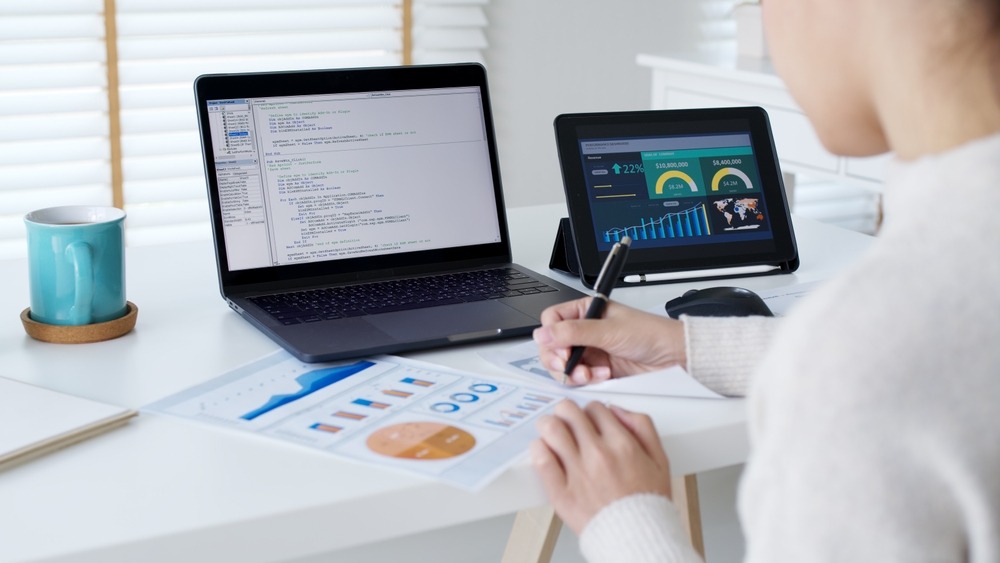
Big data has been a game-changer in the field of big data in data science, offering endless possibilities for organizations to gain valuable insights into their operations and make data-driven decisions.
With the exponential growth of data in recent years, big data has become an essential aspect of data science, presenting both challenges and opportunities for businesses.
In this blog post, we will discuss some of the challenges, opportunities, and best practices that organizations can adopt to leverage big data effectively in data science.
Challenges of Big Data in Data Science
One of the main challenges of big data in data science is managing the sheer volume of data. With data coming from various sources and in various formats, it can be challenging to store, process, and analyse large datasets.
This requires specialized tools and infrastructure, such as Hadoop, Spark, and NoSQL databases, to manage and analyse big data.
Another challenge is ensuring the quality of the data. Big data can be messy, with incomplete, inconsistent, and inaccurate data.
It is essential to ensure data quality by identifying and addressing data quality issues, such as data duplication, missing data, and inconsistencies in data formats.
Privacy and security are also significant challenges in big data. With data coming from various sources, it is challenging to ensure data privacy and security.
This requires implementing data encryption, access controls, and other security measures to protect data from unauthorized access and use.
Opportunities of Big Data in Data Science
Despite the challenges, big data offers significant opportunities for organizations to gain valuable insights into their operations and make data-driven decisions.
With big data, organizations can identify patterns, trends, and anomalies in their data, enabling them to make informed decisions and improve their operations.
One of the significant opportunities of big data is the ability to personalize products and services. By analysing customer data, organizations can gain insights into customer preferences and behaviour, allowing them to tailor their products and services to meet the needs of individual customers.
Big data also enables organizations to improve their operational efficiency.
By analysing operational data, organizations can identify inefficiencies and bottlenecks, enabling them to optimize their operations and reduce costs.
Best Practices for Leveraging Big Data in Data Science
To leverage big data effectively in data science, organizations should adopt best practices, such as:
- Defining clear objectives: Organizations should define clear objectives for their big data initiatives, such as improving customer experience or optimizing operations.
- Collecting relevant data: Organizations should collect relevant data that aligns with their objectives. This requires identifying data sources that are relevant to the organization’s objectives.
- Ensuring data quality: Organizations should ensure data quality by identifying and addressing data quality issues, such as data duplication, missing data, and inconsistencies in data formats.
- Using specialized tools and infrastructure: Organizations should use specialized tools and infrastructure, such as Hadoop, Spark, and NoSQL databases, to manage and analyse big data.
- Implementing data security measures: Organizations should implement data encryption, access controls, and other security measures to protect data from unauthorized access and use.
- Leveraging machine learning and AI: Organizations should leverage machine learning and AI to analyse big data and gain valuable insights into their operations.
Conclusion
Big data presents both challenges and opportunities for organizations in data science. To leverage big data effectively, organizations should adopt best practices such as defining clear objectives, collecting relevant data, ensuring data quality, using specialized tools and infrastructure, implementing data security measures, and leveraging machine learning and AI.
By doing so, organizations can gain valuable insights into their operations and make data-driven decisions that improve their operations and bottom line.