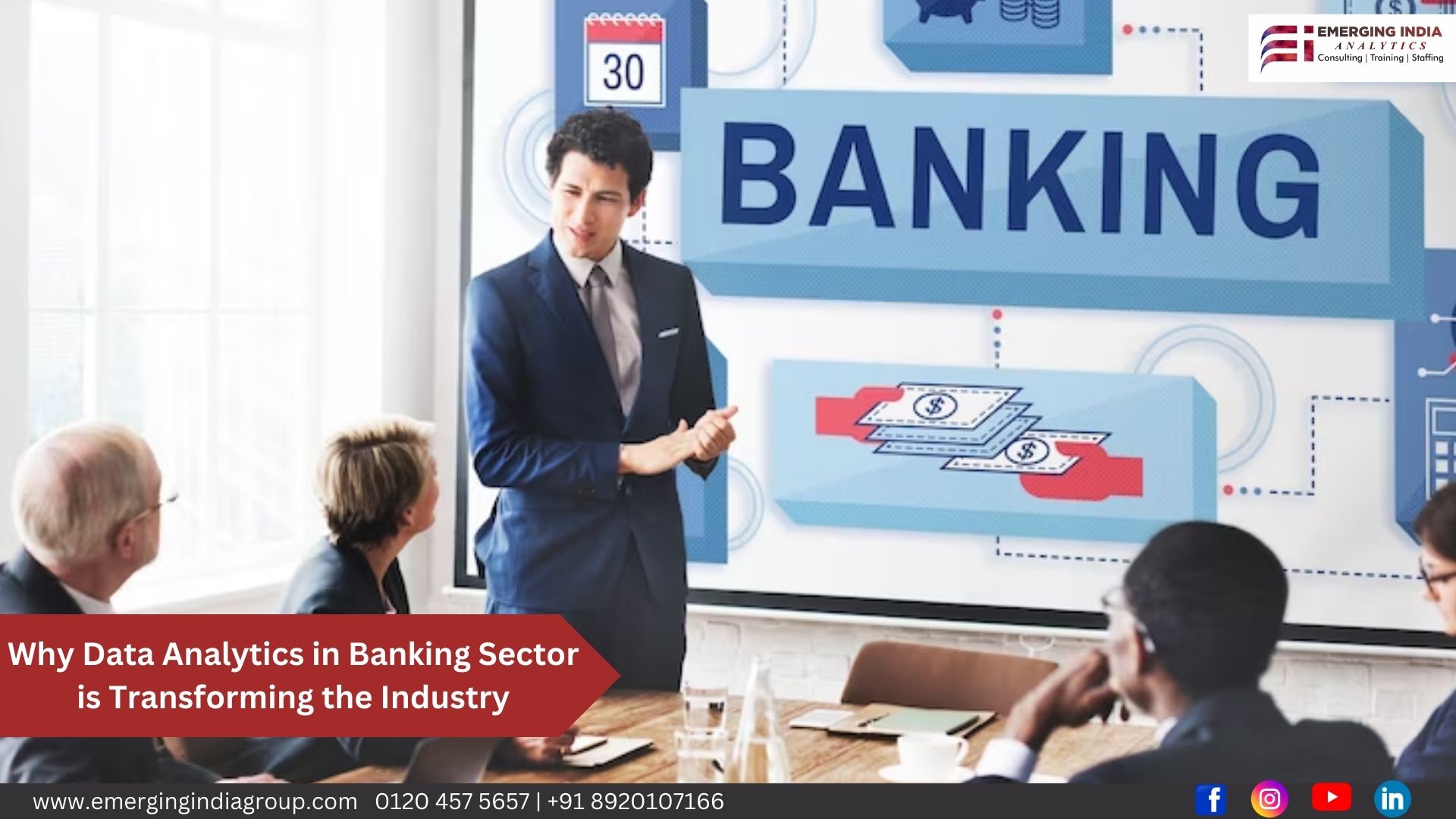
The banking industry is undergoing a major transformation, driven in large part by the adoption of data analytics. Data analytics is the process of collecting, cleaning, and analyzing data to extract meaningful insights.
Banking institutions, both large and small, are harnessing the power of data analysis to make more informed decisions, enhance customer experiences, and drive operational efficiency.
The convergence of data and banking is not a novel concept, but it is the rapid advancements in technology, coupled with the availability of vast amounts of data, that are redefining the way banks operate. Data analytics for banking has become indispensable, revolutionizing core functions such as risk assessment, customer relationship management, fraud detection, and even strategic planning.
In this article, we will examine how data analytics in banking sector is not merely an option but a necessity for financial institutions aiming to stay competitive and relevant in a digital-driven world.
We will also see the profound impact of data analysis in banking, uncover real-world examples of its application, and gain insights into the future of this dynamic relationship between data and finance. Data analytics in banking domain is more than just a trend; it’s a strategic imperative that is reshaping the financial industry landscape.
What is the Role of Data in the Banking Industry?
Data is the lifeblood of the banking industry, and its significance cannot be overstated. Banks are adept at collecting and leveraging data from diverse sources, encompassing customer transactions, financial markets, and insights from social media.
This invaluable reservoir of data serves a multitude of purposes, ranging from enhancing the customer experience, tailoring products and services to individual preferences, safeguarding against fraud, managing risk, to making more informed strategic decisions.
How do banks use data analytics? The utility of data analytics is paramount in extracting meaningful insights from the wealth of data amassed. In practice, banks leverage data analytics in the following ways:
Identify customer needs and preferences: Harnessing data analytics, banks gain deep insights into customer spending patterns, financial objectives, and risk tolerance. These insights empower banks to craft highly personalized products and services, elevating the customer experience to new heights.
Develop predictive models: Data analytics enables the creation of predictive models, forecasting customer behavior with remarkable accuracy. Banks can preemptively address concerns such as customer churn or loan defaults, taking proactive measures to ensure customer retention and loan repayment.
Detect and prevent fraud: In the unceasing battle against fraudulent transactions, data analytics is a formidable ally. Banks employ data analytics to flag irregular transactions that deviate from a customer’s typical spending habits, helping to curb fraudulent activities.
Manage risk: Risk management becomes more precise with data analytics. By scrutinizing data, banks can pinpoint customers at risk of loan defaults or other financial perils, allowing for timely interventions.
Make better business decisions: Data-driven insights extend to all aspects of banking, from product development to marketing campaigns, ensuring that decisions are well-informed and aligned with customer needs.
The application of big data in banking involves the handling of vast and intricate datasets that conventional processing methods struggle to manage. Banks are increasingly embracing big data analytics to extract invaluable insights from their data.
Big data analytics in banking sector is diverse, encompassing the following domains:
Customer segmentation: Big data analytics aids banks in categorizing customers based on their distinct needs and preferences, enabling the development of highly targeted marketing strategies and customized products.
Fraud detection: Big data analytics enhances the detection of fraudulent transactions, uncovering intricate patterns of fraudulent behavior that elude traditional methods.
Risk management: For more comprehensive risk management, big data analytics excels in identifying nuanced risks that conventional methods may overlook.
Product development: Banks employ big data analytics to shape new products and services that closely align with customer expectations. This deep understanding of customer needs facilitates the exploration of new market opportunities. Banks are investing heavily in big data analytics technologies and solutions. For example, JPMorgan Chase has invested over $1 billion in big data analytics.
Big data analytics in banking sector is rapidly gaining prominence, as banks recognize its role in refining the customer experience, mitigating fraud, managing risk, and driving informed decision-making.
Data analysts in banking sector are the linchpin of this data-driven transformation. These professionals play a pivotal role in collecting, cleansing, and analyzing data to extract invaluable insights.
Their expertise aids banks in their pursuit of enhancing the customer experience, preventing fraud, managing risk, and making strategic decisions. For data analysts in the banking sector, a strong command of data analytics tools and techniques, coupled with a deep understanding of the banking industry, is imperative.
Data analytics in banking sector is a game-changer, redefining how banks operate and interact with their customers. Those banks that effectively harness data analytics will be better poised to thrive in an increasingly competitive and dynamic financial landscape.
Do Banks Have a Data Strategy?
Banks indeed have data strategies, although the maturity of these strategies can vary significantly. The development stage of these strategies depends on multiple factors, such as the bank’s size, its industry of operation, and the level of competition it encounters.
Typically, larger banks and those operating in highly competitive industries tend to have more mature data strategies.
A mature data strategy within the banking sector comprises several crucial components. It should encompass a clear vision outlining how data will be leveraged to support the bank’s overarching business goals.
Furthermore, it should include a well-defined roadmap, elucidating the steps and processes that will enable the bank to realize its data vision. This entails a comprehensive plan for data collection, management, and analysis, ensuring that data remains a strategic asset.
Security is paramount, necessitating a plan to protect the data from breaches and unauthorized access. Lastly, the strategy should establish how the bank will harness data to make more informed and profitable decisions.
Business analytics in banking sector plays a pivotal role in enhancing operational efficiency and profitability. Banks employ business analytics to identify areas where cost reductions and process streamlining are possible, ultimately improving the bottom line.
Concurrently, data analytics in banking sector extends to risk analytics, which is instrumental in identifying and managing risks like customers at risk of defaulting on loans. Fraud analytics is a key tool in detecting and preventing fraudulent transactions and identifying anomalies in customer spending patterns.
Additionally, marketing analytics is being used to gain deeper insights into customer behavior and develop more precisely targeted marketing campaigns.
For banks with mature data strategies, the path to success in the future is clearer. Leveraging data for enhanced customer experiences, fraud prevention, risk management, and informed decision-making positions them to attract, retain, and expand their customer base, thus fostering business growth.
What are the Advantages of Data Analytics in Banking Sector?
Data analytics, a process involving data collection, cleaning, and analysis, has become a linchpin for banks and financial institutions in the quest for progress.
Certainly, here are the key points highlighting the benefits of data analytics in banking sector:
Enhanced Customer Experience: Data analytics helps banks understand customer needs and preferences, leading to more personalized products and services. It enables banks to identify at-risk customers and offer tailored solutions to reduce churn.
Personalized Products and Services: Data analytics allows banks to create customized offerings based on individual customer risk tolerance and financial goals.
Fraud Prevention: Banks use data analytics to identify and prevent fraudulent transactions by detecting anomalies in customer spending patterns.
Risk Management: Data analytics helps banks identify customers at risk of loan defaults and take timely corrective actions.
Increased Operational Efficiency: Banks optimize operations by identifying areas for cost reduction and process streamlining using data analytics.
Informed Business Decisions: Data analytics aids in identifying new market opportunities and making strategic decisions regarding product and service expansion or discontinuation.
Regulatory Compliance: Data analytics assists banks in complying with complex regulations, reducing the risk of costly fines.
Innovation: Data analytics drives the development of innovative products and services like mobile banking apps and robo-advisors.
Competitive Advantage: Banks that effectively utilize data analytics gain a competitive edge by better understanding their customers, preventing fraud, making informed decisions, and staying at the forefront of technological innovation.
These points emphasize the significant role of data analytics in banking sector, transforming and improving customer experiences to ensure compliance and fostering innovation.
How is data analytics used in banks?
These are some ways how banks use data analytics to enhance various aspects of their operations, including:
Customer Segmentation: Data analytics in banking sector categorize their customers based on their unique needs and preferences. This information paves the way for the development of precisely tailored marketing campaigns and product offerings.
For instance, a bank may use data analytics to divide its customer base into distinct groups, such as high-net-worth individuals and mass-market customers, each warranting a different approach.
Credit Scoring: Data analytics is instrumental in crafting credit scores for borrowers, a critical tool for assessing lending risk. Higher credit scores denote lower lending risk, and these scores influence loan approval and interest rates.
Banks analyze an array of data points, encompassing payment history, credit utilization, length of credit history, credit mix, and new credit inquiries, to formulate these scores. Various proprietary models factor in these data points to determine creditworthiness.
Fair Practices: While banks employ data analytics for credit scoring, they are bound by laws and regulations to ensure fairness and avoid discrimination. The Equal Credit Opportunity Act (ECOA) prohibits lending discrimination based on specific characteristics.
Banks must meticulously design their models, avoiding data points correlated with protected characteristics. Transparency is key; banks must provide borrowers with access to their credit scores and a clear explanation of the factors contributing to their scores.
Additionally, banks employ data analytics for various purposes, including customer needs identification, fraud detection, risk management, and product development. This multi-faceted approach demonstrates how data analytics plays a pivotal role in reshaping the banking sector.
Banking Account-Based Analytics Programs (BABAPs) further enhance these capabilities by offering a specialized type of data analytics that revolves around customer banking account data. These programs assist banks in identifying customer needs, segmenting their customer base, developing targeted marketing strategies, preventing fraud, and managing risk.
10 Reasons Why Data Analytics in Banking Sector is Changing the Landscape of the Industry
A Better Understanding of Customer Needs and Preferences
Banks leverage data analytics in several ways to gain a comprehensive understanding of their customer’s needs and preferences. By analyzing customer transaction data, banks identify spending patterns, product preferences, and risk tolerance. Additionally, they use customer survey data to gather feedback on products and services.
For instance, customer withdrawal patterns from ATMs could prompt the bank to offer solutions like a mobile banking app or a cashback credit card.
Development of More Targeted Marketing Campaigns
With a clearer understanding of customer needs and preferences, banks can craft more targeted marketing campaigns. They can personalize offers for products and services, catering to individual interests.
For example, identifying customers nearing retirement allows banks to provide information about retirement planning and relevant investment products.
Improved Customer Segmentation
Data analytics improves customer segmentation, allowing banks to group customers with similar needs together. This grouping is instrumental in developing targeted marketing campaigns and customizing products and services for different segments.
An example could involve segmenting customers into high-net-worth individuals and mass-market customers and tailoring products and services for each group.
Identification of Cross-Selling and Up-Selling Opportunities
Using data analytics, banks identify cross-selling and up-selling opportunities, offering complementary products and services to customers based on their current usage.
For instance, customers holding a credit card and a checking account might be offered a higher-interest savings account.
Detection of Early Signs of Customer Churn
Data analytics helps banks detect early signs of customer churn, enabling proactive steps to retain customers. Identifying inactive credit card users prompts the bank to reach out and offer assistance or reminders about card benefits.
Reduction of Customer Acquisition Costs
With a better understanding of target customers, banks optimize marketing campaigns, reducing acquisition costs by precise targeting.
For instance, targeting potential new checking account customers through online advertising or direct mail campaigns.
Improvement of Customer Retention Rates
Understanding the reasons behind customer churn helps banks take corrective actions, potentially addressing root causes.
For example, surveying churned customers to learn why they left and exploring ways to win them back.
Development of New Products and Services
Data analytics helps identify market gaps, leading to the creation of innovative products and services that fulfill customer needs.
For instance, identifying customers interested in sustainable investing might prompt the development of investment products focusing on strong environmental, social, and governance (ESG) records.
Optimization of Pricing Strategies
Understanding customer demand and cost structures allows banks to set competitive and profitable prices for products and services.
For example, determining loan interest rates based on factors like the customer’s credit score and current market rates.
Reduction of Fraud Losses
Analyzing transaction data enables banks to detect fraudulent patterns, protecting customers’ finances.
For example, identifying unusual transactions in terms of devices or locations triggers verification by contacting the customer.
These examples illustrate the transformative impact of data analytics in banking sector. Its role is increasingly crucial, positioning banks to excel in the future by effectively using data analytics.
Big Data Analytics Banking Example
JPMorgan harnesses the power of big data to craft highly personalized marketing campaigns within the dynamic banking and finance sector.
With an extensive repository of customer data at its disposal, including transaction histories, demographics, and credit scores, the bank employs an array of tools and techniques to unearth patterns and trends, enabling it to connect with its clientele more effectively.
Key to their personalization efforts is the use of machine learning, which thrives on historical data to predict future customer behavior.
For instance, machine learning algorithms delve into past marketing campaigns to identify the decisive factors that encourage customer purchases.
Once these influential factors are identified, JPMorgan tailors its marketing campaigns accordingly. This could involve sending customers targeted offers for products and services aligning with their preferences or delivering reminders about impending due dates and significant events.
JPMorgan’s application of big data to personalize marketing campaigns encompasses various facets:
Targeted Email Campaigns: The bank segments its customer base using big data, discerning individual needs and preferences. Subsequently, this insight informs the creation of customized email campaigns.
For instance, customers nearing retirement receive distinct campaigns from those just embarking on their careers.
Personalized Website Recommendations: Visitors to the JPMorgan website encounter tailored recommendations based on their previous browsing behavior and transaction history.
For instance, a customer exploring mortgage information might find mortgage product ads prominently displayed on the homepage.
Social Media Targeting: Big data also guides JPMorgan’s social media ad targeting efforts. It allows the bank to channel ads for student loans to college students and direct retirement planning product ads to those nearing retirement age.
To execute these initiatives successfully, JPMorgan leverages a suite of software tools, including:
Hadoop: This distributed computing framework empowers banks to process and analyze vast volumes of data efficiently.
Spark: An open-source distributed computing framework that provides libraries for machine learning, stream processing, and graph processing.
Hive: A data warehouse infrastructure offering robust data query and analysis services.
HBase: A NoSQL database well-suited for the storage and processing of large data volumes.
Additionally, JPMorgan employs proprietary software tools developed in-house by its IT team to further support big data analytics initiatives.
By embracing big data for personalized marketing, JPMorgan positions itself to deliver precisely timed and relevant messages to its customers. This not only enhances customer engagement but also bolsters conversion rates within the banking and finance sector.
It exemplifies the significant role of big data analytics in banking sector and the far-reaching impact of big data in the financial industry.
What is the Future of Data Analytics in Banking Sector?
Data analytics in banking is rapidly transforming the sector, and its impact is only going to grow in the future. Banks are increasingly using data analytics to improve customer experience, develop new products and services, manage risk, and prevent fraud.
In the future, data analytics is expected to play an even more critical role in the banking sector. Here are some specific trends that we can expect to see:
Increased use of artificial intelligence (AI) and machine learning (ML): AI and ML algorithms are becoming increasingly sophisticated and powerful. Banks will use AI and ML to develop new data analytics solutions that can help them to better understand their customers, make better business decisions, and manage risk more effectively.
Real-time data analytics: Banks are increasingly moving to real-time data analytics. This means that they are able to analyze data as it is being generated, rather than having to wait for it to be processed and stored in a data warehouse. Real-time data analytics gives banks the ability to respond to changes in the market and customer needs more quickly and effectively.
Greater use of cloud computing: Cloud computing provides banks with access to scalable and affordable computing resources. This will make it easier and more affordable for banks to implement and use data analytics solutions.
More open and collaborative data sharing: Banks are increasingly sharing data with each other and with third-party partners. This is opening up new opportunities for collaboration and innovation. For example, banks can share data with each other to develop new fraud prevention systems.
Overall, the future of data analytics in banking sector is very bright. Banks that are able to effectively use data analytics will be well-positioned to succeed in the future.
In addition to the above trends, we can also expect to see data analytics being used to develop new business models and disrupt existing ones. For example, banks may use data analytics to develop new ways to lend money to businesses and consumers.
Data analytics is also expected to play an important role in the development of new financial products and services. For example, banks may use data analytics to develop new investment products that are tailored to the individual needs of their customers.
Overall, the future of data analytics in banking and finance is full of possibilities. Banks that are able to embrace data analytics and use it to their advantage will be well-positioned to succeed in the years to come.
Conclusion
In conclusion, the integration of data analytics in banking and financial services has emerged as a transformative force within the industry. The banking sector’s adoption of data analytics has paved the way for enhanced customer experiences, precision-targeted marketing, risk mitigation, and strategic decision-making. This evolution not only underscores the pivotal role of data analytics in banking sector but also highlights the sector’s commitment to staying ahead in an increasingly data-driven landscape. As data analytics continues to shape the future of banking, institutions embracing this paradigm shift are better positioned to thrive in an ever-evolving and competitive environment.