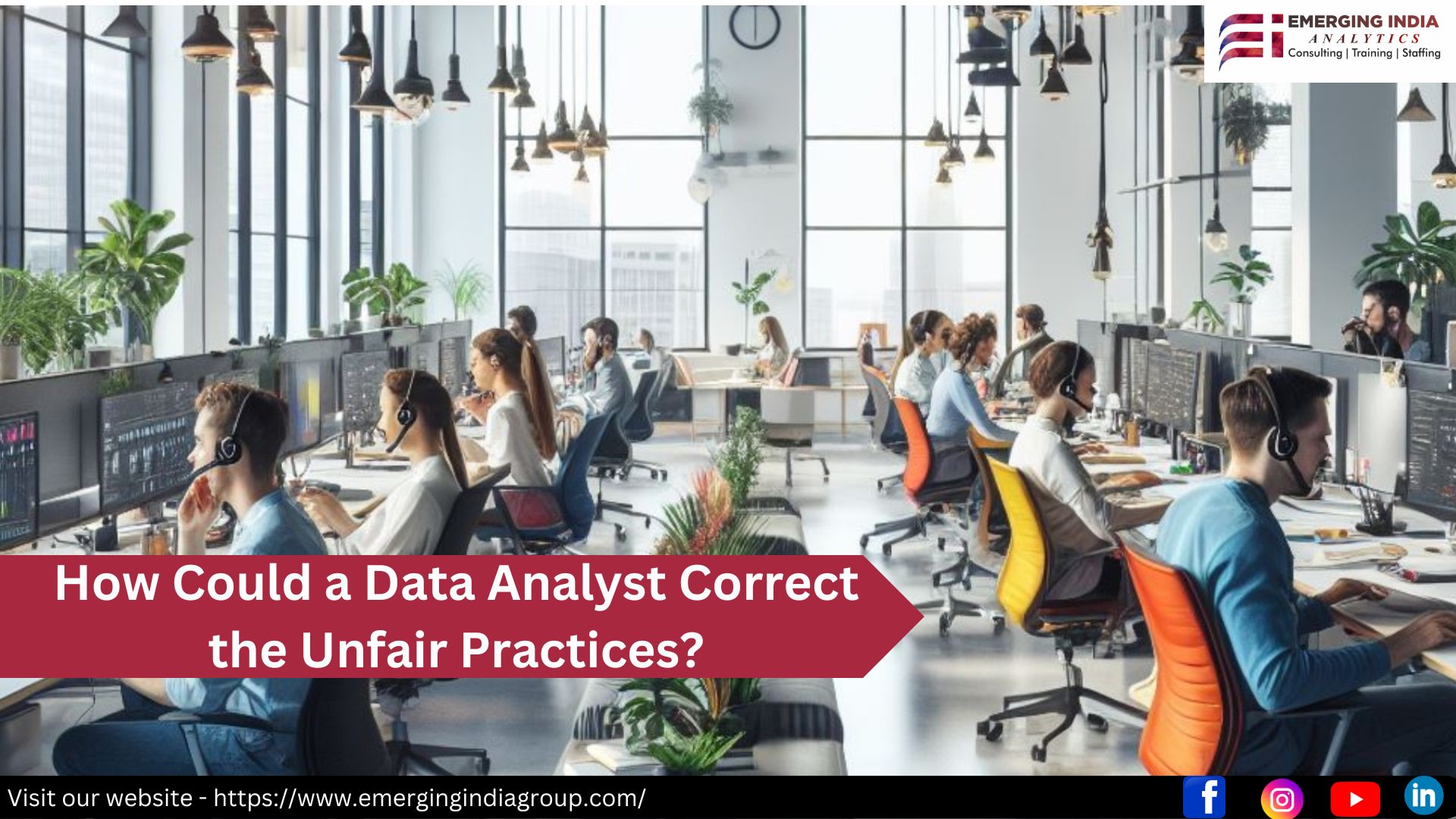
Data analysis is a crucial aspect of business decision-making. It helps organizations to make informed decisions based on data-driven insights. However, the accuracy and reliability of the data used for analysis are paramount to ensure that the decisions made are sound and unbiased.
Unfair practices in data analysis can lead to incorrect conclusions, which can have serious consequences for businesses. Therefore, it’s important to correct any unfair practices in data analysis.
In this article on “How could a data analyst correct the unfair practices”. We’ll discuss the importance of correcting unfair practices in data analysis and provide a brief overview of the steps that data analysts can take to correct unfair practices.
We’ll cover areas such as verifying the reliability and representativeness of the data, using appropriate statistical methods to avoid bias, regularly reviewing and auditing analysis processes to ensure fairness, using diverse data science teams, providing diversity training to data scientists, testing for algorithm bias, and more. By following these steps, data analysts can ensure that their analysis is fair, unbiased, and accurate.
What are Unfair Practices in an Organization?
Unfair practices refer to any actions or decisions taken by an organization that are unethical, discriminatory, or illegal. These practices can take many forms, such as workplace discrimination, unethical business practices, conflict of interest, abuse of power, harassment, retaliation, and more.
Unfair practices can have serious consequences for both the organization and its employees. They can lead to a toxic work environment, low employee morale, and even legal actions.
Therefore, it’s important for organizations to have strong corporate governance policies in place to prevent unfair practices from occurring. This includes having clear policies on organizational culture, whistleblowing, and more.
By promoting a culture of fairness and transparency, organizations can ensure that they are operating ethically and in the best interests of their employees and stakeholders.
What are the Examples of Unfair Practices in Data Analysis?
Unfair practices or limited knowledge while selecting or treating the data can hamper the business and trade practice, here are some examples of unfair practices:
Discrimination: Selecting and filtering data based on race, gender, age, religion, or other personal characteristics.
Bias: Selecting or filtering based on personal opinions or beliefs and it highly influences the decisions or actions.
Unethical practices: Engaging in actions that are morally wrong or violate ethical standards like generating forged data, data leakage, and selling out confidential data.
Deceptive practices: Generating data by misleading consumers through false advertising, misrepresentation of products or services, or other deceptive tactics.
Misrepresentation: Providing false information about a product, service, or situation.
These are just a few examples of unfair practices. It’s important to be aware of these practices and take steps to prevent them from occurring. What Steps Do Data Analysts Take to Ensure Fairness?
These are the necessary steps data analysts take to ensure fairness in the organization.
Verifying the Reliability and Representativeness of the Data
Data reliability and representativeness are crucial aspects of data analysis. It’s important to ensure that the data used for analysis is accurate, unbiased, and representative of the population being studied. Data analysts can check the source of the data, identify any potential biases, and verify that the data is relevant to the research question.
Triangulation can be used to verify the accuracy of the data by comparing it with other sources. Data analysts can check for potential biases in the data by examining the data collection process and identifying any factors that may have influenced the results.
Appropriate sampling techniques can be used to ensure that the data is representative of the population being studied. Data analysis software such as Tableau, SAS, R, Python, etc. uses various statistical and logical methods and techniques to analyze data accurately and efficiently.
A typical methodology for data analysis includes several steps such as defining research questions, collecting relevant data, cleaning and processing the data, analyzing the data using appropriate statistical techniques, and presenting the results clearly and concisely.
Using Appropriate Statistical Methods to Avoid Bias
Data analysts should use statistical methods appropriate for the type of data being analyzed to avoid bias. Statistical methods can help identify and correct potential biases in the data.
Propensity score matching, instrumental variables, difference-in-differences, regression discontinuity design, matching methods, weighting methods, randomized controlled trials, and natural experiments are some of the statistical techniques that can be used to correct for bias in the data. These techniques can help ensure that the analysis is fair and unbiased.
For example, propensity score matching can be used to match job applicants with similar characteristics and compare their hiring outcomes to correct for potential biases in the data.
Regularly Reviewing and Auditing Analysis Processes to Ensure Fairness
It’s important to ensure that the data used for analysis is accurate, unbiased, and representative of the population being studied. Data analysts can check the source of the data, identify any potential biases, and verify that the data is relevant to the research question.
Triangulation can be used to verify the accuracy of the data by comparing it with other sources. Data analysts can check for potential biases in the data by examining the data collection process and identifying any factors that may have influenced the results.
Appropriate sampling techniques can be used to ensure that the data is representative of the population being studied. Data analysis software such as Tableau, SAS, R, Python, etc. uses various statistical and logical methods and techniques to analyze data accurately and efficiently.
A typical methodology for data analysis includes several steps such as defining research questions, collecting relevant data, cleaning and processing the data, analyzing the data using appropriate statistical techniques, and presenting the results clearly and concisely.
Using Diverse Data Science Teams
In data science, diversity in teams enhances bias identification and brings diverse perspectives to the table. The wisdom of the crowd highly influences the data analysis. This diversity, coupled with an inclusive environment, promotes fairness in analysis and bias mitigation.
The amalgamation of different perspectives fosters psychological safety, encouraging open dialogue and innovative ideas. The team’s cultural competence further strengthens this dynamic, ensuring respect for all cultures and reducing cultural biases. Thus, a diverse and inclusive team can significantly improve the fairness and robustness of data analysis.
Providing Diversity Training to Data Scientists
In a business context, diversity training for data scientists is a strategic investment. It equips them to identify and rectify unconscious bias, fostering an environment of inclusive data science. This training, encompassing diversity, inclusion, and cultural awareness, ensures fair data analysis and promotes ethical data science practices.
It cultivates a culturally competent data science team capable of leveraging data for the betterment of the organization. By implementing these methods, data analysts can rectify unfair practices, ensuring that the insights derived are unbiased and representative of all customer segments.
This not only enhances the accuracy of business insights but also contributes to ethical and fair practices in the organization’s data-driven decision-making process.
Testing for Algorithm Bias
In the business context, algorithm bias testing is crucial as analytics bias often stems from incomplete data sets and a lack of context. Contextual analysis can mitigate this, providing a comprehensive understanding of the data and reducing algorithm bias.
Fairness testing ensures unbiased data analysis, is crucial for bias mitigation and promoting model transparency, and is vital for accountability in the era of Explainable AI and Responsible AI. For instance, in loan approval processes, if the algorithm used is biased against certain demographics due to skewed data, it could lead to unfair practices. Regular algorithm bias testing can rectify such issues, ensuring fairness and transparency for all applicants.
Case Study: TechGiant Inc. – Correcting Unfair Practices in Hiring
TechGiant Inc., a leading technology company, was facing criticism for a lack of diversity in its workforce. The company’s leadership recognized the need for change and initiated a comprehensive hiring process fairness review.
Problem Identification
Upon reviewing their hiring data, TechGiant Inc. identified hiring bias as a significant contributor to unfair hiring practices. They found that unconscious biases during the resume screening and interview stages were leading to unfair outcomes, affecting the company’s ability to hire a diverse workforce.
Solution Implementation
To establish fair hiring practices, TechGiant Inc. implemented several measures for bias mitigation:
- Inclusive Hiring Strategies: The company revised its job descriptions to be gender-neutral and ensured representation from diverse groups in hiring panels to promote workplace diversity.
- Hiring Process Review: TechGiant Inc. conducted a thorough analysis of its hiring data using advanced analytics tools like Tableau and Power BI. This helped them identify stages where bias was most prevalent.
- Unconscious Bias Training: The company provided training to all employees involved in the hiring process using different platforms. This training helped them understand their biases and make more objective decisions.
- Data-Driven Hiring Practices: TechGiant Inc. used AI algorithms to screen resumes, focusing on skills and experiences rather than personal attributes. They used AI platforms like Pymetrics and HireVue for this purpose.
Ensuring Algorithmic Fairness
However, they were mindful of algorithmic fairness. They regularly reviewed the algorithm using fairness testing tools like IBM’s AI Fairness 360 and Google’s What-If Tool to ensure it wasn’t perpetuating existing biases.
Outcome
Over time, these measures significantly improved diversity in their workforce, demonstrating that with conscious effort and strategic planning, data analysts can correct unfair practices and foster a more inclusive work environment.
Conclusion
In conclusion, unfair practices can have serious repercussions if left unaddressed. However, data analysts can proactively detect and rectify such issues. This includes verifying data reliability, using appropriate statistical methods, regularly auditing processes, building diverse teams, providing training, and testing for algorithmic bias.
The case study of TechGiant Inc. demonstrates how a thorough review along with bias mitigation strategies and data-driven hiring can transform unfair practices into more ethical, inclusive processes. While eliminating bias may not be possible, consistent efforts to promote algorithmic fairness, diversity, and transparency can go a long way in correcting unfair practices in data analysis.
Organizations that make fairness a priority can derive ethical insights, take informed actions, and build an environment of trust.