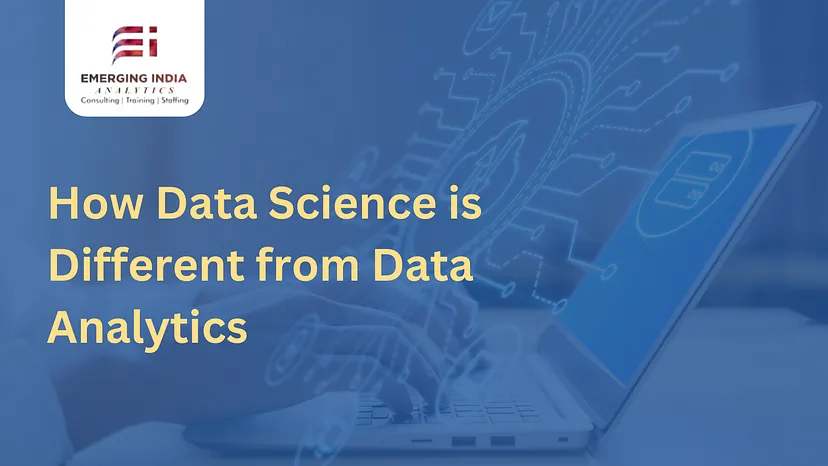
Unlocking the power of data has become a crucial aspect for businesses and industries in today’s digital era. The abundance of information available at our fingertips holds immense potential to drive informed decision-making, optimize processes, and gain valuable insights. As organizations strive to harness this vast ocean of data, two fields have emerged as game-changers: Data Science and Data Analytics.
In this blog post, we will delve into the world of data science and data analytics, exploring their key differences, applications across various sectors, job opportunities they offer, required skills for a successful career in these domains, and future growth trends. So fasten your seatbelts as we embark on an exciting journey through the fascinating realms of data!
Understanding Data Science and Data Analytics
Data Science and Data Analytics are two distinct disciplines that play a crucial role in extracting meaningful insights from data. While they share similarities, such as their reliance on data and statistical analysis, there are key differences between the two.
Data Science involves the use of various techniques, algorithms, and tools to extract knowledge or insights from structured or unstructured data. It encompasses a wide range of skills including programming, machine learning, statistics, and domain expertise. Data scientists employ complex models to uncover patterns and make predictions that help organizations optimize operations or solve complex problems.
On the other hand, Data Analytics focuses more on exploring historical datasets to identify trends, analyze performance metrics, and gain actionable insights for decision-making purposes. It involves using descriptive analytics techniques like visualization or statistical analysis to understand past trends or current scenarios.
In simpler terms: while data science is focused on discovering hidden patterns within large datasets through advanced algorithms and modeling techniques; data analytics primarily deals with analyzing existing information to provide valuable business intelligence for strategic decision-making.
Both fields rely heavily on technology but have different objectives — one aims at prediction while the other focuses on interpretation. Understanding these distinctions is essential when choosing a career path in either field. So let’s take a closer look at some of their distinguishing characteristics!
Key Differences Between Data Science and Data Analytics
Data science and data analytics are two terms that are often used interchangeably, but they actually represent distinct fields with their own unique characteristics. While both involve working with data to gain insights and drive decision-making, there are key differences between the two.
Data science is a multidisciplinary field that combines elements of statistics, mathematics, computer science, and domain knowledge. It focuses on extracting meaning from large volumes of complex data by utilizing advanced algorithms and predictive modeling techniques. Data scientists are skilled in developing innovative approaches to solve problems using machine learning algorithms.
On the other hand, data analytics primarily involves analyzing past data to understand trends, patterns, and correlations. It is focused on exploring historical datasets to uncover actionable insights that can be used for strategic planning or process optimization. Data analysts use statistical tools and visualization techniques to present findings in a clear and understandable manner.
While both roles require strong analytical skills and proficiency in programming languages like Python or R, the emphasis differs. Data scientists need more extensive knowledge of machine learning algorithms as they build models from scratch whereas data analysts focus on exploratory analysis using existing tools.
Another difference lies in the scope of work involved. Data science projects typically involve identifying business problems or opportunities where predictive modeling can provide valuable insights. In contrast, data analytics projects tend to be more descriptive in nature — examining historical patterns for reporting purposes or making recommendations based on observed trends.
Furthermore, the outputs generated also vary between these disciplines. A typical output of data science would be a predictive model capable of making accurate predictions about future events or behaviors based on historical patterns identified during the training phase. On the other hand, The output from a typical analytic project would often take the form as dashboards containing visualizations depicting key metrics derived from historical datasets
Overall, data science tends to have a broader scope encompassing various stages like problem definition, data collection, model building, fine-tuning while -data analytics is more focused on analyzing past data to uncover valuable insights.
Applications of Data Science and Data Analytics
Data science and data analytics have a wide range of applications across various industries. Let’s explore some of the key areas where these fields are making a significant impact.
In healthcare, data science is revolutionizing patient care by analyzing vast amounts of medical data to identify patterns and trends. These insights help in diagnosing diseases more accurately, predicting outcomes, and developing personalized treatment plans. Data analytics also plays a crucial role in optimizing hospital operations, improving resource allocation, and enhancing patient experience.
In finance, both data science and data analytics are used for risk assessment, fraud detection, investment strategies optimization, and algorithmic trading. By analyzing historical financial data combined with real-time market information, businesses can make informed decisions to mitigate risks and maximize returns.
E-commerce companies leverage data science techniques to gain insights into customer behavior such as purchase history or browsing patterns. This enables them to create targeted marketing campaigns that drive customer engagement leading to increased sales conversions.
The transportation industry relies on data analytics for route optimization based on traffic patterns analysis which helps reduce travel time and fuel consumption while increasing efficiency. Furthermore, data scientists work on creating predictive maintenance models ensuring timely repairs reducing downtime & operational costs
The entertainment industry uses both fields extensively to understand audience preferences through sentiment analysis of social media feeds or recommendation engines suggesting personalized content tailored to each viewer’s taste.
These are just a few examples highlighting the diverse applications of data science and data analytics in today’s world. As technology continues to advance at an exponential rate there will be countless other opportunities for these fields in sectors such as manufacturing supply chain management agriculture energy among others
Job Opportunities in the Fields of Data Science and Data Analytics
The fields of data science and data analytics offer a wide range of exciting job opportunities. With the growing importance of data in today’s digital age, professionals skilled in analyzing and interpreting large datasets are highly sought after.
Data scientists play a crucial role in extracting valuable insights from complex datasets. They utilize statistical analysis techniques, machine learning algorithms, and programming skills to uncover patterns and trends that can inform business strategies. From predicting customer behavior to optimizing supply chain operations, data scientists have the ability to drive innovation across various industries.
On the other hand, data analysts focus on collecting, cleaning, and organizing raw data for further analysis. They excel at utilizing tools like SQL or Excel to process large volumes of information efficiently. Data analysts often work closely with stakeholders to understand their specific requirements and generate reports or visualizations that help decision-making processes.
Both fields require strong analytical skills but differ in terms of depth and breadth of expertise required. While data scientists typically possess advanced degrees in computer science or mathematics, data analysts may enter the field with a bachelor’s degree coupled with relevant experience or certifications.
In terms of career paths, both roles offer diverse options such as working as consultants for specialized firms or joining established companies’ internal teams dedicated to handling their extensive datasets. Additionally, there is also ample opportunity for freelance work or starting one’s own consultancy firm catering specifically to organizations seeking expert guidance on leveraging their data assets effectively.
As technology continues to advance rapidly and more businesses recognize the value in harnessing big data capabilities, job prospects in these fields are expected to grow significantly over the coming years. Industries ranging from healthcare to finance are increasingly relying on accurate insights derived from vast amounts of information — creating a high demand for skilled professionals who can navigate this complex landscape.
To succeed in either field requires continuous learning given how fast-paced they are evolving disciplines. Staying updated with new methodologies such as deep learning algorithms and familiarizing oneself with emerging technologies like artificial intelligence (AI) and the Internet
Required Skills for a Career in Data Science or Data Analytics
To excel in the field of data science or data analytics, there are certain skills that one must possess. These skills not only help professionals stand out but also ensure they can handle the complexities and challenges of working with large datasets.
First and foremost, a strong foundation in mathematics and statistics is essential. Proficiency in areas such as linear algebra, calculus, probability, hypothesis testing, and regression analysis provides the necessary framework to understand complex algorithms and models used in data analysis.
In addition to mathematical aptitude, programming skills are crucial for both data scientists and analysts. Python and R are two popular programming languages used extensively in these fields due to their versatility and extensive libraries for statistical computing.
Data visualization is another critical skill that professionals should develop. The ability to present complex findings visually through charts, graphs, or dashboards helps stakeholders understand insights more effectively.
Domain knowledge is also vital when it comes to analyzing datasets within specific industries like healthcare or finance. Understanding the nuances of a particular domain enables professionals to ask relevant questions while interpreting data accurately.
Problem-solving abilities coupled with critical thinking play a significant role. Data scientists and analysts need to approach problems analytically by breaking them down into smaller components before arriving at solutions.
Developing expertise in mathematics/statistics, programming languages (such as Python/R), data visualization techniques,
domain knowledge and problem-solving/critical thinking will equip individuals with the necessary skills for a successful career in either data science or data analytics.
Future Growth and Trends in Both Industries
Data science and data analytics are two fields that continue to experience significant growth and show no signs of slowing down. As technology advances, the amount of data being generated increases exponentially, creating a need for professionals who can analyze and make sense of this vast amount of information.
In the field of data science, one trend that is expected to continue growing is the use of machine learning algorithms. These algorithms allow computers to learn from data without being explicitly programmed, enabling them to make predictions and decisions based on patterns they discover. This has applications in various industries such as healthcare, finance, marketing, and more.
Another area that holds great potential for both data science and data analytics is the Internet of Things (IoT). With the proliferation of connected devices, there is an enormous amount of valuable data being generated every second. Professionals in these fields will play a crucial role in extracting insights from IoT-generated data to drive informed decision-making.
Furthermore, as businesses become increasingly aware of the importance of leveraging data for strategic decision-making, there will be a growing demand for skilled professionals who can not only analyze large datasets but also communicate their findings effectively. The ability to translate complex technical concepts into actionable insights will be highly sought after by employers.
Additionally, with advancements in cloud computing technologies and storage capabilities, companies are now able to store massive amounts of structured and unstructured data at a fraction of previous costs. This opens up opportunities for both aspiring practitioners looking to gain hands-on experience with big datasets as well as established professionals seeking new challenges.
Ethical considerations surrounding privacy concerns and responsible use of personal or sensitive information are likely to shape future trends in both industries. Data scientists and analysts must navigate these ethical dilemmas while ensuring compliance with regulations such as GDPR or CCPA.
In conclusion, the field’s future growth trajectory looks promising due to technological advancements like machine learning algorithms integration into diverse industries, the rise of IoT-generated data, the need for effective communication skills in
Conclusion
Data science and data analytics are two distinct fields that play crucial roles in extracting insights and making informed decisions from vast amounts of data. While they share some similarities, such as the use of statistical techniques and programming skills, their primary focus areas differ significantly.
Data science encompasses a broader scope, combining computer science, mathematics, statistics, and domain knowledge to uncover patterns and trends in complex datasets. It requires expertise in machine learning algorithms, predictive modeling, data visualization techniques, and a deep understanding of business objectives. Data scientists are often responsible for developing new methodologies to solve real-world problems using cutting-edge technologies.
On the other hand, data analytics is more focused on analyzing historical data to gain actionable insights into past performance or current trends. Analysts utilize various tools like SQL queries or visualization software to identify patterns that can help organizations make strategic decisions. They work closely with stakeholders to understand their needs and provide them with valuable reports or dashboards that drive business growth.
Both fields offer promising career opportunities for those who possess the necessary skills and passion for working with big data. However, it’s important to note that these industries are constantly evolving due to advancements in technology and an increasing demand for data-driven decision-making.
To succeed in either field, individuals need a strong foundation in mathematics/statistics along with proficiency in programming languages like Python or R. Additionally, having good communication skills is vital as professionals must effectively translate complex findings into meaningful insights for non-technical stakeholders.
Looking ahead at future growth prospects for both industries shows no signs of slowing down. As businesses continue to generate massive amounts of data every day across various sectors such as finance, healthcare, marketing, and e-commerce; the need for skilled professionals who can extract value from this wealth of information will only increase.
In summary, data science focuses on solving complex problems through advanced mathematical models, machine learning algorithms, and domain expertise.
Data analytics, on the other hand, is geared towards deriving actionable insights by analyzing historical data.