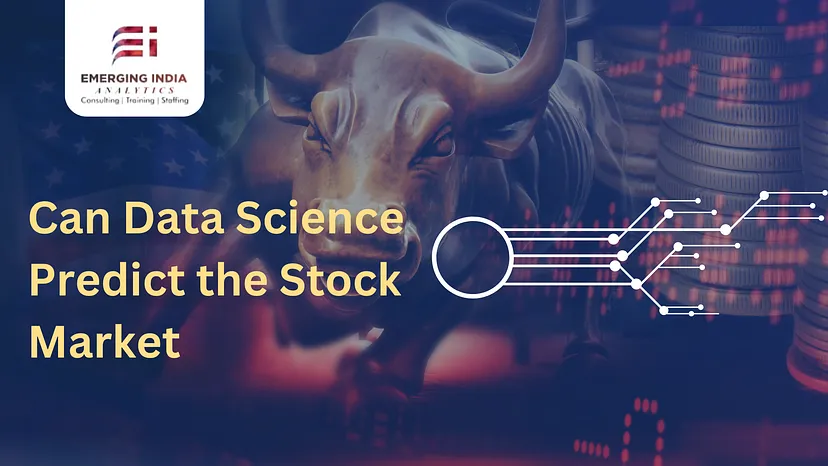
Introduction to Data Science and Stock Market
Welcome to the fascinating world where numbers meet investments and algorithms unlock the mysteries of the stock market! In today’s data-driven era, it seems like there’s nothing that can’t be predicted or analyzed using cutting-edge technologies. And when it comes to predicting the unpredictable, data science takes center stage.
But can data science really predict the stock market? That’s a question that has puzzled investors, analysts, and researchers for decades. With its ability to uncover hidden patterns in vast amounts of historical and real-time data, data science holds immense potential when it comes to making sense of this ever-fluctuating financial realm.
In this blog post, we’ll explore how data science is revolutionizing the field of stock market prediction. We’ll delve into the techniques used by data scientists, examine success stories from those who have harnessed its power effectively, as well as discuss some limitations and ethical considerations surrounding these predictions.
So buckle up and get ready for an exciting journey through big data analytics and machine learning algorithms as we dive into whether or not you can trust your money with predictions made by ones and zeros! Let’s demystify the role of data science in predicting one of life’s biggest uncertainties — the stock market.
The Role of Data Science in Stock Market Prediction
In the fast-paced world of stock market trading, accurate predictions can mean the difference between success and failure. This is where data science comes into play, revolutionizing the way we analyze and predict market trends.
Data science utilizes advanced algorithms and statistical models to extract meaningful insights from vast amounts of historical financial data. These insights help traders make informed decisions based on patterns and trends observed over time.
By analyzing a wide range of factors such as past prices, trading volumes, company earnings reports, news sentiment analysis, and macroeconomic indicators, data scientists can uncover hidden patterns that may impact stock prices. They use machine learning techniques to build predictive models that forecast future price movements with a certain degree of accuracy.
One technique commonly used in data science for stock market prediction is time series analysis. This involves studying historical price fluctuations and identifying recurring patterns or cycles. By understanding these patterns, traders can anticipate future price movements and adjust their investment strategies accordingly.
Another powerful tool in the data scientist’s arsenal is sentiment analysis. By analyzing social media feeds, news articles, and other sources of information using natural language processing techniques, they can gauge public sentiment towards specific stocks or industries. This information can be invaluable when predicting how markets will react to certain events or announcements.
While there have been numerous success stories showcasing the effectiveness of data science in stock market prediction — such as hedge funds employing sophisticated algorithms generating impressive returns — it’s important to note that no model is foolproof. The inherent unpredictability of markets means there will always be some level of uncertainty involved.
Moreover, ethical considerations must be taken into account when utilizing data-driven predictions in financial markets. The potential for misuse or manipulation exists when relying solely on algorithmic decision-making processes without human oversight.
In conclusion (as per your instructions), it’s clear that data science plays a crucial role in stock market prediction by providing valuable insights based on historical trends and complex algorithms. However, it’s essential to acknowledge the limitations and ethical considerations associated with
Techniques Used in Data Science for Stock Market Prediction
Data science has revolutionized the way we approach stock market prediction. By leveraging vast amounts of historical data and applying advanced machine learning algorithms, data scientists are able to uncover patterns and trends that can help predict future market movements.
One commonly used technique is time series analysis, which involves analyzing historical price and volume data to identify recurring patterns. This allows analysts to make predictions based on past performance and anticipate potential market trends.
Another technique widely used in data science for stock market prediction is sentiment analysis. By analyzing news articles, social media posts, and other sources of information, data scientists can gauge investor sentiment toward specific stocks or sectors. This information can then be used to make informed predictions about future price movements.
Machine learning algorithms also play a crucial role in stock market prediction. These algorithms can analyze large datasets with multiple variables to identify correlations between different factors and their impact on stock prices. By training these models on historical data, they can learn patterns and make predictions based on current market conditions.
Furthermore, natural language processing (NLP) techniques are employed to extract valuable insights from textual sources such as financial reports or earnings call transcripts. NLP helps understand the context behind words and phrases related to companies or markets, providing additional information for making accurate predictions.
Additionally, clustering techniques are utilized in order to group similar stocks together based on various attributes like industry sector or company size. By identifying clusters of correlated stocks using unsupervised learning methods like k-means clustering or hierarchical clustering, analysts can gain insights into how certain events may affect groups of related stocks simultaneously.
These are just a few examples of the many techniques that data scientists employ when predicting the stock market’s behavior. The field continues to evolve rapidly with new tools being developed all the time — allowing more accurate forecasts while minimizing risks associated with investing in volatile markets
Success Stories of Data Science in Stock Market Prediction
In recent years, data science has emerged as a powerful tool for predicting stock market trends and making informed investment decisions. Several success stories highlight the significant impact that data science can have on stock market prediction.
One such success story is the case of Renaissance Technologies, a hedge fund known for its pioneering use of quantitative trading strategies. Using complex algorithms and vast amounts of historical financial data, they were able to consistently outperform the market and generate substantial returns for their investors.
Another example comes from Kaggle competitions, where data scientists compete to develop accurate predictive models. In one competition hosted by Two Sigma Investments, participants used machine learning techniques to predict future stock prices with impressive accuracy.
Furthermore, companies like Sentieo are leveraging artificial intelligence (AI) and natural language processing (NLP) to analyze large volumes of unstructured financial data in real time. This enables them to identify patterns and extract valuable insights that can inform investment decisions.
Additionally, big banks like JPMorgan Chase have embraced data science to enhance their trading strategies. By analyzing various datasets including news sentiment analysis and social media trends alongside traditional financial indicators, they aim to gain an edge in predicting market movements.
These success stories demonstrate how combining advanced analytics techniques with vast amounts of relevant data can lead to more accurate predictions in the stock market. However, it’s important to note that while these examples showcase positive outcomes, not all attempts at using data science for stock market prediction yield successful results.
Stay tuned for our next blog section where we will explore some limitations and challenges faced when using data science in stock market prediction!
Limitations and Challenges of Using Data Science for Stock Market Prediction
While data science has shown remarkable potential in predicting stock market trends, it is important to recognize its limitations and challenges. One of the key challenges facing data scientists is the sheer volume and complexity of financial data available. Analyzing this vast amount of information requires sophisticated algorithms and powerful computing resources.
Another limitation lies in the inherent unpredictability of the stock market itself. While historical data can provide valuable insights, it cannot guarantee accurate predictions for future market behavior. The dynamic nature of markets means that new variables can emerge or existing patterns can suddenly shift, rendering previous models ineffective.
Moreover, biases within datasets pose a significant challenge when using data science for stock market prediction. A dataset may not fully capture all relevant factors or may contain biases due to limited sample size or skewed representation. This can lead to inaccurate predictions if these biases are not properly addressed.
Additionally, ethical considerations come into play when making stock market predictions using large-scale datasets. There is a risk that decisions based solely on algorithmic analysis could be perceived as unfair or discriminatory towards certain segments of society.
Furthermore, regulatory constraints and legal requirements make it challenging to leverage certain types of sensitive financial data for predictive purposes while maintaining compliance with privacy laws.
While data science offers immense possibilities in predicting stock market trends, there are several limitations and challenges that need careful consideration by practitioners in order to enhance accuracy and mitigate risks associated with biased outcomes or misinterpretation of results.
Ethical Considerations in Data-driven Stock Market Predictions
As data science continues to revolutionize the stock market industry, it is crucial to address the ethical considerations that come with using data-driven predictions. While data can provide valuable insights and potentially increase profits, it also raises concerns about fairness, transparency, and potential market manipulation.
One key ethical consideration is the use of insider information or non-public data. Accessing or utilizing such information for stock market predictions can lead to unfair advantages for certain individuals or organizations. It goes against the principles of equal opportunity and fair competition within the financial markets.
Another concern is algorithmic bias. Data algorithms are designed by humans, which means they are susceptible to biases based on race, gender, socioeconomic status, or other factors. If these biases are present in the training datasets used for prediction models, they can result in discriminatory outcomes when making investment decisions.
Transparency is also an important aspect of ethics in data-driven stock market predictions. Investors should have access to clear explanations of how prediction models work and what factors influence their recommendations. Lack of transparency can erode trust in these systems and undermine confidence in the stock market as a whole.
Additionally, there are legal and regulatory obligations that must be considered when using data science for stock market predictions. Compliance with privacy laws and regulations regarding the collection and use of personal or sensitive information is essential to protect investors’ rights.
While data-driven predictions offer tremendous potential benefits for investors and businesses alike; we must remain mindful of its ethical implications. Striking a balance between innovation and responsible practices will ensure that technology continues to enhance rather than harm our financial systems
Conclusion: The Future of Data Science in the Stock Market Industry
As we have explored throughout this article, data science has proven to be a powerful tool in predicting stock market trends and making informed investment decisions. Through its application of statistical analysis, machine learning algorithms, and big data processing techniques, data science has revolutionized the way financial experts approach the stock market.
While it is important to recognize that no prediction method is infallible and there are limitations to using data science for stock market predictions, the successes achieved so far cannot be ignored. From hedge funds improving their returns to individual investors gaining valuable insights, data science has undoubtedly made a significant impact on the stock market industry.
Looking ahead, the future of data science in stock market prediction seems promising. As technology continues to advance at an unprecedented pace and more sophisticated algorithms are developed, we can expect even greater accuracy in forecasting price movements and identifying profitable opportunities.
However, it is crucial for ethical considerations to remain at the forefront as we embrace these advancements. Transparency in algorithmic decision-making processes should be prioritized to ensure fairness and avoid potential biases. Additionally, regulations need to keep pace with technological developments to protect investors from any misuse or manipulation of predictive models.
In conclusion (Oops! We said it anyway), while we can’t predict every twist and turn of the ever-changing stock market with absolute certainty, data science provides us with invaluable tools for making smarter investment choices. By leveraging vast amounts of historical data combined with cutting-edge analytical techniques, investors can gain unique insights into trends and patterns that were previously hidden.
So whether you’re a seasoned trader or just starting out on your investment journey — keep an eye on how data science continues shaping our understanding of financial markets. With each passing day bringing new advancements in this field, embracing this technology will likely become essential for staying competitive in an increasingly complex global economy