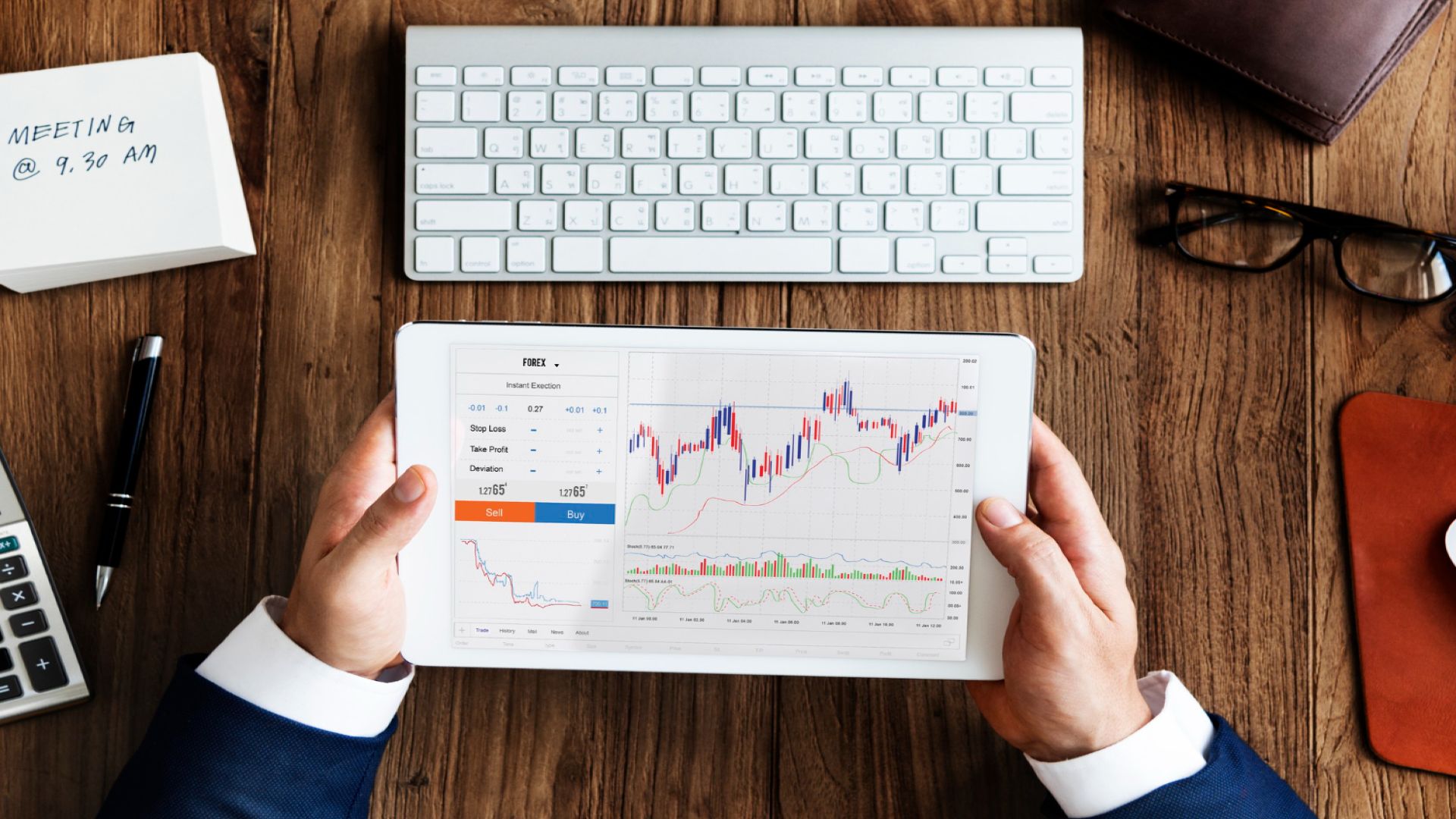
The age of intuition and gut feeling in stock market analysis is fading. Today, a new frontier emerges, driven by the power of data science in stock market. This field unlocks a treasure trove of information, transforming the way we understand and navigate the ever-shifting tides of the market.
Imagine sifting through mountains of financial data, news articles, and social media chatter – all in real-time. Data science empowers us to do just that, uncovering hidden patterns and trends invisible to the naked eye.
This data-driven approach isn’t just about predicting the future. It empowers investors to make informed decisions, optimize portfolios, and mitigate risk. By harnessing the power of data science as well as artificial intelligence in the stock market, we can unlock the true potential, creating a more efficient and potentially rewarding experience for all participants.
The Evolution of Stock Market Analysis
Stock market analysis has traditionally relied on fundamental analysis, which involves poring over company financials and economic indicators, and technical analysis, focusing on historical price charts and trading patterns. While valuable, these methods can be time-consuming, subjective, and limited by the available data.
The emergence of “big data in stock markets” has fundamentally changed the game. “Data science in stock trading” now allows us to analyze massive datasets, including news feeds, social media sentiments, and real-time market movements. By employing sophisticated algorithms and machine learning, this “stock market analysis using big data” can uncover hidden correlations, predict market trends with greater accuracy, and identify undervalued opportunities that might have been missed with traditional methods.
Key Concepts of Data Science in Stock Market Analysis
Data science has emerged as a game-changer in stock market analysis, offering a powerful arsenal of techniques that go beyond traditional methods. Here, we delve into three key concepts that are transforming the way investors approach the market:
- Machine Learning for Stock Trading: Envision an algorithm capable of scrutinizing extensive historical data, such as price fluctuations, news reports, and even social media sentiments. This exemplifies the prowess of machine learning. Through analysis of large datasets, these algorithms can unveil intricate patterns and correlations that conventional methods might overlook. Consequently, they can forecast future trends and potentially reveal lucrative opportunities in the market. The field of ‘data science in stock trading’ witnesses continual advancement of machine learning algorithms, providing investors with a potent tool to make well-informed decisions and potentially surpass market performance.
- Data Mining: Unveiling Hidden Gems: Traditionally, stock analysis relied on readily available data points. However, “data science in stock trading” introduces the concept of data mining, a process akin to sifting through mountains of financial data to unearth valuable insights. Data mining techniques can uncover subtle correlations that might otherwise go unnoticed. For instance, data mining could reveal a link between positive social media sentiment towards a company and a subsequent rise in its stock price. This hidden knowledge empowers investors to make more strategic decisions and potentially identify undervalued opportunities.
- Predictive Modeling: Forecasting the Market: By leveraging the insights gained from machine learning and data mining, “data science in stock trading” allows for the creation of predictive models. These models analyze historical data and incorporate real-time information to estimate future stock prices or even predict potential market fluctuations. While not a crystal ball, predictive modeling offers valuable forecasting capabilities that can help investors develop more informed strategies and mitigate risks associated with market volatility.
These combined data science techniques are revolutionizing stock market analysis. By providing data-driven insights and predictive capabilities, they empower investors to navigate the complex world of the market with greater confidence and potentially achieve their financial goals.
Benefits of Data Science in Stock Market Analysis
Traditionally, stock market analysis relied on fundamental analysis of company financials and technical analysis of historical price charts. While valuable, these methods can be time-consuming, subjective, and limited by the sheer volume of data available today. Data science in stock market analysis offers a powerful solution, leveraging cutting-edge techniques like machine learning and artificial intelligence (AI) to unlock the true potential of this vast data. Here are 10 key benefits of incorporating data science into your investment strategy:
- Enhanced Accuracy: Data science allows for the analysis of massive datasets, including historical price movements, news articles, social media sentiment, and economic indicators. This comprehensive approach empowers investors to identify subtle patterns and relationships that might be missed with traditional methods. For example, machine learning algorithms can analyze years of data to predict future trends with greater accuracy, potentially leading to more profitable investment decisions.
- Improved Efficiency: Sifting through mountains of financial data can be a daunting task. Data mining techniques, a core component of data science, automate this process, extracting valuable insights and correlations from vast datasets. This frees up time for investors to focus on developing investment strategies and making informed decisions, rather than getting bogged down in data collection and analysis.
- Reduced Risk Management: Data science empowers investors to make data-driven decisions that can mitigate risk. By analyzing historical data and incorporating real-time information, data science can be used to create risk models. These models can identify potential market downturns or company vulnerabilities, allowing investors to adjust their portfolios accordingly.
- Uncovering Hidden Gems: Traditional analysis often focuses on well-established companies with readily available data. Data science offers the ability to uncover hidden gems in the market through data mining techniques. By analyzing social media sentiment or alternative data sources like satellite imagery, data science can identify promising companies that might be overlooked by traditional methods.
- Discovery of Alpha: Alpha is the measure of an investment’s performance that’s not explained by the overall market. Data science techniques like machine learning can analyze vast datasets to identify trading opportunities with the potential for alpha generation. For instance, an algorithm might discover a correlation between a specific news event and a particular stock’s price movement, allowing investors to capitalize on this opportunity.
- Algorithmic Trading: Data science paves the way for algorithmic trading, where pre-programmed algorithms execute trades based on specific criteria. This allows for faster and more efficient trading compared to manual methods, reducing the impact of human emotions on investment decisions.
- Backtesting Strategies: Data science allows investors to backtest their trading strategies using historical data. This provides valuable insights into the potential performance of a strategy before deploying real capital. By testing and refining strategies using data science techniques, investors can increase their chances of success in the market.
- Customization: Data science allows investors to tailor their analysis to their specific needs and risk tolerance. By leveraging various data sources and models, investors can create a personalized approach to stock market analysis that aligns with their investment goals.
- Democratization of Finance: Data science tools and platforms are becoming increasingly accessible, even for individual investors. This empowers even those without a financial background to leverage sophisticated data analysis techniques in their investment decisions.
- Evolving Landscape: Data science is a rapidly evolving field. New techniques and applications are constantly emerging, providing investors with a continuous stream of innovative tools to navigate the ever-changing market landscape. For instance, the use of natural language processing (NLP) is gaining traction in AI for stock trading, allowing for the analysis of vast quantities of unstructured text data like news articles and analyst reports.
Case Study: A hedge fund utilized data science techniques to analyze social media sentiment surrounding a specific company. They discovered a surge in negative sentiment shortly before a major product recall announcement. By analyzing this data and acting swiftly, the hedge fund was able to avoid significant losses in their holdings of that company’s stock.
By incorporating data science into your investment strategy, you can gain a significant edge in the complex world of the stock market. While data science is not a magic formula for guaranteed success, it provides a powerful toolkit for making informed decisions, identifying promising opportunities, and ultimately achieving your financial goals. Remember, Python is a popular programming language widely used in data science due to its versatility and extensive data science libraries. Exploring Python for stock market analysis can open doors to a world of possibilities.
Challenges and Limitations
While data science in share markets offers a powerful arsenal of tools, it’s not without its challenges and limitations. Here are some key areas to consider:
- Data Quality: The effectiveness of data science hinges on the quality of data used. Big data analytics in stock markets involves massive datasets, but these datasets can be riddled with inconsistencies, errors, and biases. Incomplete or inaccurate data can lead to flawed models and misleading insights. Investors need to critically evaluate data sources and employ data cleaning techniques to ensure the integrity of their analysis.
- Model Complexity: Machine learning models used in AI predicting stock market behavior can become overly complex. These complex models can be difficult to interpret and prone to overfitting, where they perform well on historical data but fail to generalize to new situations. Focusing on simpler, more interpretable models and employing techniques like cross-validation can help mitigate this risk.
- Black Box Problem: Some complex AI models can become opaque, making it difficult to understand how they arrive at their predictions. This “black box problem” can limit investor confidence and make it challenging to identify potential biases within the model. Seeking explainable AI models and prioritizing model interpretability can address this concern.
- Market Inefficiency: The stock market is not perfectly efficient, meaning unexpected events or irrational behavior can still cause fluctuations. Data science models may struggle to predict these “black swan” events. Investors should understand the limitations of data science and combine its insights with other forms of market analysis.
- Evolving Market Dynamics: Financial markets are constantly evolving, with new regulations, economic factors, and technological advancements emerging. Data science models need to be regularly updated and adjusted to account for these changing dynamics. Investors should continuously monitor their models and be prepared to adapt their strategies as the market landscape shifts.
Despite these challenges, data science for stock market analysis remains a powerful tool. By acknowledging its limitations and employing best practices for data quality, model selection, and ongoing monitoring, investors can leverage data science to make informed decisions and navigate the complex world of financial markets.
Real-world Applications
Data science for stock market analysis isn’t just theoretical. Here are some real-world applications showcasing its impact:
- Identifying Hidden Gems: A hedge fund used data mining techniques to analyze social media sentiment and alternative data sources like satellite imagery. They discovered a surge in online searches for a specific athletic shoe brand before a major product launch. By acting on this data, the fund identified an undervalued company and profited significantly when the stock price rose after the launch.
- Algorithmic Trading: High-frequency trading firms use sophisticated algorithms powered by data science to execute trades at lightning speed. These algorithms analyze real-time market data and news feeds, exploiting short-term price fluctuations for potential gains. While not without controversy, algorithmic trading has demonstrably increased market liquidity.
- Risk Management: A large investment bank employs data science to create risk models. These models analyze historical data and market trends to identify potential risks like market crashes or sector-specific downturns. By incorporating these models into their investment decisions, the bank can mitigate risk and protect their clients’ assets.
These examples highlight the transformative power of data science in share market analytics.
Future Trends
The future of data science in stock market analysis is brimming with exciting possibilities:
- Natural Language Processing (NLP): Advances in NLP will allow AI to analyze vast amounts of unstructured text data like news articles, analyst reports, and social media conversations. This will provide deeper insights into market sentiment and potentially predict future trends.
- Alternative Data Sources: Data science will increasingly leverage alternative data sources like satellite imagery, geolocation data, and even weather patterns. These diverse datasets can offer valuable insights not captured in traditional financial data.
- Explainable AI: The development of explainable AI models will address the “black box” problem. Investors will be able to understand how AI models arrive at predictions, fostering trust and enabling better decision-making.
These trends suggest that data science will become even more integrated into stock market analysis. Investors who embrace these advancements will be better equipped to navigate the ever-evolving market landscape.
Conclusion
The power of data science in stock market analysis is undeniable. It offers a data-driven approach to investment decisions, fostering greater accuracy, efficiency, and risk management. While challenges like data quality and model complexity exist, best practices and continuous innovation are paving the way for a future where data science is central to successful share market analytics. As data science evolves, investors and analysts who leverage its power will be well-positioned to thrive in the dynamic world of financial markets.