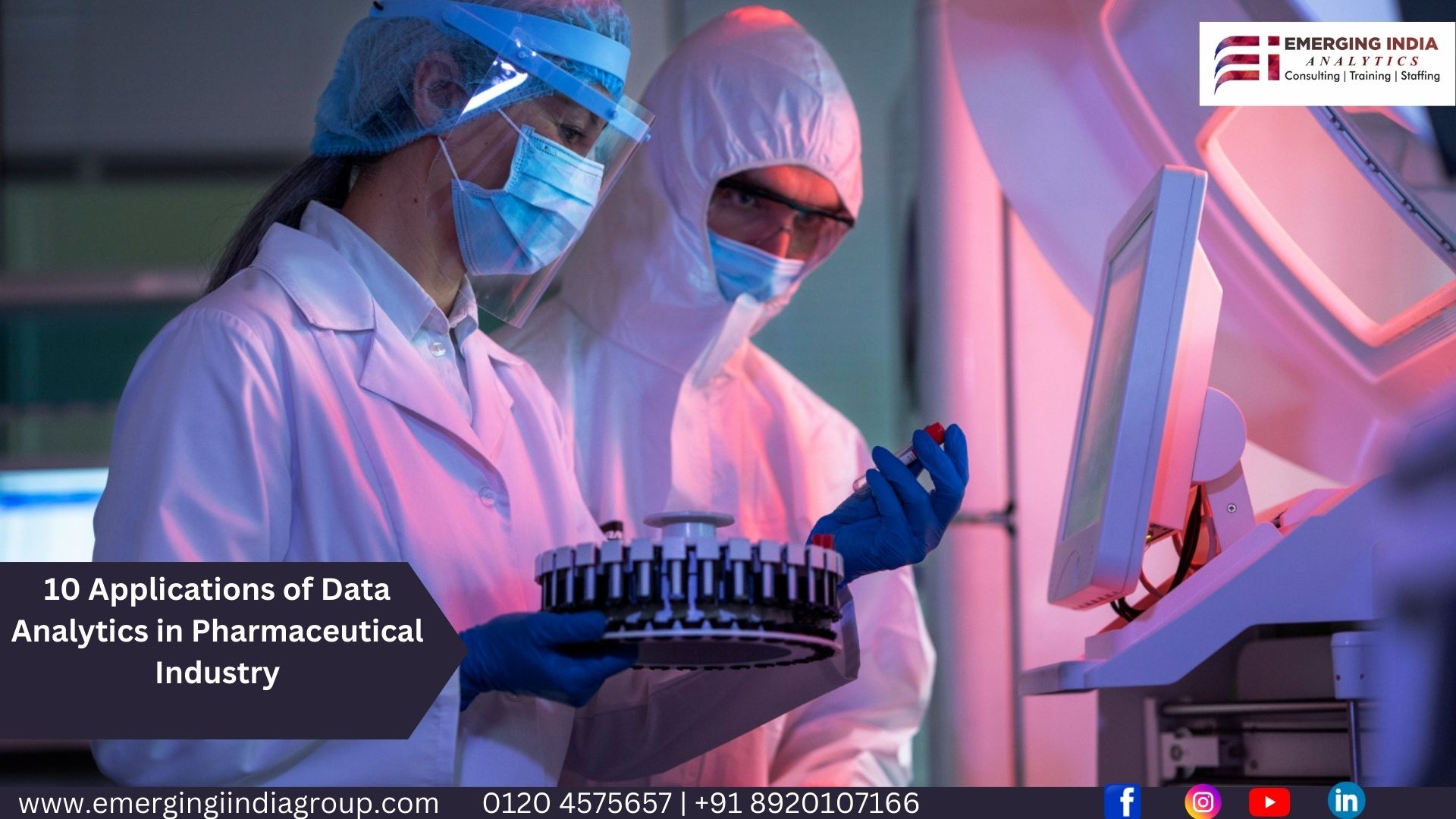
The pharmaceutical industry is undergoing a major transformation driven by data analytics. As companies strive to stay competitive, data analytics for pharmaceutical industry has become indispensable – from drug R&D to safety initiatives. The vast data from clinical trials, health records, and genomics poses challenges. However, advanced techniques like AI and machine learning help uncover insights from this information trove. Data analytics accelerates drug discovery by analyzing patient data to identify drug targets, improve trials, and predict treatment responses. For drug safety, it identifies adverse reactions by analyzing real-world evidence. On the business side, supply chain, sales, and marketing data help optimize operations, efficiency, and data-driven growth. The industry is projected to reach $1.233 trillion by 2025. As data volumes explode, analytics will remain essential to harness insights. Companies embracing analytics will drive innovation, better outcomes, and operational excellence. The pharma industry’s data-driven future has arrived. In this article, we are going to discuss the top 10 applications of data analytics in pharmaceutical industry.
10 Use Cases of Data Analytics in Pharmaceutical Industry
McKinsey estimates that advanced analytics can deliver 15-30% EBITDA gains for the pharma industry over 5 years, potentially accelerating to 45-70% over a decade. This significant impact reflects the potential of predictive modeling to optimize new age therapies and drugs. In short, pharma companies can unlock major value by scaling analytics adoption. These are the 10 use cases of how data analytics is used in pharmaceutical industry.
Drug Discovery and Development:
The use of data analytics in drug discovery and development has become increasingly important in recent years. By analyzing large amounts of data, researchers can identify potential drug targets and design more effective clinical trials. This approach can also help predict patient responses to treatment, leading to the development of safer and more effective drugs. Data analytics in pharma R&D has the potential to revolutionize the drug discovery process, making it faster, more efficient, and more cost-effective. With the help of advanced analytics tools and techniques, researchers can analyze vast amounts of data from a variety of sources, including clinical trials, electronic health records, and genomic data, to gain insights into disease mechanisms and drug efficacy. By leveraging these insights, researchers can develop more targeted and personalized treatments that are tailored to individual patients. Overall, data analytics is a critical component of drug discovery and development, and its importance is only expected to grow in the coming years.
Clinical Trial Optimization
The use of data analytics in the pharmaceutical industry has revolutionized the way clinical trials are designed and conducted. By leveraging advanced analytics tools and techniques, pharmaceutical companies can optimize clinical trial design, patient selection, and data analysis. This data-driven approach leads to more efficient trials, reduced costs, and improved patient outcomes. Data analytics for pharmaceutical companies can help identify the most promising drug candidates, select the right patients for clinical trials, and design trials that are more likely to succeed. By analyzing large amounts of data from a variety of sources, including electronic health records, genomic data, and clinical trial data, researchers can gain insights into disease mechanisms and drug efficacy. This information can be used to design trials that are more targeted and personalized, leading to better patient outcomes. Additionally, data analytics can help identify potential safety issues early in the trial process, allowing researchers to take corrective action before the trial is completed. Overall, data analytics is a critical component of clinical trial optimization, and its use is expected to continue to grow in the pharmaceutical industry.
Real-World Evidence Generation:
Real-world evidence generation is the process of collecting and analyzing data from patients in real-world settings to assess the effectiveness and safety of drugs. This approach is becoming increasingly important in the pharmaceutical manufacturing and biotechnology industries. Data analytics enables the analysis of large amounts of real-world data, providing valuable insights into drug efficacy and safety. This evidence is crucial for regulatory approvals and drug pricing decisions. Real-world evidence generation can also help identify patient subgroups that may benefit from a particular drug, leading to more targeted and personalized treatments. Overall, real-world evidence generation is a critical component of drug development and is expected to continue to grow in importance in the pharmaceutical and biotechnology industries.
Pharmacovigilance and Drug Safety
Pharmacovigilance and drug safety are critical components of the pharmaceutical engineering and life science industry. Data analytics is used to monitor patient data for adverse drug reactions, medication interactions, and other safety concerns. This proactive approach helps identify and address safety issues early on, preventing harm to patients. By analyzing large amounts of data from a variety of sources, including electronic health records, clinical trial data, and social media, researchers can gain insights into drug safety and efficacy. This information can be used to improve drug design and development, as well as inform regulatory decisions. Overall, data analytics in pharmaceutical industry is a powerful tool for improving patient safety and advancing drug development.
Supply Chain Management
Data analytics is crucial for pharmaceutical supply chain optimization, enabling companies to predict demand using sales data, manage inventory levels by identifying fast- and slow-moving drugs, and proactively identify potential disruptions by monitoring suppliers and logistics. This improves efficiency, reduces costs, and ensures drug availability. Specifically, predictive analytics informs inventory planning to avoid overstocking or shortages. Monitoring supplier and logistics data flags upcoming issues before they disrupt supply. By leveraging data analytics across the supply chain, pharma companies can boost productivity, cut waste, and maintain the availability of life-saving medications.
Product Optimization
Data analytics in pharmaceutical manufacturing is transforming production and chemical processes in the industry. By analyzing manufacturing data from sensors and equipment, pharmaceutical companies can identify inefficiencies, optimize yields, and reduce waste. This allows for more agile, intelligent manufacturing. Data analytics also enables predictive maintenance to minimize downtime. For the chemical industry supplying drug compounds, analytics improves chemical process controls for consistent quality and output. Overall, data-driven manufacturing and chemical process optimization boost productivity, asset utilization, and end-product quality in pharmaceuticals. Advanced analytics unlocks major efficiency gains across pharmaceutical and chemical operations.
Patient Adherence and Outcomes
Pharmaceutical business intelligence is increasingly using data analytics to optimize supply chain operations, reduce costs, improve efficiency, and ensure drug availability. One of the ways data analytics is used is to identify patients who are at risk of non-adherence to their medication regimens. This information allows for targeted interventions to improve patient compliance and optimize treatment outcomes. By analyzing large amounts of data, data analytics can help predict demand, manage inventory levels, and identify potential supply chain disruptions. Overall, data analytics in the pharmaceutical industry is a powerful tool for improving various aspects of supply chain management and patient outcomes.
Personalized Medicine
Data analytics in the pharma industry is being used to develop personalized treatment plans based on individual patient data. For example, a patient with a chronic condition such as diabetes may have their blood sugar levels monitored using wearable devices. The data collected from these devices can be analyzed using data analytics tools to identify patterns and insights that can be used to develop personalized treatment plans. Additionally, data analytics can help identify patient subgroups that may benefit from a particular drug, leading to more targeted and personalized treatments.
Pharmacogenomics
Pharmacogenomics is the study of how an individual’s genetic makeup influences their response to drugs. Data analytics is being used in pharmacogenomics to analyze genetic data and predict patient responses to specific drugs. This information allows for personalized prescribing and reduces the risk of adverse drug reactions. By analyzing large amounts of data from various sources, predictive analytics can identify patterns and insights that can inform the development of targeted interventions and programs and help healthcare providers make more informed decisions about patient care. Additionally, the technology can help physicians develop personalized treatment plans for individual patients, which can help to improve outcomes and reduce healthcare costs. The use of bioinformatics and machine learning methods can also aid in the identification of predictive biomarkers and drug targets, leading to more effective and targeted treatments.
Medical Imaging Analysis:
Medical Imaging Analysis harnesses data analytics to scrutinize images, including X-rays, CT scans, and MRIs, revolutionizing diagnostic accuracy and early health issue detection. By leveraging advanced algorithms and machine learning, this technology interprets intricate patterns within medical images, aiding healthcare professionals in identifying abnormalities and potential concerns. The synergy of data analytics and medical imaging not only enhances diagnostic precision but also expedites the identification of health issues at their nascent stages. This proactive approach facilitates timely interventions, improving patient outcomes and reducing the impact of illnesses. The evolving landscape of Medical Imaging Analysis underscores the transformative power of data analytics in healthcare, paving the way for more effective diagnostics and personalized medical interventions.
Types of Data in Pharmaceutical Industry
There are two types of data in the pharma industry – primary and secondary data. Pharmaceutical companies use this data to discover and develop new drugs and treatments, improve the safety and efficacy of existing drugs, conduct clinical trials, optimize manufacturing and supply chain management, market and sell drugs, and make better business decisions.
Primary Data
Clinical trial data: Data collected from human clinical trials, such as patient demographics, medical history, treatment outcomes, and adverse events.
Patient data: Data collected from patients, such as electronic health records (EHRs), medical device data, and patient-reported outcomes (PROs).
Genomics data: Data collected from genetic sequencing, such as single nucleotide polymorphisms (SNPs) and copy number variations (CNVs).
Real-world data (RWD): Data collected from patients outside of clinical trials, such as EHRs, claims data, and social media data.
Secondary data
Literature data: Data from published scientific literature, such as research papers and clinical trial reports.
Sales and marketing data: Data on drug sales, marketing campaigns, and customer preferences.
Regulatory data: Data from regulatory agencies, such as the U.S. Food and Drug Administration (FDA) and the European Medicines Agency (EMA).
Competitive intelligence: Data on competitor products and services.
As the pharmaceutical industry continues to generate and collect more data, it is important for companies to develop robust data management and analytics capabilities. This will enable them to extract insights from their data and make better decisions that improve patient care and business success.
How Does Pfizer Use Data Analytics: Case Study
Pfizer is using data analytics to identify patients with rare diseases. The company has developed a novel machine-learning model that can identify symptoms of rare diseases based on patient data from electronic health records (EHRs). The model was validated with a dataset of over 100,000 patients with rare diseases.
Pfizer is using the model to screen patients for rare diseases in clinical trials. The company is also working to make the model available to clinicians so that they can use it to diagnose rare diseases in their patients.
This is just one example of how Pfizer is using data analytics to improve its drug discovery and development process, clinical trials, manufacturing, and marketing. Pfizer is committed to using data analytics to develop better medicines and to improve the lives of patients.
Machine Learning in Pharmaceutical Industry
ML is being used in the pharmaceutical industry in a variety of ways, including:
Drug discovery and development: ML can be used to identify new drug targets, design and develop new drugs, and predict patient outcomes. For example, ML algorithms can be used to analyze large datasets of genomic data and clinical trial data to identify new genes and proteins that could be potential drug targets. ML can also be used to design new drugs and predict how they will interact with the human body.
Clinical trials: ML can be used to optimize clinical trial design and detect adverse events. For example, ML algorithms can be used to identify the most promising patient populations for clinical trials and to design trials that are more efficient and effective. ML can also be used to monitor clinical trial data for safety signals so that adverse events can be detected early.
Manufacturing: ML can be used to improve manufacturing efficiency and quality. For example, ML algorithms can be used to predict demand for drugs and to optimize the supply chain. ML can also be used to monitor manufacturing processes for quality control.
Marketing: ML can be used to target marketing messages to specific patient populations and to measure the effectiveness of marketing campaigns. For example, ML algorithms can be used to identify patients who are most likely to benefit from drugs and to develop marketing messages that are relevant to their needs. ML can also be used to track the performance of marketing campaigns and to make adjustments as needed.
Big Data Analytics in Pharma: Key Challenges
There are a number of key challenges associated with big data analytics in the pharmaceutical industry, including:
Data silos: Pharmaceutical companies often have data silos, where data is stored in different systems and formats. This can make it difficult to integrate data and get a holistic view of patient care and drug development.
Data quality: Pharmaceutical companies collect a wide variety of data, from clinical trials to real-world data. This data can be messy and incomplete, making it challenging to analyze.
Data privacy and security: Pharmaceutical companies must comply with strict data privacy and security regulations. This can make it difficult to share data with external partners and to use cloud-based analytics solutions.
Lack of skills and expertise: Pharmaceutical companies need to have the skills and expertise to manage and analyze big data. This can be a challenge, as there is a shortage of data scientists and other professionals with the necessary skills.
Conclusion
In conclusion, data analytics has become a critical component of the pharmaceutical industry, transforming the way drugs are discovered, developed, and marketed. The use of data analytics in the pharma industry has enabled the development of personalized treatment plans based on individual patient data, leading to more effective and targeted treatments. It has also helped analyze genetic data to predict patient responses to specific drugs, allowing for personalized prescribing and reducing the risk of adverse drug reactions. Additionally, data analytics has been used to optimize supply chain operations, reduce costs, improve efficiency, and ensure drug availability. The pharma industry has benefited from the rapid growth of technology and constantly increased its adoption of pharma analytics and other digital technologies to become more efficient and protected against future systemic shocks like coronavirus pandemics. Overall, data analytics in the pharma industry is a powerful tool for improving patient outcomes, advancing drug development, and driving business growth.