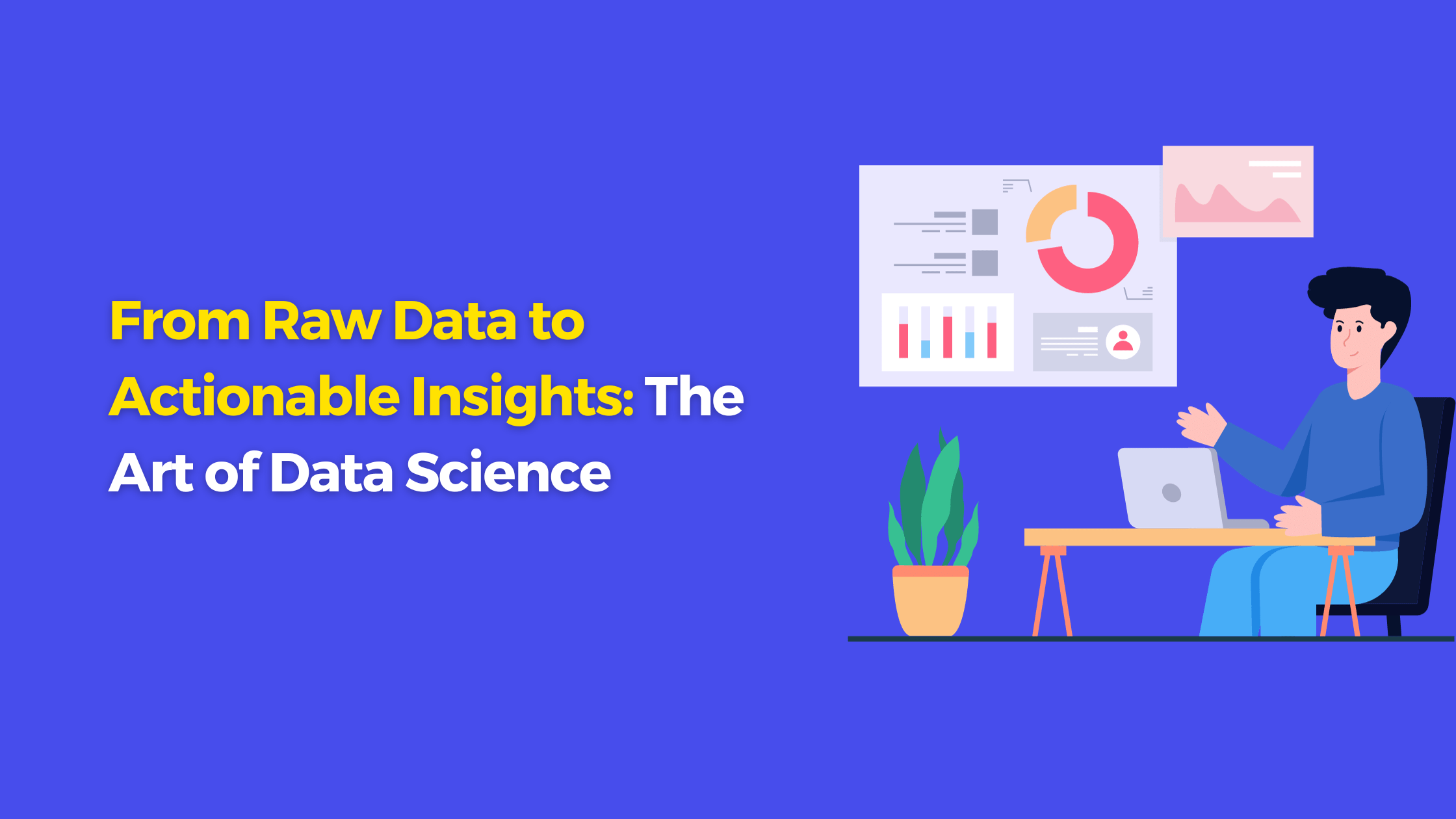
Welcome to the world of data science, where raw information transforms into valuable insights that drive innovation and decision-making. In today’s digital era, data has become the lifeblood of businesses across industries, and harnessing its power requires a unique blend of analytical skills and technological prowess. Enter data science – the art of extracting meaningful patterns and trends from vast amounts of data.
But what exactly is data science? How does it differ from artificial intelligence or machine learning? And how can this field revolutionize industries as diverse as healthcare, finance, and marketing? Join us as we unravel the mysteries surrounding data science, explore its applications in various domains, uncover the skills needed to thrive in this fast-paced field, and glimpse into its promising future.
So grab your curiosity by the hand and embark on this exciting journey through the realm of data science! Whether you’re an aspiring professional seeking to break into this field or simply someone who wants to understand how algorithms can shape our everyday lives – there’s something here for everyone. Let’s dive in!
What is Data Science
Data science is the practice of extracting insights from data using a combination of statistical analysis, machine learning techniques, and domain expertise. It involves collecting, cleaning, and analyzing large datasets to uncover patterns that can drive informed decision-making in various industries.
Data Science in Simple Words
Data science is the art of extracting meaning from data. It involves using techniques like statistical analysis and machine learning to uncover patterns and insights that can drive informed decision-making. In simpler words, it’s all about turning raw data into actionable information!
Data Science vs Artificial Intelligence vs Machine Learning
Data Science, Artificial Intelligence, and Machine Learning are often used interchangeably, but they have distinct differences. Data Science focuses on extracting insights from data, AI involves creating intelligent systems, while ML enables machines to learn and make predictions based on patterns in data.
The Data Science Process
The Data Science Process involves a systematic approach to extract, analyze, and interpret data in order to derive valuable insights. It encompasses various stages such as data collection, preprocessing, modeling, evaluation, and deployment. Each step plays a crucial role in transforming raw data into actionable knowledge.
The Data Science Lifecycle
The data science lifecycle is a structured approach that involves defining the problem, collecting and preparing data, analyzing and modeling it, and finally deploying the results. It’s a continuous process of discovery and iteration to extract valuable insights from raw data.
Data Science Techniques
Data Science Techniques are the secret sauce that turns raw data into valuable insights. From exploratory analysis to predictive modeling, these techniques help uncover patterns, make predictions, and solve complex problems. It’s like detective work for data nerds!
Roles in Data Science
Data Science involves a team of experts with different roles. The Data Scientist applies algorithms to extract insights, the Data Analyst interprets data patterns, and the Data Engineer builds infrastructure for data storage and processing. Each role contributes to the success of a data-driven project.
Data Scientist
Data Scientists are the masterminds behind transforming raw data into valuable insights. They have a unique blend of skills in programming, statistics, and domain knowledge that allows them to uncover patterns and trends hidden within complex datasets. Their expertise drives informed decision-making and innovation.
Data Analyst
Data Analysts play a crucial role in the world of data science. They are experts at interpreting and analyzing large sets of data to uncover valuable insights. With their skills, businesses can make informed decisions and drive growth.
Data Engineer
Data engineers play a crucial role in data science projects. They are responsible for building and maintaining the infrastructure needed to collect, store, and process large amounts of data. Without them, data scientists wouldn’t have the raw material they need to work their magic.
Applications and Uses of Data Science
Data Science is everywhere! From predicting customer behavior to optimizing supply chains, its applications are vast. It helps healthcare professionals make accurate diagnoses and enables financial institutions to detect fraud. The possibilities are endless, making Data Science an essential tool in various industries.
Examples of Data Science
Data Science is everywhere, powering countless real-world applications. It helps Netflix recommend movies, predicts customer preferences for Amazon, and even aids in medical research. From finance to healthcare to marketing, data science has endless possibilities that continue to transform industries.
Data Science in Different Industries
Data science is revolutionizing industries across the board. From finance to healthcare, data-driven insights are driving innovation and improving decision-making processes. Whether it’s predicting customer behavior or optimizing supply chains, data science has become a cornerstone in various sectors.
Prerequisites and Skills in Data Science
Data Science Eligibility: A strong foundation in mathematics and statistics is crucial. Programming skills, especially in languages like Python or R, are also important. Additionally, data visualization and communication skills are valuable for effectively conveying insights to stakeholders. Keep learning!
Data Science Eligibility
Data Science Eligibility requires a strong foundation in mathematics, statistics, and programming. A degree in computer science or related fields is preferable, but self-learning and online courses can also help you gain the necessary skills. Stay motivated and keep learning!
Learning Data Science on Your Own
If you have a passion for data and want to dive deep into the world of Data Science, you can teach yourself! With online resources, tutorials, and practice projects, you can develop the necessary skills at your own pace. Embrace self-learning and unlock endless possibilities!
Tools and Technologies in Data Science
Data Science is a rapidly evolving field, and there are numerous tools and technologies that enable professionals to analyze data effectively. From programming languages like Python and R to libraries such as TensorFlow and scikit-learn, these resources empower data scientists in their quest for actionable insights.
Future of Data Science
The Future of Data Science is bright and promising. With advancements in technology, data scientists will continue to play a crucial role in solving complex problems and driving innovation across industries. Exciting developments lie ahead as we harness the power of data to shape a better future.
Conclusion
Data science is a rapidly growing field that holds immense potential for businesses and industries across the globe. It involves extracting valuable insights from raw data to drive informed decision-making and solve complex problems. By combining statistical analysis, machine learning techniques, and programming skills, data scientists have the power to unlock hidden patterns and trends.
Throughout this article, we have explored what data science is all about, its relationship with artificial intelligence and machine learning, as well as the step-by-step process involved in harnessing its power. We have also discussed various roles within the field of data science and highlighted some common applications across different industries.
To embark on a successful journey in data science, it is important to possess certain prerequisites such as knowledge of mathematics, statistics, programming languages like Python or R, and critical thinking abilities. While formal education can provide a solid foundation in this field,
one can also learn data science independently through online courses or by participating in real-world projects.
Furthermore, we touched upon some popular tools and technologies used by data scientists to analyze vast amounts of data efficiently. These include programming languages like Python or R for coding purposes,
as well as specialized software such as Tableau or Apache Hadoop for visualization
and big data processing respectively.