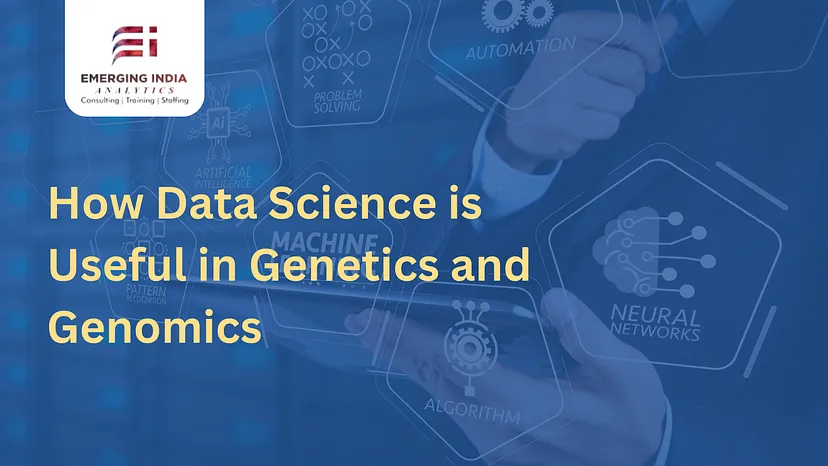
Introduction to Data Science in Genetics and Genomics
Unlocking the mysteries of our genetic makeup has always been a captivating pursuit for scientists and researchers alike. With advancements in technology and the advent of data science, this quest has taken on an exhilarating new dimension. Welcome to the thrilling world where data meets genetics and genomics!
In this blog post, we will explore how data science is revolutionizing the field of genetics and genomics, opening up unprecedented opportunities for discovery and innovation. From unraveling complex diseases to understanding our evolutionary history, data science is empowering scientists with powerful tools to analyze vast amounts of genomic information like never before.
So fasten your seatbelts as we embark on a fascinating journey into the realm where DNA sequences meet algorithms, where big data uncovers hidden insights, and where machine learning unlocks secrets buried within our genes. Let’s dive in!
The Role of Data Science in Genetic Research
Data science has revolutionized the field of genetics by providing researchers with powerful tools to analyze and interpret complex genetic data. By harnessing the power of algorithms and statistical models, data scientists can uncover patterns and insights that were previously hidden within vast amounts of genomic information.
One key role that data science plays in genetic research is in the analysis of DNA sequences. With advances in sequencing technology, massive amounts of genomic data are now being generated at an unprecedented scale. Data scientists use their expertise to develop algorithms that can efficiently process this raw sequence data, identify variations between individuals, and infer potential disease-causing mutations.
Another important aspect where data science shines is in understanding gene expression patterns. By analyzing large-scale gene expression datasets using machine learning techniques, researchers can gain valuable insights into how genes are regulated and which ones might be involved in specific diseases or conditions.
Furthermore, data science enables researchers to classify patients based on their genetic profiles. This allows for more precise diagnosis and personalized treatment plans tailored to each individual’s unique genetic makeup.
In addition, data scientists play a crucial role in integrating different types of biological datasets such as genomics, proteomics, and transcriptomics. By combining these diverse sources of information through sophisticated analytical methods like network analysis or pathway enrichment analysis, researchers can unravel complex interactions between genes and proteins implicated in various diseases.
The role of data science in genetic research cannot be overstated. It has transformed our ability to understand the intricate complexities underlying human genetics while paving the way for breakthroughs that have far-reaching implications for healthcare advancements. The integration of big genomics datasets with advanced computational methods holds immense promise for furthering our knowledge about inherited disorders and developing targeted therapies based on an individual’s unique genetic profile.
Data Analysis and Interpretation in Genomics
In the field of genomics, data analysis and interpretation play a crucial role in uncovering the hidden insights within vast amounts of genetic information. With advancements in technology, we are now able to generate enormous volumes of genomic data, from DNA sequencing to gene expression profiles.
Once this raw data is obtained, it needs to be processed and analyzed using various computational methods. Data scientists employ statistical techniques and algorithms to identify patterns, variations, and correlations within the genomes. This enables them to extract meaningful information that can help understand genetic diseases or predict an individual’s response to certain treatments.
Interpreting these findings requires expertise in genetics as well as a deep understanding of computational biology. Researchers need to carefully analyze the results obtained from their analyses and interpret them accurately. This involves comparing genomic data with existing databases, identifying potential mutations or disease-causing variants, and drawing conclusions based on evidence-backed research.
One powerful technique used for interpreting genomic data is functional annotation. This involves annotating genes based on their known functions or characteristics by leveraging existing knowledge about gene function from public databases like Gene Ontology (GO) or UniProt.
Another important aspect of data analysis in genomics is visualization. Visual representations such as heatmaps or scatter plots allow researchers to explore complex datasets more easily and identify trends visually.
Effective data analysis and interpretation are essential for making sense out of vast amounts of genomic information. By employing sophisticated computational tools combined with biological knowledge, researchers can unlock valuable insights into the genetic basis of diseases and pave the way for personalized medicine approaches tailored specifically for individuals based on their unique genetic makeup
Machine Learning Applications in Genetics
Machine learning, a subset of artificial intelligence, has revolutionized numerous fields and is now making significant strides in genetics. With its ability to analyze large amounts of data and identify patterns that humans may miss, machine learning is transforming our understanding of genetic processes.
One area where machine learning shines is in the prediction of disease risk based on genetic information. By training algorithms on vast datasets containing both genetic and clinical data, researchers can develop models that accurately predict an individual’s likelihood of developing certain diseases. This knowledge can then inform personalized healthcare decisions, enabling early detection and intervention.
Another application lies in genomics research. Machine learning algorithms can analyze genomic sequencing data to uncover associations between specific genetic variations and various traits or conditions. By identifying these links, scientists gain insights into the underlying mechanisms driving complex diseases like cancer or neurodegenerative disorders.
Additionally, machine learning techniques are utilized for gene expression analysis. These algorithms help classify genes based on their activity levels under different conditions, aiding in the identification of key regulatory pathways involved in development or disease progression.
Moreover, machine learning plays a crucial role in drug discovery by predicting drug-target interactions and optimizing lead compounds with desired properties through virtual screening methods. This accelerates the process of finding potential treatments for various ailments by narrowing down candidates from extensive libraries of compounds.
Machine learning has immense potential to transform genetics research by providing new tools for analyzing vast amounts of genomic data quickly and accurately. Its applications range from predicting disease risk to unraveling complex biological processes within our DNA. As technology advances further and datasets continue to grow exponentially larger, we can expect even more breakthroughs at the intersection of machine learning and genetics.
Case Studies: Examples of Data Science in Action in Genetics and Genomics
In the field of genetics and genomics, data science has proven to be invaluable in unlocking the secrets hidden within our DNA. Through the use of advanced computational techniques and statistical analysis, researchers have been able to gain a deeper understanding of genetic variations and their implications for human health.
One example is the study conducted by scientists at Stanford University, where they used data science methods to analyze genomic sequences from thousands of individuals. By identifying patterns in the data, they were able to pinpoint specific genes that are associated with an increased risk of developing certain diseases such as cancer or heart disease.
Another case study involved researchers at MIT who utilized machine learning algorithms to analyze large-scale genomic datasets. They developed a predictive model that could accurately identify genetic mutations linked to drug resistance in cancer cells. This information is crucial for developing personalized treatment plans for patients based on their unique genetic profiles.
Additionally, data science has played a significant role in improving our understanding of complex traits such as height or intelligence. A team of researchers from various institutions collaborated on a project known as GIANT (Genetic Investigation of Anthropometric Traits). Using sophisticated statistical models and analyzing massive amounts of genomic data from over 250,000 individuals, they identified hundreds of regions within the genome that influence human height.
These case studies demonstrate how data science techniques can revolutionize genetic research by uncovering meaningful insights from vast amounts of complex genomic data. The integration between computer science and biology allows us to explore new avenues for diagnosis, treatment, and prevention strategies tailored specifically to individual patient’s unique genetic makeup.
As technology continues to advance and more extensive datasets become available, we can expect even greater advancements facilitated by data science applications in genetics and genomics. The potential impact on healthcare is immense — personalized medicine approaches will become more precise, leading to better patient outcomes overall.
The power lies not only in collecting vast amounts of genomic data but also in analyzing and interpreting it effectively. With the aid of sophisticated algorithms
Challenges and Limitations of Using Data Science in this Field
While data science holds great promise for advancing genetics and genomics research, it also comes with its fair share of challenges and limitations. One major challenge is the sheer volume and complexity of genetic data that researchers are dealing with. Genomic datasets are often massive, consisting of billions of base pairs, which can be daunting to process and analyze effectively.
Another challenge lies in the quality and accuracy of the data itself. Genetic data can be prone to errors or inconsistencies due to various factors such as sequencing errors or sample contamination. Ensuring the reliability and integrity of the data is crucial for obtaining meaningful insights.
Furthermore, ethical considerations play a significant role when working with genetic information. Privacy concerns arise when dealing with sensitive genomic data, as individuals’ identities might be at risk if not handled properly. Striking a balance between utilizing valuable genetic information for research purposes while respecting individuals’ privacy rights poses an ongoing challenge.
In addition to these challenges, there are also limitations in our current understanding of complex biological processes underlying genetics and genomics. Despite advancements in machine learning algorithms, predicting phenotypic outcomes solely based on genomic information remains challenging due to intricate gene-gene interactions, environmental factors, epigenetic modifications, etc.
Moreover, ensuring reproducibility and transparency in computational analysis workflows is another hurdle that needs attention. Replicating results obtained from one study by other researchers should be feasible for scientific validity; however, variations in software tools used or inconsistent methodologies may hinder reproducibility efforts.
To overcome these challenges and address their limitations effectively requires interdisciplinary collaboration among scientists from fields such as computer science, statistics, biology, and medicine — fostering cross-pollination of ideas across disciplines will accelerate progress significantly.
Overall, data science offers immense potential but must navigate through these challenges thoughtfully to fully realize its impact on genetics and genomics research
Future Prospects and Impact on Healthcare
The future of data science in genetics and genomics is brimming with promise. As technology continues to advance, so too does our ability to collect and analyze vast amounts of genetic data. With this wealth of information at our fingertips, the potential for improving healthcare outcomes becomes limitless.
One area where data science has the potential to make a significant impact is personalized medicine. By mining genomic data, scientists can identify specific genetic mutations or variations that may contribute to an individual’s susceptibility to certain diseases or influence their response to treatment. This knowledge allows doctors to tailor medical interventions based on an individual’s unique genetic makeup, leading to more effective and targeted treatments.
Another exciting prospect is the use of machine learning algorithms in diagnosing rare genetic disorders. By training algorithms on large datasets containing both clinical information and genomic sequences, researchers can develop models capable of accurately identifying disease-causing variants. This could lead to earlier diagnosis, improved prognosis, and ultimately better patient outcomes.
Furthermore, data science has the potential to revolutionize drug discovery by accelerating the identification of novel therapeutic targets. Through sophisticated computational analysis methods such as network analysis and pathway modeling, researchers can uncover hidden relationships between genes and diseases that were previously unknown. This knowledge opens up new avenues for developing targeted therapies tailored specifically towards these molecular pathways.
Additionally, advances in data science have also paved the way for breakthroughs in cancer research. By harnessing big data analytics techniques like deep learning neural networks, scientists are able to identify patterns within complex tumor genomic profiles that may predict response rates for different treatment options. This allows oncologists to make informed decisions about which therapies are most likely to be effective for each patient’s unique cancer profile.
As we continue delving into the realms of genetics and genomics with increasingly powerful computing technologies at our disposal, it is clear that there are immense opportunities for improving healthcare through data science applications. From personalized medicine strategies tailored to individual genomes to revolutionizing drug discovery and advancing cancer research, the impact
Conclusion
Data science has revolutionized the field of genetics and genomics by providing powerful tools for analyzing and interpreting vast amounts of genetic information. The role of data science in genetic research cannot be underestimated, as it enables researchers to uncover crucial insights into complex diseases and potential therapeutic targets.
Through advanced data analysis techniques, such as machine learning algorithms, researchers can identify patterns and correlations within genomic datasets that may have otherwise gone unnoticed. This not only accelerates the pace of discovery but also enhances our understanding of the intricate interplay between genes and disease.
Moreover, data science has paved the way for personalized medicine by enabling scientists to extract meaningful information from individual genomes. By integrating genomic data with clinical records and other health-related variables, healthcare providers can make more accurate diagnoses, predict patient outcomes, and develop targeted treatment strategies tailored to each individual’s unique genetic makeup.
However, utilizing data science in genetics and genomics also comes with its fair share of challenges. Ethical considerations regarding privacy issues must be carefully addressed when handling large-scale genomic datasets. Additionally, ensuring the accuracy and reliability of computational models is essential to avoid misinterpretation or faulty predictions based on incomplete or biased information.
Looking ahead, the future prospects for leveraging data science in this domain are promising. As technology continues to advance at an unprecedented rate, we can expect even more sophisticated algorithms capable of extracting valuable insights from increasingly complex genomic datasets. This will undoubtedly lead to further breakthroughs in precision medicine and contribute significantly to improving healthcare outcomes worldwide.
The marriage between data science and genetics has opened up new possibilities for unraveling the mysteries encoded within our DNA. By harnessing cutting-edge analytical tools like machine learning algorithms and applying them to massive sets of genetic information, scientists are gaining unprecedented insights into human health and disease. The impact on healthcare is profound — personalized medicine is becoming a reality while empowering patients with better diagnosis options leading ultimately towards healthier lives for all.