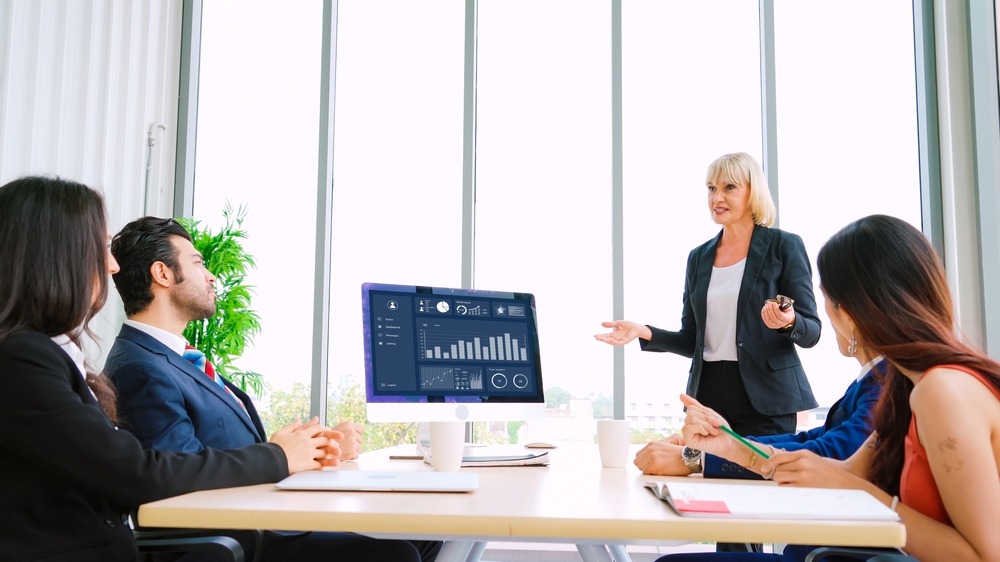
Data science has become a critical tool for businesses seeking to improve decision-making and boost performance. By analyzing data, businesses can gain insights into customer behavior, market trends, and operational performance, among other things. In this article, we’ll explore how businesses can use data science to improve decision-making and boost performance in 2023.
Identify Business Goals and Key Metrics
The first step in using data science for business is to identify your business goals, pain points and the key metrics that will help you measure success. For example, if you want to increase customer satisfaction, you might track metrics like Net Promoter Score (NPS) and customer churn rate. By identifying these metrics, you can begin to collect and analyze data to gain insights into what’s driving them.
Collect and Clean Data
Once you’ve identified your key metrics, the next step is to collect and clean your data. This involves gathering data from various sources, such as customer transactions, social media, and website analytics, and ensuring that it is accurate, complete, and consistent. Data cleaning involves removing any errors or inconsistencies in the data to ensure that it is reliable and useful for analysis.
Use Data Visualization Tools to Gain Insights
Data visualization tools, such as charts, graphs, and dashboards, can help businesses gain insights into their data quickly and easily. These tools can help identify trends, patterns, and anomalies in the data, making it easier for businesses to make informed decisions.
Apply Machine Learning Algorithms
Machine learning algorithms can help businesses analyze their data and make predictions about future trends and behaviors. For example, a machine learning algorithm might analyze customer data to predict which customers are most likely to churn and what actions businesses can take to retain them. Machine learning algorithms can also be used to identify patterns in operational data, such as supply chain and logistics data, to identify opportunities for improvement and cost savings. Basing on the type of data the algorithms are selected and along with the algorithms various methods like Hyper parameter tuning, Cross Validations are also used to make the algorithm more efficient
Implement Predictive Analytics
Predictive analytics involves using historical data and machine learning algorithms to predict future outcomes. For example, a business might use predictive analytics to forecast sales for the next quarter or to predict which products are most likely to be popular with customers. By using predictive analytics, businesses can make more informed decisions and take actions that are more likely to result in positive outcomes.
Utilize Data-Driven Decision-Making
Data-driven decision-making involves using data to inform business decisions. By making decisions based on data, rather than intuition or assumptions, businesses can reduce the risk of making poor decisions and increase the likelihood of success. Data-driven decision-making also enables businesses to be more agile and responsive to changing market conditions.
Continuously Monitor and Improve
Finally, businesses should continuously monitor and improve their data science efforts. This involves tracking key metrics, analyzing data regularly, and making adjustments to strategies and algorithms as needed. By continuously monitoring and improving their data science efforts, businesses can ensure that they are maximizing the value of their data and making informed decisions that drive success.
While the above steps provide a general roadmap for implementing data science in business, it’s important to note that there are additional considerations and best practices that can help businesses achieve even greater success.
For example, businesses should focus on building a strong data culture and ensuring that all employees understand the importance of data and how it can be used to drive success. This may involve investing in data literacy training and education programs to help employees develop the skills they need to analyze and interpret data effectively.
Another important consideration is data privacy and security. Businesses must ensure that they are collecting and using data in a responsible and ethical manner, and that they are taking the necessary steps to protect sensitive information from unauthorized access or disclosure.
Additionally, businesses should consider the role of data science in improving customer experience. By analyzing customer data, businesses can gain insights into customer needs, preferences, and behavior, and use this information to personalize their products and services and deliver more engaging and relevant experiences.
Finally, businesses should be aware of the limitations of data science and the potential for bias in algorithms and models. While data science can provide valuable insights and help businesses make better decisions, it’s important to approach it with a critical eye and ensure that algorithms and models are being used appropriately and ethically.
In conclusion, data science has become a critical tool for businesses seeking to improve decision-making and boost performance. By identifying business goals and key metrics, collecting and cleaning data, using data visualization tools, applying machine learning algorithms, implementing predictive analytics, utilizing data-driven decision-making, and continuously monitoring and improving, businesses can gain valuable insights into their operations and make informed decisions that drive success in 2023 and beyond.