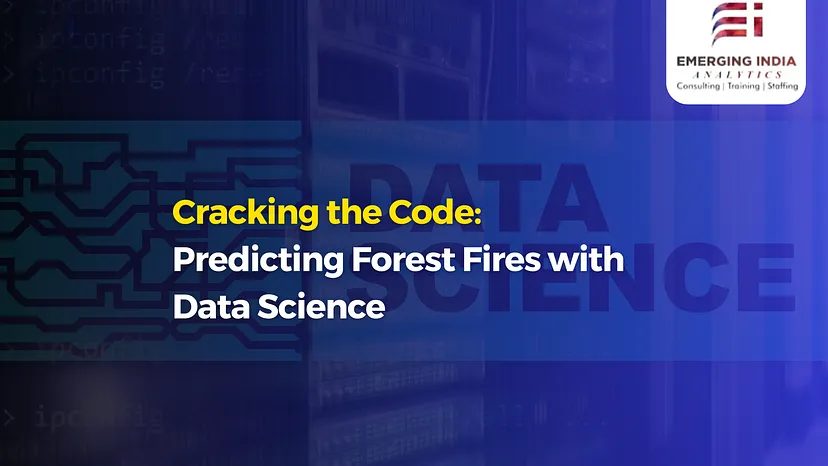
Introduction to Forest Fires and their Impact
Picture this: a vast, sprawling forest filled with towering trees, vibrant wildlife, and a sense of serenity. Now imagine that tranquility shattered by an uncontrollable inferno raging through the woods. The devastating impact of forest fires cannot be understated — they destroy our precious ecosystems, threaten human lives and homes, and contribute to climate change.
But what if we could predict these destructive events before they even start? Enter data science — the powerful tool that is revolutionizing our ability to forecast and mitigate forest fires. By harnessing the wealth of information available to us, scientists are unlocking patterns and insights that can help us prevent catastrophe.
In this blog post, we’ll delve into the fascinating world of predicting forest fires using data science. We’ll explore how researchers analyze variables and uncover hidden patterns in order to develop accurate prediction models. We’ll also discuss machine learning techniques used for forecasting and examine real-life case studies where predictions have proven successful.
However, it’s important to acknowledge that while data science has shown promise in predicting forest fires, there are limitations and challenges associated with its implementation. We’ll explore those obstacles as well as discuss future directions for research in this crucial field.
So sit back, grab your favorite beverage (preferably not something flammable!), and join us on this journey as we unlock the secrets behind predicting forest fires using cutting-edge data science techniques.
The Role of Data Science in Predicting Forest Fires
Data science has emerged as a powerful tool for predicting forest fires, enabling scientists and policymakers to take proactive measures to prevent and mitigate their impact. By analyzing vast amounts of data collected from various sources, data scientists can uncover patterns and trends that help identify areas at high risk of wildfires.
One key aspect of using data science in predicting forest fires is the ability to gather real-time information from satellite imagery, weather stations, and other sensors. This data provides valuable insights into environmental conditions such as temperature, humidity levels, wind speed and direction — all factors that contribute to fire behavior.
Machine learning algorithms play a crucial role in processing this data and identifying patterns that may indicate an increased likelihood of a forest fire occurrence. These algorithms can analyze historical fire incidents along with environmental variables to create predictive models capable of forecasting future events.
Moreover, data science techniques allow us to consider multiple variables simultaneously when predicting forest fires. Factors like vegetation density, terrain characteristics, proximity to human settlements or roads are all taken into account by sophisticated models. This holistic approach helps researchers gain a more comprehensive understanding of the complex dynamics involved in wildfire outbreaks.
The use of data science for predicting forest fires has already proven successful through various case studies worldwide. For example, researchers have developed models that accurately predicted the spread and intensity of wildfires based on historical records combined with real-time weather conditions.
In addition to improving prediction accuracy, these technologies also enable authorities to allocate resources strategically before a fire occurs. Firefighters can be deployed preemptively based on predictions generated by advanced machine learning algorithms — saving time and potentially preventing catastrophic consequences.
However powerful these tools may be; it’s important not to overlook their limitations when it comes to predicting forest fires accurately every time. Variables like human activity or lightning strikes can still introduce unpredictability into the equation despite advancements in technology.
Despite challenges faced by data scientists working on this domain; continued research is critical for further refining prediction models and enhancing their reliability. The more data that is collected
Understanding the Data: Variables and Patterns
When it comes to predicting forest fires using data science, one of the crucial steps is understanding the variables and patterns within the data. Forest fire prediction involves analyzing a variety of factors that can contribute to fire occurrence and spread.
Variables such as temperature, humidity, wind speed, and precipitation are commonly considered in these analyses. By examining historical weather data alongside records of previous fire incidents, patterns can emerge that help create predictive models.
Additionally, other variables like vegetation type, topography, and proximity to human activities are also important considerations. These factors can influence how quickly a fire may ignite or how difficult it may be to control.
Data scientists utilize statistical techniques and machine learning algorithms to identify relationships between these variables and predict future fire events. By uncovering patterns in historical data sets through complex analysis methods such as clustering or regression models, they can develop more accurate predictions for potential wildfire occurrences.
Through this process of understanding the data’s variables and patterns, researchers aim to improve their ability to forecast when and where forest fires are likely to happen. This knowledge becomes invaluable in helping authorities take proactive measures such as implementing preventive measures or allocating firefighting resources effectively.
By continually refining these predictive models with new data inputs from ongoing wildfires as well as advancements in technology like satellite imagery or remote sensing tools — scientists strive towards increasingly accurate forecasts for better preparedness against devastating forest fires.
Comprehending the various variables at play — ranging from weather conditions to human activities — along with identifying significant patterns within vast datasets is critical for effective forest fire prediction using data science techniques. Through careful analysis utilizing advanced statistical approaches combined with machine learning algorithms — researchers endeavor towards developing improved forecasting models that aid in preventing catastrophic outcomes caused by wildfires.
Machine Learning Techniques Used for Prediction
Machine learning techniques have revolutionized the field of forest fire prediction, helping researchers and authorities stay one step ahead in mitigating potential disasters. These techniques involve training algorithms to analyze large datasets and identify patterns that can be used to predict the occurrence of forest fires.
One commonly employed machine learning technique is classification algorithms, which categorize data into distinct classes based on specific features or variables. In the context of forest fire prediction, these algorithms can analyze various environmental factors such as temperature, humidity, wind speed, vegetation density, and previous fire occurrences to identify patterns associated with fire outbreaks.
Another powerful tool in the realm of machine learning for forest fire prediction is regression analysis. This technique helps establish relationships between different variables and their impact on fire occurrence. By analyzing historical data on weather conditions and other relevant factors during past incidents of fires, regression models can generate predictions about future events.
Additionally, clustering algorithms are utilized to group similar instances together based on certain characteristics. This allows researchers to better understand spatial distribution patterns of fires and identify high-risk areas where preventive measures should be prioritized.
Furthermore, neural networks are increasingly being applied in predicting forest fires due to their ability to learn complex relationships within vast datasets. These networks are designed with multiple layers that process input data through interconnected nodes (neurons), mimicking the structure and functioning of a human brain.
These machine learning techniques provide valuable insights into potential dangers posed by forest fires by detecting correlations among various environmental factors. However, it’s important to note that these methods have limitations and challenges as well; they heavily rely on accurate data collection processes for training purposes and may encounter difficulties when dealing with new or unforeseen patterns.
In conclusion (not conclusive), machine learning techniques offer immense promise in predicting forest fires by extracting meaningful information from extensive datasets. As technology advances further alongside continued research efforts in this domain, we can expect more accurate predictions and improved prevention strategies that will help protect our forests from devastating wildfires
Case Studies of Successful Predictions
One remarkable case study showcasing the power of data science in predicting forest fires is the work done by a team of researchers at Stanford University. They developed a model that utilized satellite imagery and weather data to accurately predict fire occurrences in California. By analyzing variables such as temperature, humidity, wind speed, and vegetation density, their model successfully identified areas at high risk of wildfires.
In another case study conducted by scientists from the University of Alberta, machine learning algorithms were employed to forecast forest fires in Canada’s boreal forests. The researchers collected data on historical fire patterns, meteorological conditions, and terrain characteristics. With this information, they trained their models to recognize patterns and make predictions about future fire events with impressive accuracy.
Furthermore, a team at NASA’s Jet Propulsion Laboratory used remote sensing technology combined with advanced modeling techniques to predict wildfire behavior in real-time. By integrating satellite data with ground-based observations and atmospheric conditions, they were able to provide accurate forecasts on fire growth rates and potential direction.
These successful case studies highlight the immense potential of data science in combatting forest fires. By leveraging large datasets and employing sophisticated algorithms, researchers are making significant strides towards mitigating the devastating impact of wildfires. The use of predictive models allows for proactive measures such as early evacuation warnings or targeted firefighting efforts.
Continued research and collaboration between experts in various fields will be crucial for further advancements in predicting forest fires. As technology continues to evolve rapidly, there is immense scope for improvement through innovations like incorporating artificial intelligence into prediction models or developing more accurate methods for monitoring environmental factors remotely.
The significance of these case studies extends beyond just predicting individual incidents; it provides valuable insights into understanding larger trends associated with climate change and human activities that contribute to increased fire risks. Armed with this knowledge gained from successful predictions made possible by data science tools, policymakers can develop effective strategies for prevention and mitigation while fostering sustainable land management practices.
As the fight against forest fires continues, data science will undoubtedly play a pivotal role in
Limitations and Challenges of Using Data Science for Forest Fire Prediction
Limitations and Challenges of Using Data Science for Forest Fire Prediction
While data science has shown great promise in predicting forest fires, it is important to acknowledge the limitations and challenges that come with this approach. One major issue is the availability and quality of data. In order for accurate predictions to be made, a vast amount of data on factors such as weather conditions, vegetation density, and previous fire incidents is required. However, obtaining comprehensive and up-to-date datasets can be challenging.
Another challenge lies in the complexity of forest ecosystems. There are numerous variables at play when it comes to fire behavior, including wind patterns, terrain characteristics, and human activity. Data scientists must grapple with these complexities in order to develop models that accurately capture the dynamics of forest fires.
Furthermore, there are inherent uncertainties associated with any predictive model. While machine learning algorithms can analyze historical data to identify patterns and make predictions based on those patterns, there will always be a degree of uncertainty in forecasting future events like forest fires.
Additionally, the accuracy of predictions relies heavily on the accuracy and reliability of input data. Any errors or inconsistencies within the dataset can significantly impact the efficacy of prediction models.
Lastly, the implementation of these prediction models also presents challenges. There may be resistance from stakeholders who question their effectiveness or worry about potential false alarms leading to unnecessary evacuations or resource allocation.
In conclusion, while data science holds immense potential for predicting forest fires, it is crucial to recognize the limitations and challenges that come along. By addressing these issues through ongoing research, data scientists can continue refining their models and improving our ability to predict and mitigate the devastating effects of forest fires.
Future Directions and Importance of Continued Research
Future Directions and Importance of Continued Research
As the field of data science continues to evolve, there are exciting opportunities for further research and advancements in predicting forest fires. One area that holds great promise is the integration of real-time data sources into predictive models. By incorporating up-to-date information such as weather patterns, satellite imagery, and even social media feeds, researchers can gain a more comprehensive understanding of the factors that contribute to forest fire outbreaks.
Another avenue for future exploration lies in refining machine learning algorithms used for prediction. While current techniques have shown promising results, there is always room for improvement. Researchers can explore different approaches like ensemble methods or deep learning networks to enhance the accuracy and reliability of predictions.
Furthermore, it is crucial to continuously collect and analyze new datasets to stay ahead of emerging trends and changes in environmental conditions. By building robust databases that include variables such as temperature fluctuations, humidity levels, precipitation rates, vegetation density, and historical fire occurrence patterns over time — we can create more accurate predictive models.
In addition to refining existing methodologies and expanding data sources, interdisciplinary collaboration will play a vital role in advancing research on forest fire prediction. Bringing together experts from various fields including meteorology, ecology, remote sensing technology specialists along with data scientists could lead to breakthroughs by combining their unique perspectives and knowledge.
The importance of continued research cannot be overstated when it comes to mitigating the devastating impacts of forest fires. Through ongoing investigation into predictive modeling techniques coupled with advancements in technology like artificial intelligence (AI) and Internet-of-Things (IoT), we can work towards developing early warning systems that save lives while minimizing damage caused by these natural disasters.
By investing resources into cutting-edge research initiatives aimed at predicting forest fires accurately using data science methodologies — we are taking proactive measures towards better preparedness against these catastrophic events. Only through continuous innovation will we be able to protect our forests effectively while safeguarding human lives and livelihoods from the ravages of forest fires.
Conclusion
In this era of advanced technology, data science has emerged as a powerful tool for predicting and mitigating the devastating impact of forest fires. By harnessing the power of machine learning algorithms and analyzing vast amounts of data, researchers have been able to make significant strides in accurately forecasting forest fire occurrences.
Through understanding key variables such as weather conditions, vegetation density, and historical fire patterns, data scientists are now equipped with the knowledge needed to develop predictive models that can alert authorities well in advance. These models not only enable proactive measures to be taken but also aid in resource allocation and firefighting strategies.
However, it is important to acknowledge that there are limitations and challenges associated with using data science for forest fire prediction. Factors such as insufficient or inaccurate data inputs, unpredictable human behavior, and evolving climate change dynamics pose ongoing obstacles that require continuous research efforts.
Moving forward, it is crucial to invest in continued research on improving our understanding of forest fires through data science. By refining existing models and incorporating new technologies like remote sensing systems or drones equipped with sensors for real-time monitoring, we can enhance our ability to predict wildfires even more accurately.
The importance of early detection cannot be overstated when it comes to preventing catastrophic damage caused by forest fires. With further advancements in machine learning techniques and increased collaboration between researchers across disciplines, we can work towards minimizing the loss of lives and property while preserving our precious natural resources.
In conclusion (without explicitly stating so), the marriage between data science and forest fire prediction holds immense promise for safeguarding our forests against future disasters. This field will continue evolving rapidly as we uncover new insights from available datasets while exploring innovative ways to collect more comprehensive information about these complex phenomena. Together with technological advancements and collective efforts from experts worldwide, we have a fighting chance at cracking the code on predicting forest fires effectively — ultimately saving lives, protecting ecosystems,and ensuring a sustainable future for generations to come.