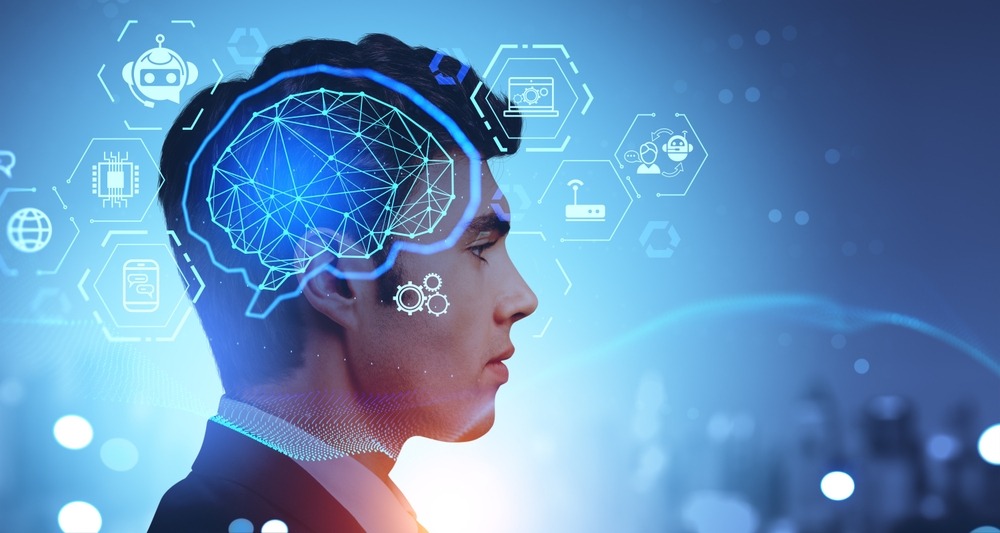
Are you ready to embark on a thrilling journey into the world of artificial intelligence and machine learning? Prepare to be amazed as we delve into the revolutionary concept of synthetic data and how it is breaking barriers, unlocking new horizons for AI. In this blog post, we will uncover the transformative power of synthetic data in accelerating machine learning algorithms and propelling them towards unprecedented heights. Get ready to witness the future unfold before your eyes as we explore how AI is reshaping our understanding of what’s possible in technology.
Introduction to Synthetic Data and AI
Synthetic data is data that has been generated by a computer. It is often used to train machine learning models when real data is not available or is too expensive to obtain.
AI is transforming machine learning by making it possible to generate realistic synthetic data. This synthetic data can be used to train machine learning models to achieve high accuracy without the need for large amounts of training data. Additionally, AI can be used to improve the quality of synthetic data and make it more realistic.
Benefits of Using Synthetic Data in Machine Learning Projects
As machine learning becomes more prevalent, the need for high-quality training data increases. However, acquiring this data can be difficult and costly. Synthetic data can provide a solution to this problem.
Synthetic data is computer-generated data that mimics real-world data. It can be used to train machine learning models in a variety of ways. For example, synthetic data can be used to create realistic simulations for model training. Additionally, synthetic data can be generated at scale, which is often not possible with real-world data.
There are many benefits to using synthetic data in machine learning projects. First, it can help reduce the cost and time needed to acquire training data. Second, it can improve model performance by providing more realistic and varied training data. Third, it can help protect sensitive information by allowing for the creation of privacy-preserving synthetic datasets. It can facilitate the development of new machine learning methods by providing a way to generate large amounts of diverse training data.
Challenges Faced with Generating Synthetic Data
The biggest challenge with generating synthetic data is ensuring that it is realistic enough to be useful for training machine learning models. If the data is too far from reality, the model will not learn anything useful from it. There are a number of ways to generate synthetic data, but all of them have some trade-offs that need to be considered.
One popular method is to use Generative Adversarial Networks (GANs). GANs are able to generate very realistic data, but they are also very computationally expensive and can be difficult to train. Another approach is to use a Variational Autoencoder (VAE), which is less computationally demanding but can sometimes produce less realistic results.
Ultimately, the best way to generate synthetic data will depend on the specific application and what kind of trade-offs are acceptable. It is important to experiment with different methods and see what works best in each case.
Comparing Real and Synthetic Data Quality
There are many ways to compare real and synthetic data quality. One way is to consider the overall accuracy of the data. Another way is to compare the error rates for specific types of data. One can also compare the performance of machine learning models trained on real and synthetic data.
In terms of accuracy, synthetic data is often more accurate than real data. This is because synthetic data can be generated with perfect knowledge of the underlying distribution. Real data, on the other hand, is often noisy and contains errors.
In terms of error rates, synthetic data often has lower error rates than real data. This is because synthetic data can be generated with perfect knowledge of the underlying distribution. Real data, on the other hand, is often noisy and contains errors.
In terms of model performance, machine learning models trained on synthetic data often perform better than those trained on real data. This is because synthetic data can be generated with perfect knowledge of the underlying distribution. Real data, on the other hand, is often noisy and contains errors.
Examples of How Synthetic Data is Used in Machine Learning Projects
As machine learning becomes more ubiquitous, so too does the need for high-quality training data. However, acquiring this data can be difficult and expensive. This is where synthetic data comes in.
Synthetic data is artificially generated data that can be used to train machine learning models. It has many advantages over real-world data, including being more affordable and easier to obtain. Additionally, synthetic data can be generated to match specific desired properties, making it ideal for training machine learning models.
There are a variety of ways that synthetic data can be used in machine learning projects. One common use case is to generate realistic datasets for testing and development purposes. This allows developers to test their algorithms and models on data that closely resembles what they will encounter in the real world.
Another way that synthetic data can be used is to create new training examples from existing ones. This can be especially helpful when there is limited real-world data available. By using synthetic data, it’s possible to expand the training dataset without having to collect new real-world examples.
Synthetic data can also be used to augment existing datasets. This involves adding additional artificial samples to a dataset in order to improve the performance of machine learning models. Data augmentation is often used when there is a need for more diverse training data, such as in the case of image classification tasks.
Synthetic data provides a powerful tool for machine learning practitioners. By leveraging artificial data, it
The Future of Artificial Intelligence and Machine Learning with Synthetic Data
The future of artificial intelligence (AI) and machine learning looks very bright, particularly when it comes to the use of synthetic data. Synthetic data is a powerful tool that can be used to train machine learning models more effectively and efficiently. In addition, synthetic data can be used to create more realistic simulations for testing purposes. As the use of synthetic data becomes more widespread, it is likely that AI and machine learning will become even more transformative technologies.
Conclusion
Synthetic data has revolutionized machine learning, providing a new way to break barriers and increase the accuracy of AI. By leveraging synthetic data generated via generative algorithms, organizations can gain access to an unlimited supply of training data that is tailored specifically for their use cases and easily updated as needed. This enables them to test out new models without needing large amounts of real-world data or having to worry about privacy concerns. With its ability to reduce costs and improve efficiency, synthetic data is proving to be a game changer in the world of AI and ML.