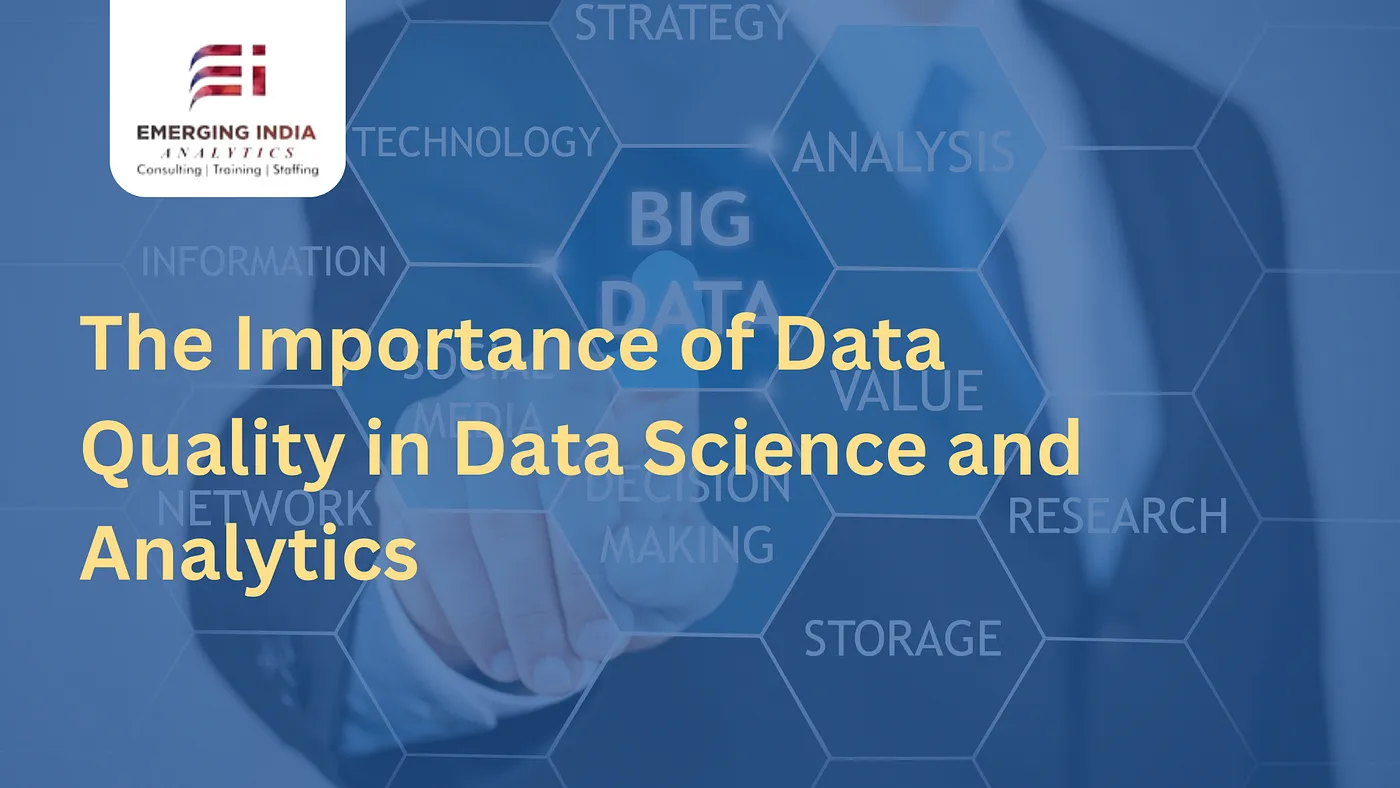
Introduction to Data Quality
Unlocking the full potential of data science and analytics requires more than just access to vast amounts of information. It hinges on a crucial factor that often goes overlooked — data quality. Picture this: you have all the ingredients for a mouthwatering dish, but if one ingredient is spoiled or contaminated, the entire meal is compromised. Similarly, in the realm of data science and analytics, poor data quality can undermine even the most sophisticated algorithms and models, rendering insights useless or even misleading. In this blog post, we will delve into why data quality is essential in driving accurate and actionable insights, explore its impact when neglected, discuss strategies for ensuring high-quality data, highlight tools and technologies that can assist in maintaining data quality standards, and outline best practices for effective data quality management. So fasten your seatbelts as we embark on an enlightening journey through the world of pristine datasets!
Why Data Quality is Essential in Data Science and Analytics
Data quality plays a crucial role in the field of data science and analytics. It refers to the accuracy, completeness, timeliness, consistency, and relevance of data. Without high-quality data, any analysis or insights derived from it would be unreliable and potentially misleading.
In the world of data science and analytics, decisions are made based on patterns and trends identified within datasets. If the underlying data is flawed or contains errors, these patterns may be distorted or inaccurate. This can lead to incorrect conclusions being drawn and ineffective strategies being implemented.
Poor data quality can have a wide range of negative impacts on businesses. For instance, it can result in missed opportunities for growth or cost savings as important insights may go unnoticed due to erroneous or incomplete information. Additionally, organizations may face compliance issues if their data does not meet regulatory standards.
To ensure high-quality data, various strategies should be adopted throughout the entire lifecycle of a dataset. This includes implementing robust validation processes during data collection to minimize errors at the source and conducting regular audits to identify and rectify any existing inconsistencies.
Several tools and technologies are available today that assist in maintaining good data quality. These include automated validation scripts that check for anomalies in real time as new information is added to databases. Data cleansing tools can also help identify duplicate entries or missing values that need attention.
It is essential for organizations to establish best practices for managing their data quality effectively. This involves clearly defining roles and responsibilities related to maintaining accurate datasets across different departments within an organization. Regular training programs should also be conducted to educate employees about proper procedures for handling sensitive information.
In conclusion (without using those words), ensuring high-quality data is vital for driving accurate insights that inform decision-making processes in both business operations and strategic planning initiatives alike.
The Impact of Poor Data Quality
Data is the lifeblood of any data science and analytics project. However, when the quality of data is compromised, it can have far-reaching consequences. Poor data quality can lead to inaccurate analysis and flawed insights, which in turn can undermine business decisions and strategies.
One major impact of poor data quality is decreased trust in the results generated from analytics projects. Decision-makers rely on accurate and reliable information to make informed choices, but if they cannot trust the data being presented to them, their confidence will be eroded.
Inaccurate or incomplete data also hinders effective predictive modeling. Machine learning algorithms require high-quality training datasets to generate accurate predictions. If these datasets are tainted with errors or inconsistencies, the models produced will yield unreliable results.
Poor data quality can also result in wasted time and resources for organizations. Data scientists and analysts may spend a significant amount of time cleaning and rectifying erroneous or duplicate records instead of focusing on extracting valuable insights from the data.
Moreover, poor data quality poses compliance risks for businesses operating in regulated industries such as finance or healthcare. Incomplete or incorrect customer information may lead to violations of privacy regulations or financial reporting standards.
Poor data quality has a cascading effect that impacts every aspect of an organization’s operations — from decision-making to resource allocation to regulatory compliance. It underscores the critical importance of ensuring high-quality data throughout the entire lifecycle of a project.
Strategies for Ensuring High-Quality Data
Maintaining high-quality data is essential for accurate and reliable insights in data science and analytics. To ensure the integrity of your data, consider implementing these effective strategies.
1. Establish Data Governance: Create a robust framework that outlines clear roles and responsibilities for managing data quality. This includes defining processes, standards, and guidelines to ensure consistent practices across the organization.
2. Implement Data Profiling: Conduct thorough profiling of your datasets to identify any inconsistencies or anomalies. By understanding the structure and content of your data, you can address issues such as missing values, duplicate entries, or incorrect formatting.
3. Enforce Data Validation: Put checks in place during data collection to validate its accuracy and completeness. Use automated validation techniques like regular expression matching or referential integrity constraints to catch errors at the source itself.
4. Cleanse Your Data: Utilize advanced cleansing techniques such as deduplication, standardization, and normalization to eliminate redundancies and inconsistencies within your dataset. This process ensures that you are working with clean and standardized information throughout your analysis.
5. Implement Data Monitoring: Regularly monitor incoming data streams for any anomalies or discrepancies by setting up alerts or dashboards that highlight potential issues in real-time.
Ensuring continuous monitoring helps maintain the quality of your datasets over time.
By following these strategies, you can significantly improve the quality of your data thereby enhancing the accuracy, reliability, and usefulness of insights derived from it.
Never underestimate the importance of maintaining high-quality data; it is a critical component that underpins successful decision-making and enables organizations to unlock valuable business intelligence
Tools and Technologies for Maintaining Data Quality
In the world of data science and analytics, maintaining high-quality data is crucial for accurate insights. Thankfully, there are various tools and technologies available that can help organizations ensure the integrity and reliability of their data.
One such tool is data profiling software, which allows businesses to assess the quality of their data by analyzing its structure, completeness, consistency, and validity. By identifying any anomalies or errors in the dataset, companies can take corrective measures to improve its quality.
Data cleansing tools are also essential for maintaining clean and accurate data. These tools automate the process of identifying duplicate records, correcting inconsistencies or inaccuracies in the dataset, and removing any irrelevant or outdated information. This ensures that decision-makers have access to reliable and up-to-date information when making strategic choices.
Another technology that plays a significant role in maintaining data quality is master data management (MDM) software. MDM solutions enable organizations to create a single source of truth by consolidating disparate datasets from various systems into a central repository. This not only improves efficiency but also ensures consistency and accuracy across different departments within an organization.
Moreover, advanced analytics platforms equipped with machine learning algorithms can help detect patterns or outliers in large datasets quickly. These algorithms can identify potential errors or inconsistencies based on historical trends or predefined rules set by analysts.
Additionally, technologies like blockchain offer promising opportunities for ensuring data integrity through distributed ledger systems. Blockchain’s decentralized nature eliminates the risk of tampering with sensitive information while providing transparency and traceability throughout the entire lifecycle of a transaction.
These tools and technologies play a vital role in maintaining high-quality data for effective decision-making in today’s rapidly evolving digital landscape. By investing in robust solutions that address both current needs and future scalability requirements,
organizations can enhance their analytical capabilities while driving innovation forward.
Best Practices for Data Quality Management
1. Establish Data Governance: Implementing a robust data governance framework is crucial for ensuring data quality. This involves defining clear roles and responsibilities, establishing processes and policies, and enforcing compliance with data standards.
2. Conduct Regular Audits: Regularly auditing your data helps identify any inconsistencies or errors that may be affecting its quality. By conducting thorough assessments, you can pinpoint areas of improvement and take corrective actions to maintain high-quality data.
3. Standardize Data Entry: Consistency is key when it comes to maintaining data quality. Establishing standardized formats for entering and storing data reduces the likelihood of errors or discrepancies creeping into the dataset.
4. Validate Incoming Data: Validating incoming data ensures that only accurate information enters your systems. Use automated validation techniques such as rule-based checks, range tests, and cross-field validations to catch any anomalies early on.
5. Cleanse and Correct Data: Implementing robust cleansing procedures helps remove duplicate records, correct inaccuracies, and eliminate irrelevant or outdated information from the dataset.
6. Monitor Data Quality Metrics: Keep track of key metrics such as completeness, consistency, accuracy, timeliness, relevance, and integrity to assess the overall health of your dataset continuously.
7. Provide Training & Awareness Programs: Educate employees about the importance of maintaining high-quality data through training programs on proper data handling practices.
8. Ensure Secure Storage & Access Controls: Protecting your datasets from unauthorized access safeguards their integrity while also complying with privacy regulations like GDPR or HIPAA.
By implementing these best practices consistently across your organization’s analytics workflows,data-driven decisions will become more reliable, enabling you to unlock valuable insights efficiently without compromising their accuracy
Conclusion: The Role of Data Quality in Driving Accurate and Actionable Insights
Data quality plays a crucial role in the success of data science and analytics projects. It is the foundation upon which accurate and actionable insights are built. Without high-quality data, organizations risk making misguided decisions that can have far-reaching consequences.
Poor data quality can lead to various negative impacts, including inaccurate analysis, biased results, wasted resources, missed opportunities, and damaged reputation. Businesses need to recognize the importance of maintaining data quality throughout its lifecycle.
To ensure high-quality data, organizations should adopt strategies such as implementing robust data governance policies, establishing clear processes for data collection and validation, regularly monitoring and cleansing their datasets, and leveraging advanced technologies like machine learning algorithms for automated error detection and correction.
Fortunately, there are several tools available in the market that can assist in maintaining data quality effectively. These range from simple software solutions for deduplication and standardization to more advanced platforms that offer comprehensive capabilities for profiling, cleansing, enriching, and integrating large volumes of structured or unstructured data.
In addition to using these tools and technologies effectively at each stage of the data pipeline — from ingestion to extraction to transformation — it is crucial to follow best practices for ongoing data quality management. This includes regular audits of processes and systems involved in handling valuable information assets within an organization.
By prioritizing strong governance practices like defining clear roles and responsibilities related to managing data quality issues within teams or departments while fostering a culture that values accuracy over speed or volume alone — organizations will be well-equipped to drive accurate insights through effective use of their analytical capabilities on top-quality datasets!
In conclusion, investing time and effort into ensuring excellent standards regarding your dataset’s integrity pays off tremendously when it comes to delivering reliable insights derived from sophisticated analysis techniques applied across vast amounts of raw but valuable organizational records!