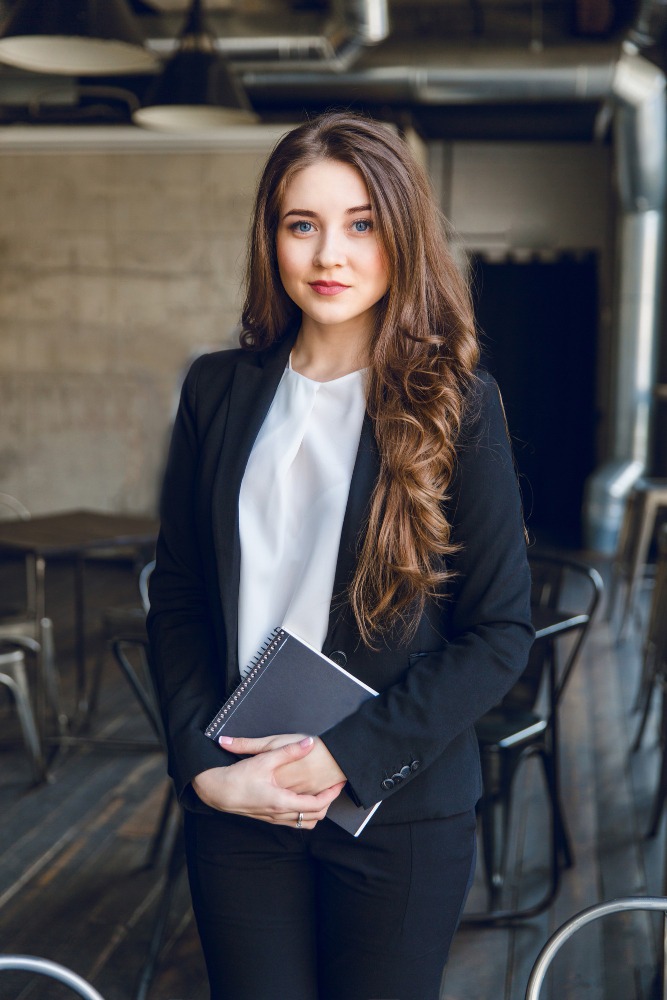
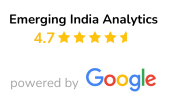
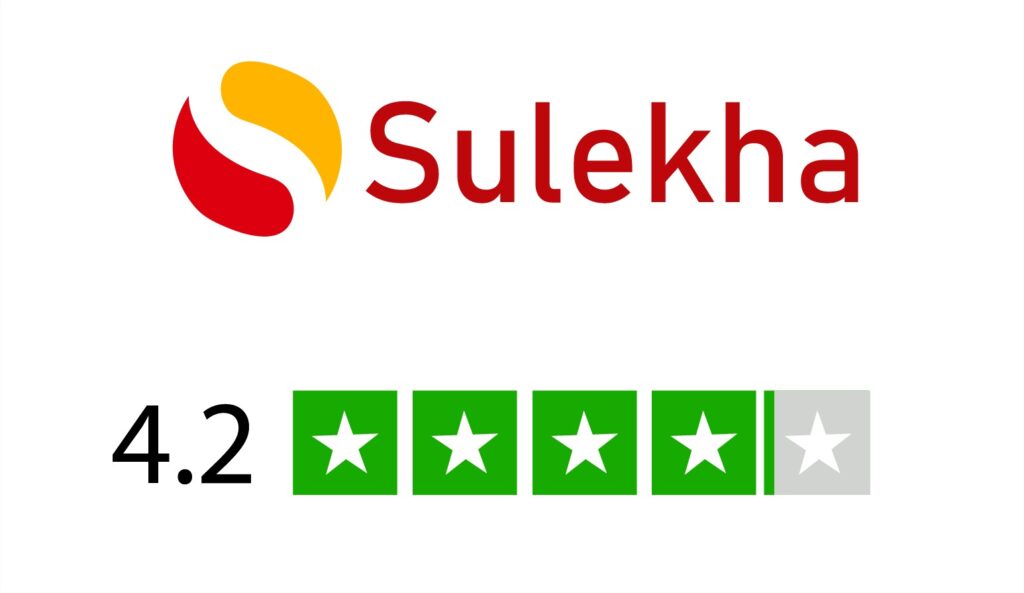
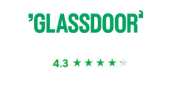
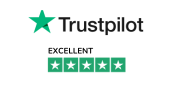
Data Science and Artificial Intelligence
Meet Your Mentors
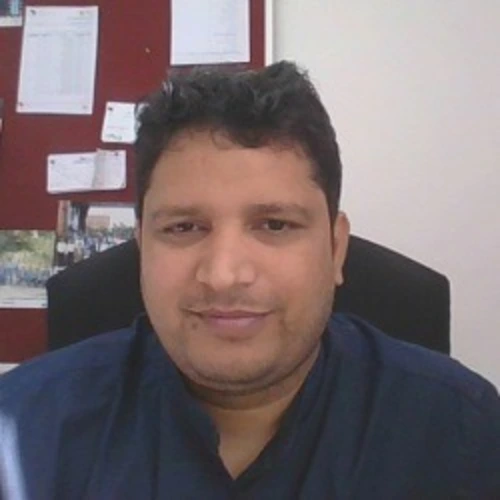
Dr Santosh Srivastava
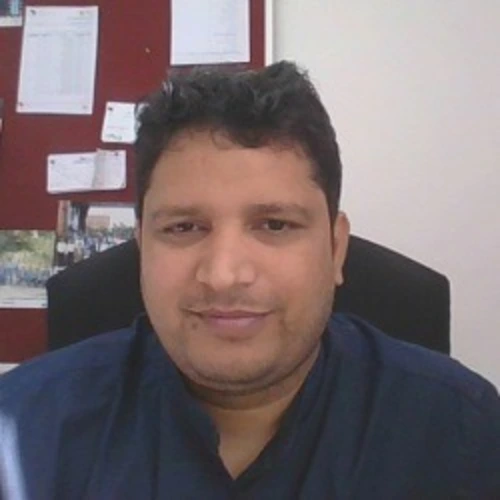
Dr Santosh Srivastava
Lead Consultant & Master Trainer- Phone: +91 88605 99698, 0120 457 5657
- Email: care@emergingindiagroup.com
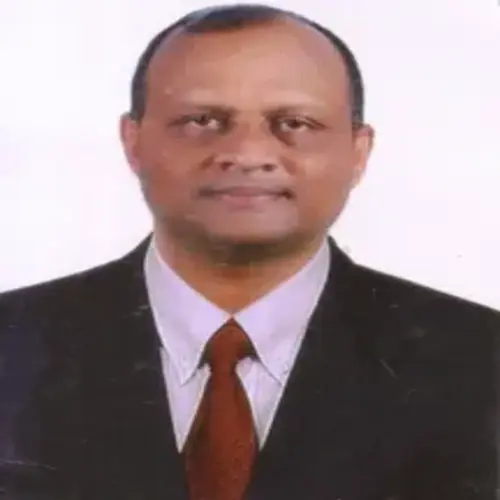
S Suresh Kumar
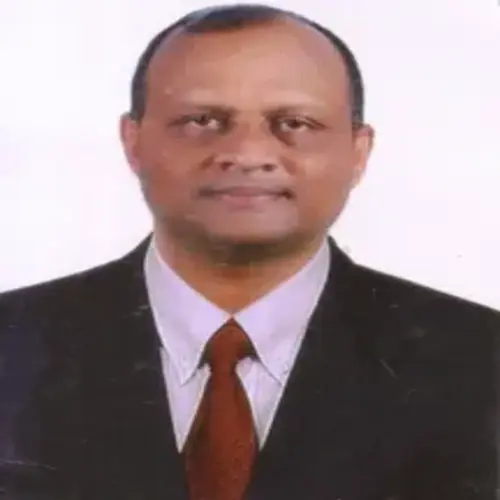
S Suresh Kumar
Data Scientist & Data Science/Analytics Trainer- Phone: +91 88605 99698, 0120 457 5657
- Email: care@emergingindiagroup.com
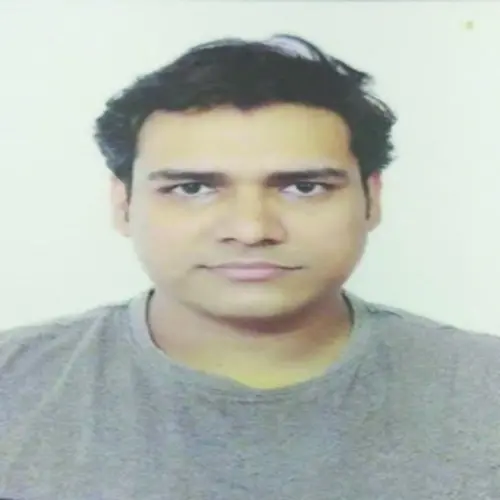
Saurabh Tripathi
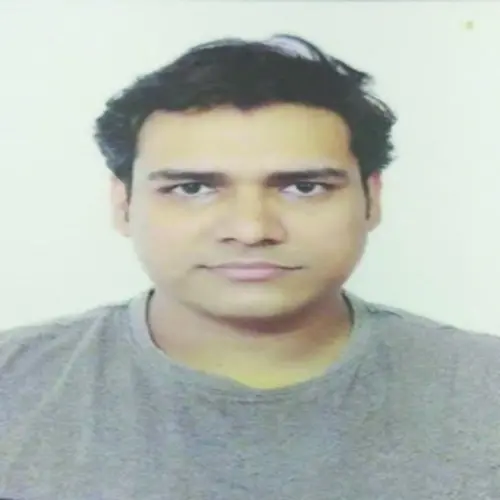
Saurabh Tripathi
Data Scientist & Data Science/Analytics Trainer- Phone: +91 88605 99698, 0120 457 5657
- Email: care@emergingindiagroup.com
lEARN WITH A WORLD CLASS CURRICULUM
Module 1. Data Science with Advanced Excel, Python, Statistics, Machine Learning, Deep Learning and R-Programming
-
📚No. of Lectures:1
-
⏳Duration of Lecture:1.5 hours
-
📝Assesment:0
-
🌟Assignment: 0
- Microsoft Excel Overview, Formatting excel and shortcuts.
- Basic Formulas, Sorting data, Filtering data, Column chart.
- Pie chart, Pivot tables, Vlookup, Match Function, Lookup Function.
- VBA, Interview Questions.
-
📚No. of Lectures: 4
-
⏳Duration of Lecture: 12 Hours
-
📝Assessment: 3
-
🌟Assignment: 2
- Why Python, PEP-8, Variables,Data Types, Operators,Strings, Indexing
- Block Structure, Data Structures.
- Functions, Lamda Function ,Modules, Class.
- Intro to Numpy, Pandas,
- Pandas, Matplotlib.
- Seaborn.
-
📚No. of Lectures: 6
-
⏳Duration of Lecture: 18 Hours
-
📝Assessment: 3
-
🌟Assignment: 2
- Descriptive stats [Sample, Population, Mean, Median, Mode, Std Deviation, Variance, Range, IQR, Outliers]
- skewness, kurtosis,Probability
- Probability Distributions- Discrete Probability Distribution [Probability Mass Function, Binomial Distribution, Poisson Distribution, Discrete Uniform Distribution
- Probability Distributions- Continuous Probability Distribution [Probability Density Function, Normal Distribution, Continuous Uniform, Exponential Distribution, Central Limit Theorem, Confidence Interval
- Hypothesis testing [Z test: One Sample , Student T distribution: One Sample T-test, Two Sample T-test]
- Hypothesis testing : [Chi Square- Chi-Square goodness of fit, Chi square test of independence]
- Annova [one Way Annova]
- Covariance, Correlation [ Pearson, Spearman, Linear]
-
📚No. of Lectures: 4
-
⏳Duration of Lecture: 12 Hours
-
📝Assessment: 4
-
🌟Assignment: 2
- Introduction to ML, Encoding, Feature Scaling[Normalization, Standardization], Linear regression
- AUC ROC Curve, Confusion Matrix, MSE, MAE,RMSE, R2 Score, Adj R2 Score, F1 Score, Accuracy
- Logistic Regression,Decision Trees
- Cross Validation [K-fold cross-validation, Hold-out cross-validation, Stratified k-fold cross-validation]
- Naive Bayes,KNN
- SVM,Random Forest
- Type 1 and Type 2 error, Lasso Regression, Ridge Regression
- Ada Boost, Adagrad
- XgBoost
- PCA,K-Means
-
📚No. of Lectures: 10
-
⏳Duration of Lecture: 30 Hours
-
📝Assessment: 4
-
🌟Assignment: 4
- Introduction to Neural Network, Foreward Propagation, Activation Function
- Activation Function[ Linear, Sigmoid, Tanh, ReLu, Leaky ReLu, ELU, Softmax, Swish]
- Optimizers[ SGD, RMSProp, Adam, Adadelta, Adamax, Adagrad,Nadam]
- Loss [Classification- BinaryCrossentropy class/ CategoricalCrossentropy class/ SparseCategoricalCrossentropy class/ Poisson class/ binary_crossentropy function/ categorical_crossentropy function/ sparse_categorical_crossentropy function]
- Loss[Regression- MeanSquaredError class, MeanAbsoluteError class, MeanAbsolutePercentageError class MeanSquaredLogarithmicError class, CosineSimilarity class]
- Weight Initialization [ Xavier Weight Initialization, Normalized Xavier Weight Initialization, He weight Initialization]
- Padding, Pooling, Convolution
- NN Implemention
- Callbacks [ ReduceLROnPlateau, ModelCheckpoint]
- Callbacks [Earlystopping, TensorBoard], Keras Tuner
- Neural Network Implementation
-
📚No. of Lectures: 11
-
⏳Duration of Lecture: 33 Hours
-
📝Assesment: 3
-
🌟Assignment: 2
- Introduction to TSA, Component of TSA
- Methods to check stationarity [ADF (Augmented Dickey-Fuller), KPSS (Kwiatkowski–Phillips–Schmidt–Shin)]
- Time Series Analysis – AR
- Time Series Analysis [Moving Average, Simple Moving Average (SMA), Cumulative Moving Average (CMA) Exponential Moving Average (EMA)]
- ACF, PACF, ARMA, ARIMA
- LSTM, GRU
-
📚No. of Lectures: 6
-
⏳Duration of Lecture: 33 Hours
-
📝Assesment: 3
-
🌟Assignment: 2
- What is R Programming, Variables and Data Type in R.
- Logical Operators,Vectors,List,Matrix,Data Frame,Flow Control, Functions in R.
- Data Manipulation in R- dplyr, Data Manipulation in R- tidyr.
- Data Visualization In R,
- 📚No. of Lectures: 4
- ⏳Duration of Lecture: 12 Hours
- 📝Assessment: 2
- 🌟Assignment: 0
-
📚No. of Lectures: 1
-
⏳Duration of Lecture: 1.5 Hours
-
🌟Projects: 5
Module 2. Business Intelligence Using SQL,Tableau and Power Bl
-
📚No. of Lectures: 1
-
⏳Duration of Lecture: 1.5 Hours
-
📝Assessment: 0
-
🌟Assignment: 0
- BASIC OF DATABASE,TYPES OF DATA BASE,DATA TYPES,SQLOPERATORS,EXPRESSION (BOOLEAN,DATE,NUMERIC), CREATE,INSERT
- DROP,TRUNCATE,DELETE,ALTER, UPDATE,SELECT, RANGE OPERATER,IN,WILDCARD, LIKE CLAUSE
- CONSTRAINT,AGGREGATION FUNCTION,GROUP BY,ORDER BY , HAVING,
- JOINS,CASE,COMPLEX QUERIES,DOUBT CLEARING
- Trigger, Stored Procedures, Common Table Expression, Index, Except, Exists, Grouping set
- Pivot, Rollup, Cube, Constraints, Partition
-
📚No. of Lectures: 6
-
⏳Duration of Lecture: 18 Hours
-
📝Assessment: 3
-
🌟Assignment: 2
- Tableau Desktop, Tableau products
- Data import, Measures, Filters
- Data transformation, Marks, Dual Axis
- Pareto Analysis, Table calculation, Multiple data source blending
- Advanced charting techniques, Tableau prep tool
- Manage worksheets, Data visualization, Dashboarding,Project
-
📚No. of Lectures: 6
-
⏳Duration of Lecture: 18 Hours
-
📝Assessment: 3
-
🌟Assignment: 2
- POWER BI PLATFORM, PROCESS FLOW
- FEATURES, DATASET,BINS
- PIVOTING, QUERY GROUP,DAX FUNCTION
- Multiple data source blending
- Basic of SSIS for ETL
- FORMULA, CHARTS, REPORTS, DASHBOARDS
-
📚No. of Lectures: 6
-
⏳Duration of Lecture: 18 Hours
-
📝Assessment: 3
-
🌟Assignment: 1
-
📚No. of Lectures: 1
-
⏳Duration of Lecture: 1.5 Hours
-
🌟Projects: 3 – 5
Module 3. BIG DATA
-
📚No. of Lectures: 1
-
⏳Duration of Lecture: 1.5 Hours
-
📝Assessment: 0
-
🌟Assignment: 0
- Introduction, Installation, Data Modelling , Database operation- create and drop, Collection- create and drop Data types, Insert, Query
- Update, Delete, Find, Limit , Skip, Create Index, ObjectID
- Aggregate, Replication, Shrading, Dumping and Restore, Mongostat and Mongotop,
- MongoDB client setup using Java, Reference and Embedded Relationship, DbRefs, Cover Query, $explain, $hint
- findAndModify, Indexing Array and subfield, ObjectId, Mapreduce, Text Search, Index, RegeX
-
📚No. of Lectures: 5
-
⏳Duration of Lecture: 15 Hours
-
📝Assessment: 2
-
🌟Assignment: 1
- Installation, Syntax [main()/printIn()/print()], Variable [String, Int, Boolean, float, char]
- Datatypes, Type casting, Operators, conditions, loop [ for, while], switch, break, continue
- array, methods [ parameters, overloading, recursion]
- Class [attributes, constructors, modifiers, oops, encapsulation, polymorphism, enums, abstraction, interface, exceptions, iterator, hashmap, hashset,threads], file handling
-
📚No. of Lectures: 5
-
⏳Duration of Lecture: 15 Hours
-
📝Assessment: 2
-
🌟Assignment: 1
- Types of Data,Introduction to Bigdata(History,V’s of Bigdata, Advantages & Disadvantages of BigData ), Use of Bigdata in different sectors, Introduction to Hadoop, Scaling (Horizontal and Vertical), Challenges in Scaling, Concept and challenges in parallel computing, Distributed Computing and use in Hadoop,Intro to Tools in Hadoop, Life cycle of Bigdata Analytics
- On Premises Installation Oracle Virtual Box and setup of VM & Ubuntu, Basic Linux command,Download and Installation of Hadoop,Introduction to Hadoop, Core components of Hadoop, Hadoop working Principle,HDFS Architecture
- VM creation on Cloud (AZURE), Configuration & Insight to Single Node Hadoop Deployment(bsshrc, hadoop-env, core-site, hdfs-site,mapred-site,yarn-site) , Format HDFS Namenode.
- Hadoop Commands and implementation
- Mapreduce, Mapreduce Implementation
- Mapreduce Implementation, Introduction to Hive, Hive Installation,Hive Implementaion,
- Hive Query Language, SQL Opeartions
- HIVE_SQL Opeartions
- Introduction to Sqoop, Hbase, Installation of Sqoop, Installation of Hbase, Installation of Spark, Hbase Query
- Hbase Query, PySpark Query
- PIG Installation and Query
- Oozie
- Flume [Introduction , Architecture, Environment, Implementation]
- Scala [ introduction, Architecture, Environment, Variable, Functionsclass objects]
- Scala [ Operators, Traits, Implementation]
-
📚No. of Lectures: 16
-
⏳Duration of Lecture: 48 Hours
-
📝Assessment: 6
-
🌟Assignment: 3
-
📚No. of Lectures: 1
-
⏳Duration of Lecture: 1.5 Hours
-
🌟Projects: 3-5
MODULE 4: Generative AI
- Introduction to AI, Hype vs. Reality, Business Applications, Ethical Considerations, Introduction to Generative AI, From Text Generation to Multimodal Models, Potential and Challenges
-
📚No. of Lectures: 1
-
⏳Duration of Lecture: 3 Hours
-
📝Aassessment: 0
-
🌟Assignment: 0
- Introduction to open source Huggingface transformers platform, Review of NLP Basics & Text Pre-processing, Introduction to NLP Concepts: Language Representations, Tokenization, Part-of-Speech Tagging, Text Preprocessing
- Feature Engineering: Normalization, Stemming, Lemmatization, Stop Word Removal, Understanding key NLP Applications using Huggingface platform
- Sentiment analysis, Sentence classification, Generating text, Extracting an answer from text
-
📚No. of Lectures: 3
-
⏳Duration of Lecture: 9 Hours
-
📝Aassessment: 2
-
🌟Assignment: 1
- Sorting, Conditional Execution, Assignment Statement, Modifying Variable attribute,
- Functions, Coercion, Loops, Data Validation, DataWrangling
-
📚No. of Lectures
-
⏳Duration of Lecture
-
📝Worksheets
-
🌟Test/Projects
-
Introduction to Large Language Models (LLMs)
– Structure of popular models
– Types of Models: text to text, text to image, text to video, multimodal - Other types of Generative AI algorithms
– GANs ( Generative Adverserial Networks),
– Variational Autoencoders (VAEs), Diffusion Models, Mixture of Experts,
– Diffferent models available currently for image ( DALLE-2, Midjourney) - Hands on practice of NLP tasks using Huggingface library and opensource language models such as Bloom for finetuning a LLM, zero and few shot classification,
-Applications of Generative AI in business - – Customer Insights & Sentiment Analysis
– Personalized Marketing & Content Creation
– Chatbots: Automating Customer Service and Support
– Document Processing Automation
-
📚No. of Lectures: 4
-
⏳Duration of Lecture: 12 Hours
-
📝Aassessment: 2
-
🌟Assignment: 1
- AI Application Stack: Infrastructure & foundation layer
– Overview of AI infrastructure: cloud platforms, GPU, and distributed computing,
– Setting up an AI environment for generative models
– Infrastructure considerations for scalable AI applications
– Retrieval augmentation generation or RAG
-
📚No. of Lectures: 1
-
⏳Duration of Lecture: 3 Hours
-
📝Assesment: 1
-
🌟Assignment: 0
- Langchain, Applied use case for Gen AI
– hands on exercise
– Designing a custom chatbot
– Data analytics using Gen AI model such as OpenAI API
-
📚No. of Lectures: 1
-
⏳Duration of Lecture: 3 Hours
-
📝Assesment: 0
-
🌟Assignment: 1
- Hallucination, Data Privacy, Ethics, and Environmental Impact of AI & future of Work
– Importance of data privacy in AI applications
– Ethical considerations in AI development and Deployment
– Environmental Impact and Sustainability in AI
– The Future of Work: How AI Will Reshape Roles and Responsibilities
-
📚No. of Lectures: 1
-
⏳Duration of Lecture: 3 Hours
-
📝Assesment: 1
-
🌟Assignment: 1
-
📚No. of Lectures: 1
-
⏳Duration of Lecture: 1.5 Hours
-
🌟Projects: 3-5
MODULE 5: ARTIFICIAL INTELLIGENCE
-
📚No. of Lectures: 1
-
⏳Duration of Lecture: 3 Hours
-
📝Assessment: 0
-
🌟Assignment: 0
- Introduction to text mining, text processing using python
- Introduction to NLTK, Tokennization, Stemming, Bag of words
- Sentiment Analysis, Name- Entity Recognition
- Text Segmentation, Text Mining, Text Classification
- LDA
- Automatic speech recognition (Librosa/gardio/transformers)
- Automatic speech recognition (Librosa/gardio/transformers)
- 📚No. of Lectures: 7
- ⏳Duration of Lecture: 21 Hours
- 📝Assessment: 4
- 🌟Test/Projects
- Introduction to Image Processing, Feature Detection, OpenCV
- Convolution, Padding, Pooling & its Mechanisms
- Forward Propagation & Backward Propagation for CNN
- CNN Architectures like AlexNet, VGGNet, InseptionNet, ResNet, Transfer Learning
-
📚No. of Lectures: 4
-
⏳Duration of Lecture: 12 Hours
-
📝Aassessment: 2
-
🌟Assignment: 1
- Introduction, YOLO Architecture, Working Methods
- Application, Pre trained object detection
- Custom trained object detection, Darknet preparation, Data collection, Image labelling, Sync Compilation and testing
-
📚No. of Lectures: 3
-
⏳Duration of Lecture: 9 Hours
-
📝Aassessment: 1
-
🌟Assignment: 1
- RL Framework, Component of RL Framework, Exampes of Systems
- Types of RL Systems, Q-Learning
-
📚No. of Lectures: 4
-
⏳Duration of Lecture: 12 Hours
-
📝Aassessment: 1
-
🌟Assignment: 1
-
📚No. of Lectures: 1
-
⏳Duration of Lecture: 1.5 Hours
-
🌟Projects: 3-5
MODULE 6: DSA
- Orientation
-
📚No. of Lectures: 1
-
⏳Duration of Lecture: 1.5 Hours
-
📝Assessment: 0
-
🌟Assignment: 0
- Introduction to Data Structure [What are Data Structures?, Types of Data Structures, Types of Algorithms]
- Recursion[ What is Recursion?, How Recursion works?, Recursive vs Iterative Solutions, When to use/avoid Recursion?, How to find Fibonacci numbers using Recursion?]
- Big O [Analogy and Time Complexity, Big O, Big Theta and Big Omega, Space Complexity, Drop the Constants and the non-dominant terms, Add vs Multiply, measure the codes using Big O?,time complexity for Recursive calls?]
- Arrays[Types of Arrays, Arrays in Memory, Operation on one dimensional and two-dimensional array,Time and Space complexity of one-dimensional Array and two-dimensional Array]
- Dictionaries ,Tuples, LIst [ What is a List, List Operations, Common List pitfalls and ways to avoid them, Time and Space Complexity of List]
-
📚No. of Lectures: 1
-
⏳Duration of Lecture: 3 Hours
-
📝Assessment: 2
-
🌟Assignment: 1
- Circular Singly Linked List [Insertion in Circular Singly Linked List, Insertion Algorithm in Circular Singly Linked List, Insertion method in Circular Singly Linked List, Traversal of Circular Singly Linked List,Searching a node in Circular Singly Linked List, Deletion of a node from Circular Singly Linked List, Deletion Algorithm in Circular Singly Linked List, Method in Circular Singly Linked List, Deletion of entire Circular Singly Linked List, Time and Space Complexity of Circular Singly Linked List]
- Doubly Linked List [ Creation of Doubly Linked List, Insertion in Doubly Linked List, Insertion Algorithm in Doubly Linked List, Insertion Method in Doubly Linked List, Traversal of Doubly Linked List
- Reverse Traversal of Doubly Linked List, Searching for a node in Doubly Linked List, Deletion of a node in Doubly Linked List, Deletion Algorithm in Doubly Linked List, Deletion Method in Doubly Linked List, Deletion of entire Doubly Linked List, Time and Space Complexity of
-
📚No. of Lectures: 1
-
⏳Duration of Lecture: 3 Hours
-
📝Assessment: 2
-
🌟Assignment: 3
- Doubly Linked List
- Linked List [ Insertion in Singly Linked List in Memory, Insertion in Singly Linked List Algorithm, Insertion Method in Singly Linked List, Traversal of Singly Linked List, Search for a value in Single Linked List, Deletion of node from Singly Linked List, Deletion Method in Singly Linked List, Deletion of entire Singly Linked List, Time and Space Complexity of Singly Linked List]
- Queue [ Queue using Python List – no size limit and operations (enqueue, dequeue, peek), Circular Queue and Operations (enqueue, dequeue, peek), Linked List and Operations (Create, Enqueue(), Dequeue(), isEmpty, Peek),, Time and Space complexity of Queue using Linked List]
-
📚No. of Lectures: 1
-
⏳Duration of Lecture: 3 Hours
-
📝Assessment: 2
-
🌟Assignment: 2
- Binary Tree [Binary Tree, Types, Basic Operations, Searching for a node in Binary Tree, Insertion, Deletion, Traversals with LinkedList and List, PreOrder , InOrder , PostOrder LevelOrder]
- Binary Heap[ Binary Heap Property, Heap Operations Creation, Peek, Insertion, Extraction, Deletion ,Time and space complexity of Binary Heap]
- Sort Algorithms [What is Sorting?,Types of Sorting, Sorting Terminologies, Bubble Sort, Selection Sort, Insertion Sort, Bucket Sort, Merge Sort, Quick Sort, Heap Sort]
- Circular Doubly Linked List [ Creation of Circular Doubly Linked List, Insertion in Circular Doubly Linked List, Insertion Algorithm in Circular Doubly Linked List, Insertion Method in Circular Doubly Linked List
- Traversal of Circular Doubly Linked List, Reverse Traversal of Circular Doubly Linked List, Search for a node in Circular Doubly Linked List, Delete a node from Circular Doubly Linked List, Deletion Algorithm in Circular Doubly Linked List, Deletion Method in Circular Doubly Linked List, Entire Circular Doubly Linked List, Time and Space Complexity of Circular Doubly Linked List, Time Complexity of Linked List vs Arrays ]
-
📚No. of Lectures: 1
-
⏳Duration of Lecture: 3 Hours
-
📝Assessment: 2
-
🌟Assignment: 2
- Stack [Stack Operations, Create Stack using List without size limit, Operations on Stack using List (push, pop, peek, isEmpty, ), Create Stack with limit (pop, push, peek, isFull, isEmpty, ), Create Stack using Linked List
- Operation on Stack using Linked List (pop, push, peek, isEmpty, ), Time and Space Complexity of Stack using Linked List]
-
📚No. of Lectures: 1
-
⏳Duration of Lecture: 3 Hours
-
📝Assessment: 2
-
🌟Assignment 2
- Binary Search Tree [Binary Search Tree, Insert a node to BST, Traversal, Search , Delete , Delete entire BST]
- AVL Tree [Operations on AVL Trees- Insertion,Left Left Condition, Left Right Condition, Right Right Condition, Right Left Condition, Deletion , Left Left Condition, Left Right Condition, Right Right Condition, Right Left Condition, Time and Space complexity of AVL Tree]
- Trie [Introduction to Trie, Common Operations on Trie , Insert a string in Trie, Search for a string in Trie, Delete a string from Trie
-
📚No. of Lectures: 1
-
⏳Duration of Lecture: 3 Hours
-
📝Assessment: 2
-
🌟Assignment: 2
- Hashing [What is Hashing? Why do we need it?, Hashing Terminology, Hash Functions, Collision Resolution Techniques, Hash Table ]
- Searching Algorithms [Introduction to Searching Algorithms, Linear Search, Binary Search, Time Complexity of Binary Search]
- Greedy Algorithms[ Introduction, Greedy Algorithm, Activity Selection Problem, Coin Change Problem, Fractional Knapsack Problem]
-
📚No. of Lectures: 1
-
⏳Duration of Lecture: 3 Hours
-
📝Assessment: 2
-
🌟Assignment: 1
- Divide and Conquer Algorithms[ Divide and Conquer AlgorithM, Common Divide and Conquer algorithms, How to solve Fibonacci series using Divide and Conquer approach?, Number Factor House Robber, Convert One String to another, Zero One Knapsack problem , Longest Common Sequence Problem, Longest Palindromic Subsequence , Minimum cost to reach the Last cell , Minimum Cost to reach the Last Cell in 2D array , Number of Ways to reach the Last Cell with given Cost
-
📚No. of Lectures: 1
-
⏳Duration of Lecture: 3 Hours
-
📝Assessment: 2
-
🌟Assignment: 1
- Graph Algorithms[ Graph Representation, Create a graph using Python, Graph traversal – BFS, BFS Traversal in Python, Graph Traversal – DFS,DFS Traversal in Python, BFS Traversal vs DFS Traversal, Topological Sort, Topological Sort Algorithm, Topological Sort in Python, Single Source Shortest Path Problem (SSSPP), BFS for Single Source Shortest Path Problem (SSSPP), BFS for Single Source Shortest Path Problem (SSSPP) in Python, Why does BFS not work with weighted Graphs?, Why does DFS not work for SSSP?, Dijkstra’s Algorithm for SSSP, Dijkstra’s Algorithm in Python, Dijkstra Algorithm with negative cycle, Bellman Ford Algorithm, Bellman Ford Algorithm with negative cycle, Why does Bellman Ford run V-1 times?, Bellman Ford in Python, BFS vs Dijkstra vs Bellman Ford, All pairs shortest path problem
-
📚No. of Lectures: 1
-
⏳Duration of Lecture: 3 Hours
-
📝Assessment: 1
-
🌟Assignment: 1
- Dry run for All pair shortest path, Floyd Warshall Algorithm, Why Floyd Warshall?, Floyd Warshall with negative cycle,, Floyd Warshall in Python,, BFS vs Dijkstra vs Bellman Ford vs Floyd Warshall,Minimum Spanning Tree, Disjoint Set, Disjoint Set in Python, Kruskal Algorithm, Kruskal Algorithm in Python, Prim’s Algorithm, Prim’s Algorithm in Python,Prim’s vs Kruskal
-
📚No. of Lectures: 1
-
⏳Duration of Lecture: 3 Hours
-
📝Assessment: 1
-
🌟Assignment: 1
-
📚No. of Lectures: 1
-
⏳Duration of Lecture: 1.5 Hours
-
🌟Projects: 5-7
Course Certificates
Career Services By emergingindiagroup
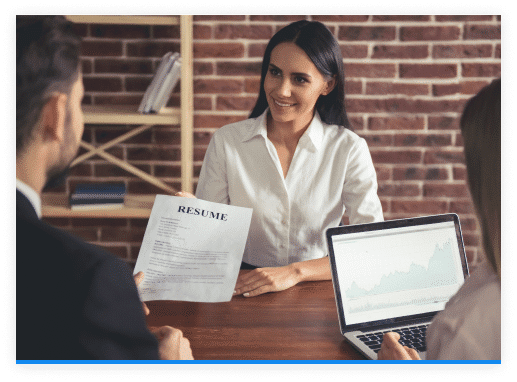
Placement Assistance
Exclusive access
Mock Interview Preparation
1 on 1 Career Mentoring Sessions
Career Oriented Sessions
Resume & LinkedIn Profile Building
Real World Projects
- Practice 20+ Essential Tools
- Designed by Industry Experts
- Get Real-world Experience
Real State Analytics
Solar Power Efficiency
Recommendation Engine
Stock Price Prediction
Weather Forecasting
Image Classification
Gesture Recognition
American Sign Language Recognition
Sentiment Analysis
Our Alumni Works At
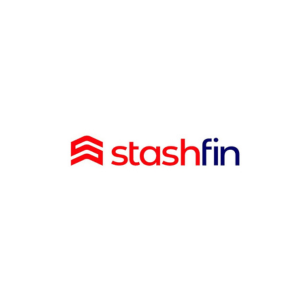
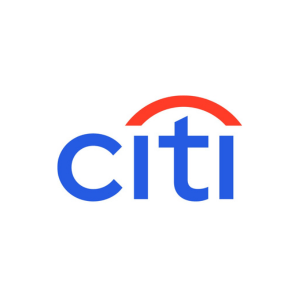
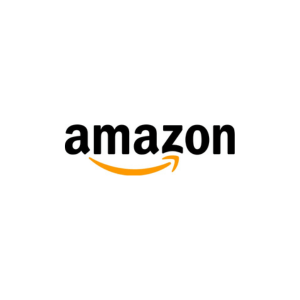
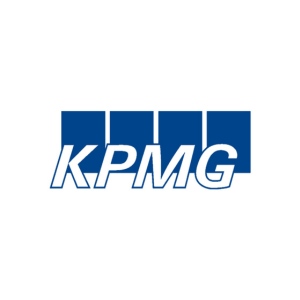
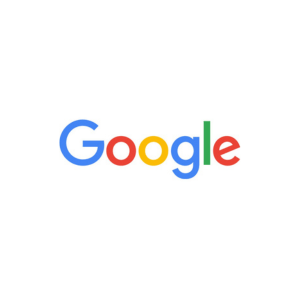
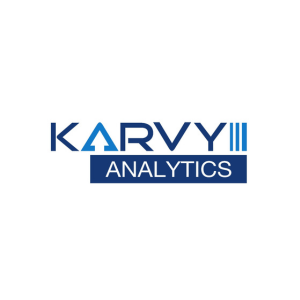
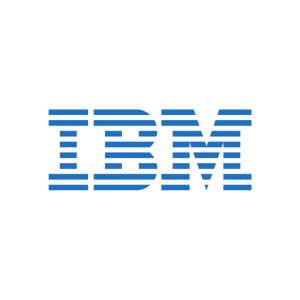
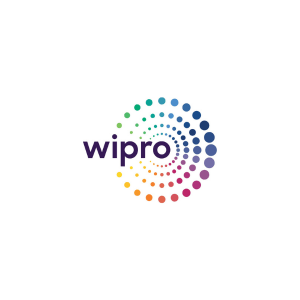
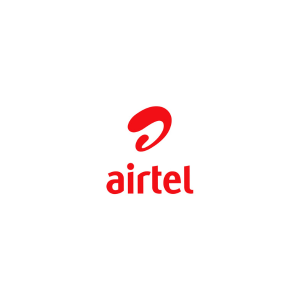
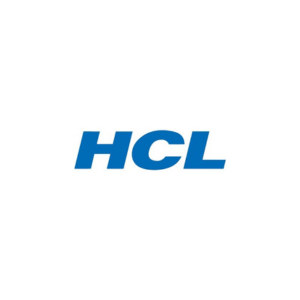
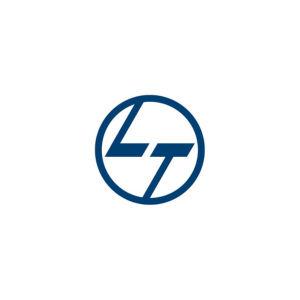
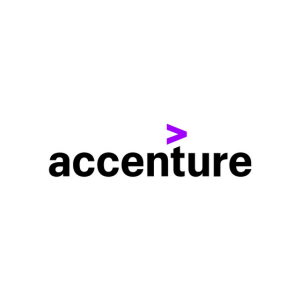
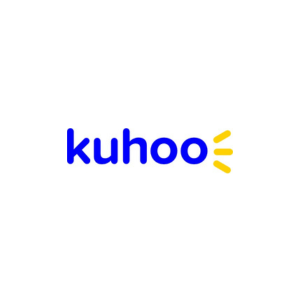
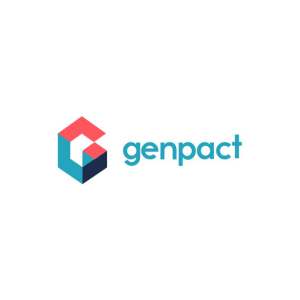
Learners thought about us
Admission Details

Submit Application
Tell us a bit about yourself and why you want to join this program

Application Review
An admission panel will shortlist candidates based on their application

Admission
Selected candidates will be notified within 1week.
Program Fees
Our Loan Partners
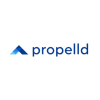
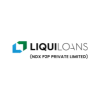
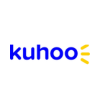
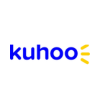
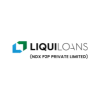
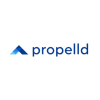
Zero Cost EMI options Available
from RBI Approved NBFCs
Starting from ₹2,999*
Others Payment Options
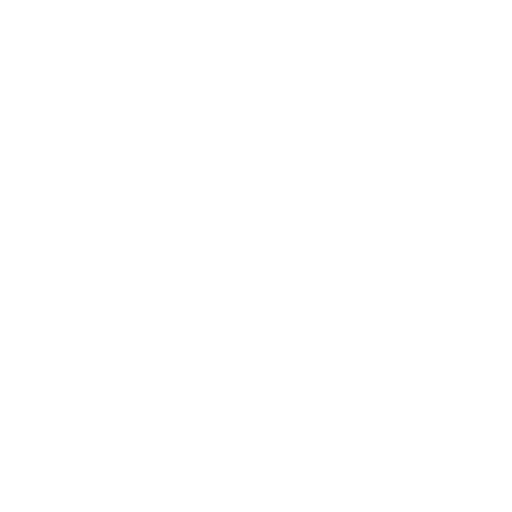
Internet Banking
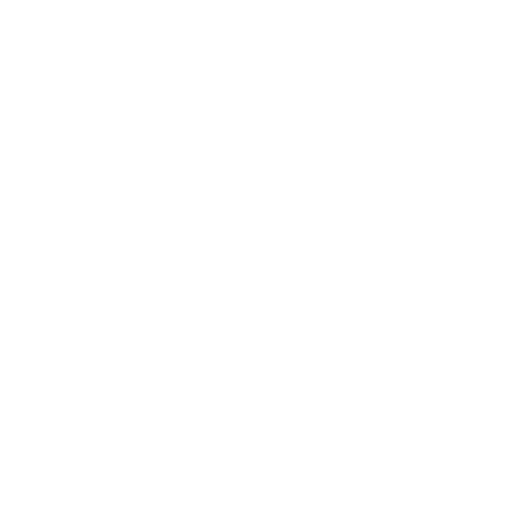
Credit / Debit Card
Total Admission Fees
₹1,99,999*(Excluding GST)
Data Science and Artificial Intelligence Course Delivery Plan
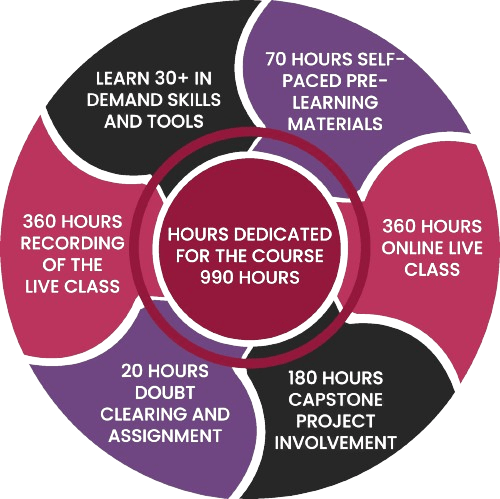
Why Choose Us?
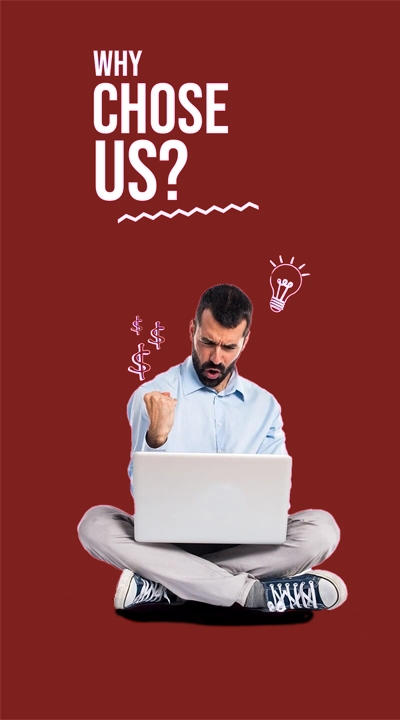
By choosing Emerging India Analytics, you’ll gain the skills and knowledge to:
Program Objectives
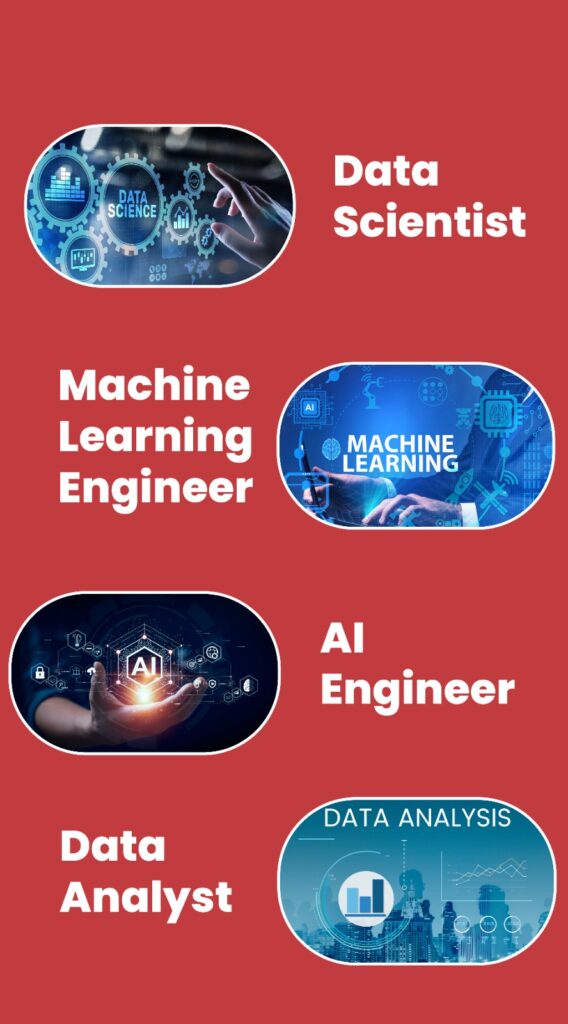
Who Should Apply?
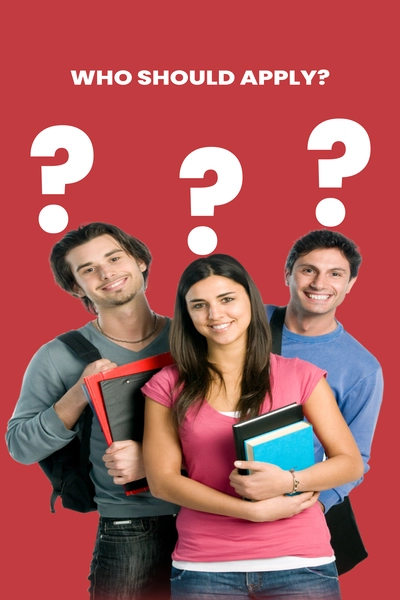
Are you passionate about using data to solve complex problems and drive innovation? Do you have a strong analytical mind and a desire to learn cutting-edge technologies? If so, then our Data Science Masters Programs with Artificial Intelligence might be the perfect fit for you!
This program is ideal for:
If you’re ready to unlock the power of data and become a leader in the field of artificial intelligence, we encourage you to apply!
Why the NASSCOM-led Data Science and Artificial Intelligence Course Stands Out?
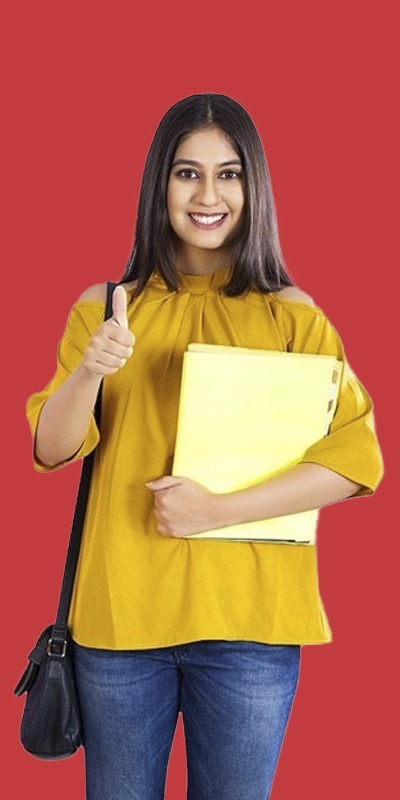
Salary Package of Data Scientist and Artificial Intelligence Professionals
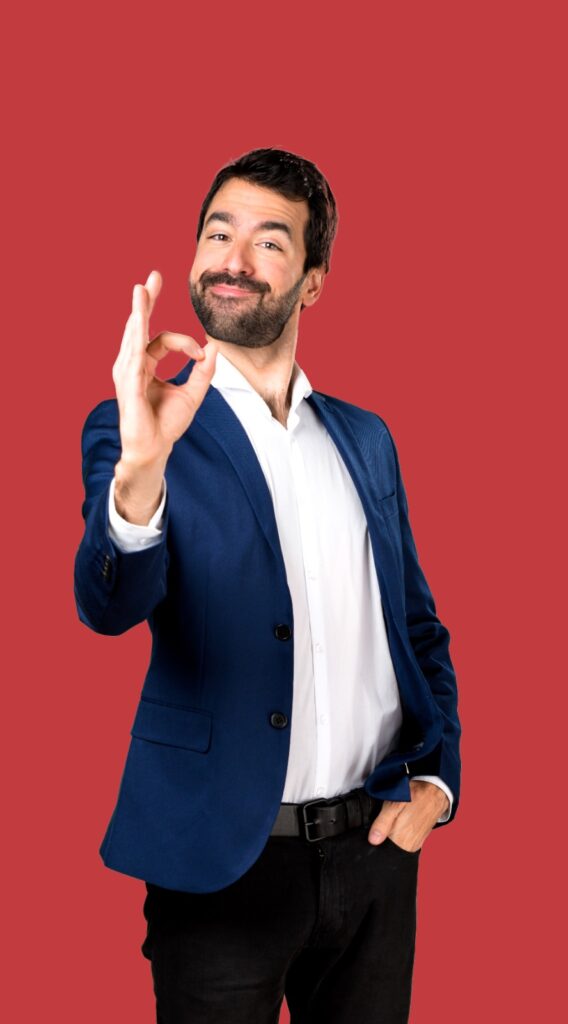
The booming demand for data science expertise translates to impressive salary packages. Studies show the average annual salary for data scientists in India falls between ₹7 lakhs and ₹14 lakhs. However, this figure soars with experience and specialization. Top data scientists with advanced skills and proven success can command salaries exceeding ₹20 lakhs annually.
This earning potential makes a well-designed data science masters program a strategic investment. Programs like those offered by Emerging India Analytics equip you with the knowledge and skills to not only kickstart your data science career but also thrive in this lucrative field.
Our NASSCOM-aligned curriculum ensures you master the latest tools and techniques used by top companies. Our expert instructors, passionate about data science and your success, guide you through practical applications. You’ll graduate with the confidence and skills to secure high-paying data science positions.
Don’t miss out! Invest in your future with a top-tier data science masters program. Embrace the exciting world of data science and its rewarding career path.
What are the Career Growth Opportunities Post-Course Completion?
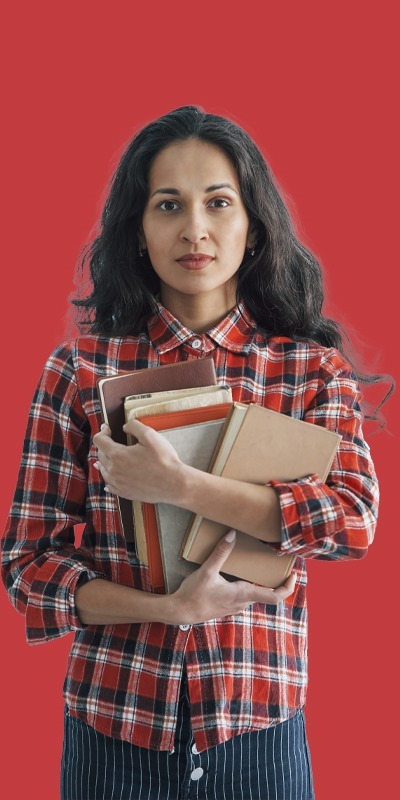
Leading Companies Recruit Data Science Specialists
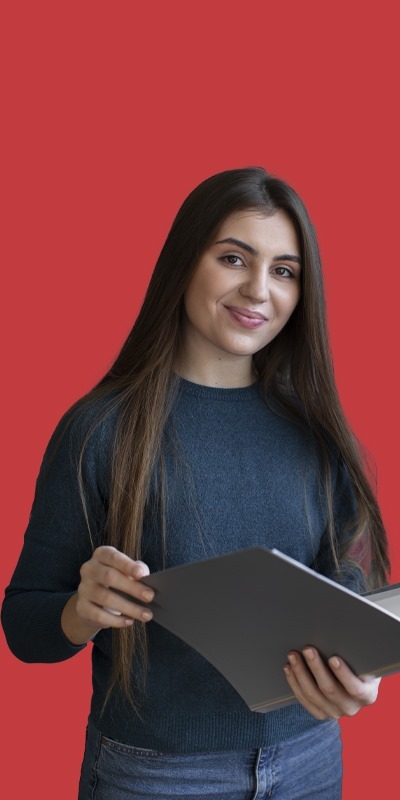
Roles and Responsibilities in the Data Science Field
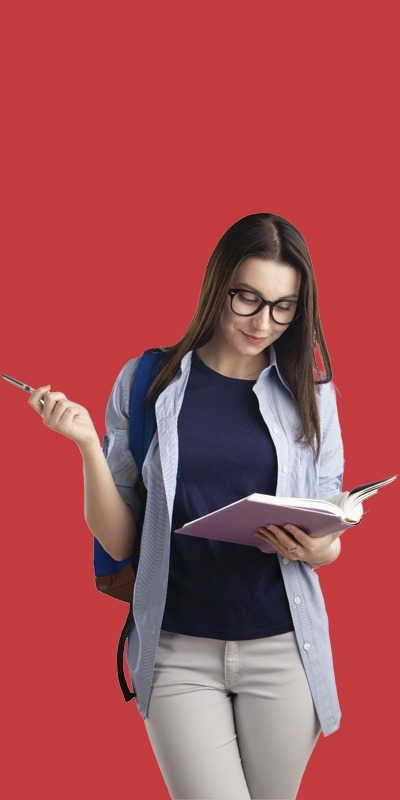
Top FAQ
While a strong foundation in math, statistics, and computer science is beneficial, our program is designed to be accessible to individuals with a bachelor’s degree in any quantitative field. We recommend reviewing our program brochure for a detailed list of prerequisites.
This program is suitable for both beginners and those with some experience in data science. We offer a comprehensive curriculum that starts with fundamental concepts and progresses to advanced topics in data science and artificial intelligence. No matter your experience level, you’ll gain the necessary skills and knowledge to excel in this field.
Our advanced certificate program in data science covers a wide range of data science and AI concepts, including:
- Statistics and Probability
- Machine Learning Algorithms (Supervised & Unsupervised Learning)
- Data Wrangling and Cleaning
- Data Visualization Techniques
- Deep Learning and Neural Networks
- Natural Language Processing
- Big Data Processing with tools like Hadoop and Spark
We believe in a learning-by-doing approach. Our program incorporates a significant amount of hands-on experience through real-world case studies, projects, and assignments. You’ll work with industry-standard tools and technologies, allowing you to apply your learnings to practical scenarios.
Throughout the program, you’ll undertake a variety of projects designed to challenge your skills and develop your problem-solving abilities. These projects may involve tasks like building a machine learning model to predict customer churn, analyzing social media data to understand customer sentiment, or developing a data visualization dashboard to communicate insights to stakeholders.
Graduates of our advanced certificate program in data science are well-positioned to pursue a variety of exciting career paths in the data science field. Some potential job titles include:
- Data Scientist
- Machine Learning Engineer
- Data Analyst
- Business Intelligence Analyst
- AI Engineer
- Data Visualization Specialist
Yes, we are committed to your success beyond graduation. We offer dedicated career placement assistance to help you connect with leading companies in the data science and AI space. Our team will provide guidance on resume writing, interview preparation, and job search strategies.
Yes, we understand that some students may have scheduling constraints. We offer a flexible enrollment option that allows you to complete the program at your own pace.
We encourage you to visit our program website or contact our admissions office for the latest information on tuition fees. We also offer various scholarship opportunities to help make our program more accessible.
While our advanced certificate program in data science is comprehensive and rigorous, it is not a substitute for a full master’s degree. However, it provides a strong foundation and practical skills to launch a successful data science career.
Absolutely! Our advanced certificate program in data science is designed for individuals with diverse academic backgrounds who are looking to transition into the data science field. The program equips you with the necessary skills and knowledge to bridge the gap between your existing knowledge and the demands of the data science industry.