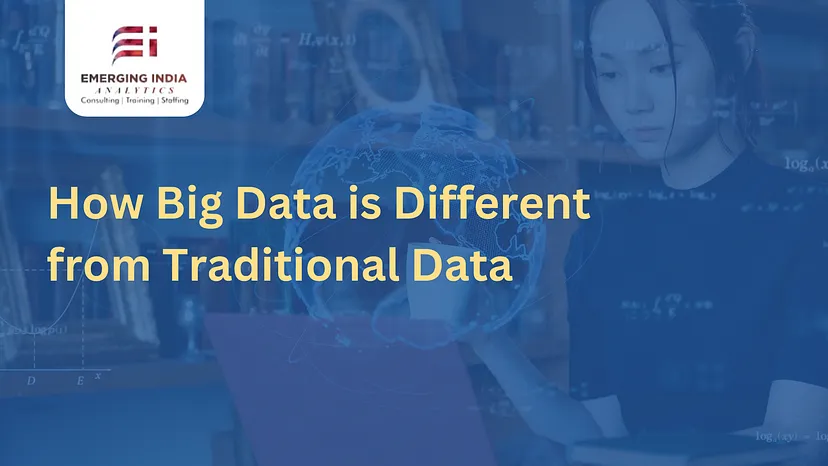
Introduction to Big Data
In today’s digital age, data is being generated at an unprecedented rate. From social media posts and online transactions to sensor readings and customer preferences, every click, swipe, and interaction produces a vast amount of information. This explosion of data has paved the way for big data — a game-changing concept that promises to transform how we understand and utilize information.
Big data refers to extremely large datasets that are too complex or cumbersome for traditional data processing methods to handle effectively. It encompasses not only structured information but also unstructured or semi-structured data from various sources. The sheer volume, velocity, variety, and veracity of big data present unique challenges and opportunities that set it apart from traditional data analysis.
In this blog post, we will delve into the world of big data — exploring its characteristics, key differences from traditional approaches, types of big data encountered in real-world scenarios, as well as its challenges and advantages in processing. So fasten your seatbelts as we embark on an exciting journey through the realm of big data analytics!
Characteristics of Big Data
When it comes to big data, there are several key characteristics that set it apart from traditional data. Big data is all about volume. It refers to vast amounts of information that cannot be easily managed or analyzed using traditional methods.
Another characteristic is velocity. Big data is generated and updated at an incredible speed, requiring real-time processing and analysis. This rapid flow of data demands efficient systems that can handle the continuous influx of information.
Variety is also a defining feature of big data. Unlike traditional structured databases, big data encompasses diverse types and formats such as text, images, videos, social media posts, sensor readings, and more. The ability to process this heterogeneous mix is essential for extracting valuable insights.
Big data also exhibits variability in terms of its inconsistency and unpredictability. Data sources may have different levels of accuracy or reliability which adds complexity to the analysis process. Moreover,
the sheer magnitude and diversity of big data mean that it often requires distributed computing frameworks for effective storage and processing.
Last but certainly not least important is veracity — the quality or trustworthiness of the collected information. With big datasets sourced from various channels like social media platforms or IoT devices,
data quality becomes a critical consideration since inaccuracies could lead to flawed conclusions.
Understanding these characteristics allows organizations to harness the power of big data by implementing appropriate technologies and strategies for collecting, managing, analyzing, and deriving meaningful insights from this wealth of information.
Traditional Data vs Big Data: Key Differences
Traditional Data vs Big Data: Key Differences
When it comes to data, size does matter. Traditional data refers to a more structured and manageable type of information that can be easily stored and analyzed using traditional database systems. On the other hand, big data is characterized by its sheer volume, velocity, variety, and veracity.
In terms of volume, traditional data sets are typically smaller in size compared to big data sets. While traditional data can be measured in gigabytes or terabytes, big data can reach petabytes or even exabytes. This exponential increase in volume requires specialized tools and techniques for storage and processing.
Velocity is another key difference between traditional data and big data. Traditional datasets are usually static or updated periodically. In contrast, big data streams continuously from various sources such as social media feeds or sensor networks in real time.
Variety is yet another aspect where traditional data differs from big data. Traditional datasets are often well-structured with a predefined schema that allows for easy analysis. Big Data encompasses unstructured and semi-structured formats such as text files, images, videos, and social media posts — making it a challenge to extract meaningful insights without proper preprocessing methods.
We have veracity — the reliability or trustworthiness of the dataset itself. Traditional datasets tend to have higher levels of accuracy due to their structured nature; however, big data is prone to inaccuracies and noise due to various sources of errors and uncertainties in the data collection process.
This is why it’s important to implement validations and algorithms for cleansing big data sets before analysis has taken place
Types of Big Data
When it comes to big data, there isn’t a one-size-fits-all approach. In fact, big data can be classified into various types based on its sources and characteristics. Let’s explore some of the different types of big data.
We have structured data. This type of data is highly organized and follows a specific format. It can easily fit into traditional databases and spreadsheets. For example, customer information like names, addresses, and purchase history would fall under structured data.
Next up is unstructured data. Unlike structured data, this type doesn’t conform to a predefined format or model. Examples include social media posts, emails, videos, and audio files. Unstructured data requires advanced techniques such as natural language processing to extract meaningful insights.
Then we have semi-structured data which lies somewhere in between structured and unstructured forms. It contains both organized elements (like tags or labels) as well as unorganized components (like comments or descriptions). XML files and JSON documents are common examples of semi-structured data.
Moving on to temporal or time-series data — this refers to any form of information that is recorded over time intervals. It includes stock market prices, weather patterns, sensor readings from IoT devices — anything that captures changes over time.
Last but certainly not least is spatial or geospatial data which involves geographical locations and their attributes represented in digital form using coordinates or addresses. Geospatial datasets help analyze location-based trends for urban planning purposes or even tracking the spread of diseases across regions.
These are just some examples highlighting the diverse nature of big data types out there! Understanding these distinctions enables businesses to develop tailored strategies for handling different kinds
Challenges and Advantages of Processing Big Data
Processing big data comes with its fair share of challenges and advantages. One of the biggest challenges is the sheer volume of data that needs to be processed. Traditional data processing methods often fall short when it comes to handling such massive amounts of information. However, advancements in technology have made it possible for businesses to leverage big data analytics tools and platforms that can handle this mammoth task.
Another challenge is the variety of data types involved in big data processing. Unlike traditional structured databases, big data encompasses unstructured and semi-structured data from various sources like social media feeds, sensor logs, videos, and more. This requires sophisticated algorithms and techniques to extract meaningful insights from diverse datasets.
The velocity at which big data is generated poses yet another challenge. The speed at which new information is being created necessitates real-time or near-real-time analysis for timely decision-making. Processing huge volumes of streaming data requires robust infrastructure and powerful computational capabilities.
Despite these challenges, there are numerous advantages associated with processing big data as well. For starters, analyzing large datasets allows organizations to gain valuable insights into customer behavior patterns, market trends, and other crucial factors affecting their business operations.
Big Data analytics also enables companies to make informed decisions based on evidence rather than intuition alone. By leveraging advanced analytical models and algorithms, businesses can identify hidden correlations between different variables within their datasets.
Furthermore, processing big data opens up opportunities for innovation by uncovering new product ideas or service offerings that cater specifically to customer demands identified through careful analysis.
In conclusion (not conclusive), while the challenges posed by processing big Data cannot be ignored; the benefits are equally significant if handled properly.
Therefore it becomes essential for organizations across industries — whether they’re in finance or healthcare -to invest in technologies that enable them to effectively process, analyze , and derive actionable insights from Big Data
Real-life Applications of Big Data
Big data has revolutionized various industries by providing valuable insights and driving decision-making processes. Here are some real-life applications where big data is making a significant impact.
1. Healthcare: Big data analytics helps in patient monitoring, predicting disease outbreaks, improving diagnosis accuracy, and personalized treatments. By analyzing large volumes of medical records and genomic data, healthcare providers can make informed decisions and improve patient outcomes.
2. Retail: Big data enables retailers to understand consumer behavior, optimize inventory management, and personalize marketing campaigns. With the help of customer purchase history, social media interactions, and demographic information, retailers can offer targeted promotions tailored to individual preferences.
3. Finance: In the finance industry, big data plays a crucial role in fraud detection and risk assessment. It allows financial institutions to analyze patterns in transactions to identify potential fraudulent activities quickly. Moreover, big data analysis helps in portfolio optimization for investment firms.
4. Transportation: The transportation sector uses big data for traffic management systems that optimize routes based on real-time traffic conditions. This reduces congestion on roads and improves fuel efficiency for vehicles. Additionally, ride-sharing platforms use big data algorithms to match drivers with passengers efficiently.
5. Energy Sector: The energy industry utilizes big data analytics to monitor power grids’ stability by analyzing sensor readings from smart meters installed at consumers’ locations.
The analysis helps predict demand fluctuations accurately, reducing power outages & optimizing energy distribution.
These are just a few examples illustrating how big data is transforming various sectors across the globe.
Businesses have realized the immense value hidden within their vast amounts of structured or unstructured information.
Big Data provides actionable insights that enable organizations to stay competitive, gain new opportunities, and deliver enhanced products or services.
It’s clear that harnessing the power of big data opens up endless possibilities for innovation
Conclusion
Big data has revolutionized the way we handle and analyze vast amounts of information. Its unique characteristics, such as volume, velocity, variety, and veracity, set it apart from traditional data sources. With big data analytics, organizations can gain valuable insights that were previously unimaginable.
While traditional data focuses on structured information collected from predetermined sources, big data encompasses unstructured and semi-structured data from diverse sources like social media platforms, sensors, and machines. This diversity allows for a more comprehensive understanding of customer behavior patterns and market trends.
Processing big data comes with its challenges. The sheer scale of the data requires specialized tools and technologies to effectively store, manage, process, and analyze it. Privacy concerns also arise due to the nature of personally identifiable information present in large datasets.
However daunting these challenges may seem, the advantages offered by big data are undeniable. From improving business operations through predictive analytics to enhancing healthcare outcomes through personalized medicine approaches — big data has endless potential for innovation across industries.
Real-life applications demonstrate how big data is transforming various sectors. Healthcare providers can utilize patient-generated health records to develop personalized treatment plans. Retailers can leverage customer purchase history to offer tailored recommendations that improve customer satisfaction while boosting sales revenue.