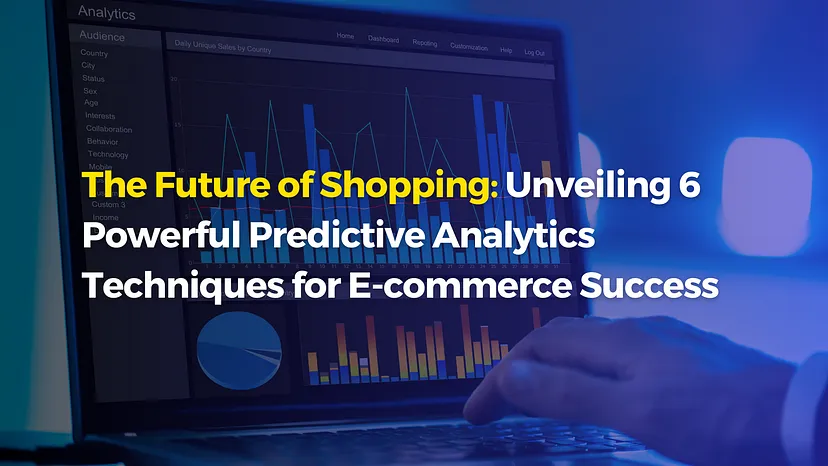
Introduction to predictive analytics in e-commerce
Welcome to the future of shopping! In today’s fast-paced digital world, e-commerce has become an integral part of our lives. With just a few clicks or taps, we can now purchase products and services from the comfort of our own homes. But what if there was a way to make this online shopping experience even more tailored and personalized? Enter predictive analytics — the game-changer that is revolutionizing the way businesses understand their customers and make informed decisions.
In this blog post, we will dive into the fascinating realm of predictive analytics in e-commerce. We will explore how analyzing data can help us gain valuable insights into customer behavior, allowing businesses to offer personalized recommendations and experiences. We’ll also uncover how demand forecasting can optimize inventory management, while dynamic pricing strategies ensure competitive advantage. And let’s not forget about fraud detection — where predictive analytics plays a vital role in keeping transactions secure.
But it doesn’t stop there! Artificial intelligence (AI) and machine learning are taking these techniques to new heights, enabling businesses to harness immense amounts of data for better decision-making. So get ready as we unveil six powerful predictive analytics techniques that are propelling e-commerce success like never before!
Are you excited? You should be because the future of shopping is here, powered by predictive analytics! So let’s jump right in and discover how these cutting-edge techniques are reshaping the e-commerce landscape with unparalleled precision and insight!
Understanding customer behavior through data analysis
Understanding customer behavior through data analysis is a game-changer for e-commerce businesses. By harnessing the power of predictive analytics, companies can gain valuable insights into their customers’ preferences, purchasing patterns, and even future buying behavior.
One key aspect of this analysis is collecting and analyzing data from various sources such as website traffic, social media interactions, and purchase history. This information provides invaluable insights into what customers want and how they engage with a brand. For example, by tracking user clicks on specific products or categories, businesses can determine which items are most popular and tailor their marketing efforts accordingly.
Furthermore, predictive analytics allows for segmenting customers based on demographics, psychographics, or past behaviors. This segmentation enables personalized marketing campaigns that resonate with individual consumers on a deeper level. By delivering tailored recommendations or promotions to each customer segment based on their unique preferences and interests, companies can significantly enhance the overall shopping experience.
Additionally, data analysis helps identify potential friction points in the customer journey. By pinpointing where shoppers drop off or abandon their carts during the checkout process — often called cart abandonment — businesses can optimize these touchpoints to increase conversions.
Overall,
understanding customer behavior through data analysis has become an essential tool for e-commerce success in today’s digital landscape.
By leveraging the power of predictive analytics techniques,
companies can stay one step ahead of customer needs
and deliver exceptional experiences that drive sales.
As technology continues to advance,
the possibilities for using data-driven insights are endless.
From personalization strategies to demand forecasting,
dynamic pricing tactics to fraud detection,
predictive analytics will continue to shape the future of shopping.
So embrace this powerful tool and unlock its full potential
to thrive in an increasingly competitive e-commerce market!
Personalization and recommendation engines
Personalization and recommendation engines are revolutionizing the way we shop online. With advancements in predictive analytics, e-commerce platforms are now able to provide personalized recommendations based on a customer’s browsing behavior, purchase history, and preferences.
One of the key benefits of personalization is that it enhances the overall shopping experience for customers. By analyzing their past interactions with the website, recommendation engines can suggest relevant products or services tailored specifically to their needs and interests. This not only saves time for shoppers but also increases the likelihood of making a purchase.
Furthermore, personalization helps businesses build stronger customer relationships by fostering a sense of individual attention. When customers feel understood and catered to, they are more likely to become loyal patrons who return for future purchases.
Recommendation engines use complex algorithms that continuously learn from user data to improve their accuracy over time. They take into account various factors such as product popularity, customer reviews, and similar user profiles when generating recommendations. This ensures that each suggestion is highly targeted and relevant.
In addition to enhancing the shopping experience for customers, personalization also benefits e-commerce businesses by increasing sales conversions. When users see products or services they are genuinely interested in, they are more likely to make a purchase rather than abandon their cart or leave without completing a transaction.
Personalization and recommendation engines play an integral role in shaping the future of e-commerce by providing customized experiences tailored specifically to each individual shopper’s preferences and needs. As technology continues to advance, these predictive analytics techniques will further evolve, paving the way for even more personalized online shopping experiences.
Demand forecasting for inventory management
Demand forecasting is a crucial aspect of inventory management for e-commerce businesses. By utilizing predictive analytics techniques, companies can gain valuable insights into customer behavior and accurately predict future demand patterns. This allows them to optimize their inventory levels, reduce stockouts, and minimize excess inventory.
One powerful technique used in demand forecasting is time series analysis. This involves analyzing historical sales data to identify patterns, trends, and seasonality. By understanding these patterns, businesses can make informed decisions about when and how much stock to order.
Another effective method is regression analysis, which takes into account various factors that may influence demand such as price changes or promotions. By analyzing the relationship between these variables and sales data, businesses can estimate the impact on future demand and adjust their inventory accordingly.
Machine learning algorithms are also widely used in demand forecasting for e-commerce. These algorithms analyze vast amounts of data including customer demographics, purchase history, browsing behavior, and external factors like weather or holidays. By leveraging this information, businesses can create accurate models that predict future demand with high precision.
Furthermore,
Predictive analytics plays a crucial role in helping e-commerce businesses effectively manage their inventories by accurately forecasting consumer demands.
By utilizing advanced statistical techniques like time series analysis and regression analysis; business owners can analyze past sales data along with other influential factors such as pricing strategies or promotional activities.
Moreover,
Machine learning algorithms have proven highly effective in predicting consumer demands by analyzing massive amounts of structured as well as unstructured data.
These algorithms take into consideration various parameters like customer preferences based on demographic information
This enables them to build sophisticated models capable of making precise predictions about future demands.
In conclusion,
By implementing predictive analytics techniques for demand forecasting purposes; e-commerce retailers will be able to optimize their inventories
They will be able to meet customer demands more efficiently while minimizing stockouts or overstock situations
This ultimately leads to improved operational efficiency; higher customer satisfaction rates; increased profitability
Dynamic pricing strategies using predictive analytics
Dynamic pricing strategies using predictive analytics have revolutionized the way e-commerce businesses operate. By leveraging advanced data analysis techniques, companies can now adjust prices in real-time based on various factors such as demand, competition, and customer behavior. This allows them to optimize their revenue and stay competitive in the ever-changing market.
One of the key benefits of dynamic pricing is its ability to maximize profitability. By analyzing historical sales data and current market trends, businesses can identify patterns that help determine optimal price points for different products or services. This ensures that they are not leaving money on the table by underpricing items or losing customers due to high prices.
Another advantage of dynamic pricing is its flexibility. With traditional fixed pricing models, businesses often struggle to find the right balance between attracting customers with low prices and maintaining profitability. However, with predictive analytics, companies can automatically adjust prices based on factors such as time of day, seasonality, competitor pricing, and even individual customer preferences.
Predictive analytics also enables businesses to effectively manage inventory and avoid overstocking or stockouts. By forecasting demand accurately using historical data and other relevant variables like promotions or events happening around a specific timeframe, companies can make informed decisions about stocking levels at any given time. This helps reduce costs associated with holding excess inventory while ensuring that popular items are always available for purchase.
Moreover, dynamic pricing strategies allow businesses to respond quickly to changes in market conditions or customer preferences. For example, if there is sudden surge in demand for a particular product due to external factors like a celebrity endorsement or social media buzz; predictive analytics algorithms can detect this trend early on and recommend adjusting the price accordingly before competitors catch up.
However it’s worth noting that implementing dynamic pricing strategies requires careful consideration of ethical implications as well as potential backlash from customers who may perceive fluctuating prices negatively.
Overall, dynamic pricing strategies using predictive analytics have proven to be a powerful tool for e-commerce businesses seeking to optimize their revenue,
Fraud detection and prevention in e-commerce
Fraud detection and prevention in e-commerce is a crucial aspect of ensuring the security and trustworthiness of online transactions. As technology advances, so do the methods used by fraudsters to deceive unsuspecting customers. That’s why predictive analytics techniques are becoming increasingly important in identifying and preventing fraudulent activities.
By analyzing large volumes of data, predictive analytics can help detect patterns and anomalies that may indicate fraudulent behavior. Machine learning algorithms can be trained to recognize these patterns, enabling e-commerce businesses to take proactive measures to prevent fraud.
One effective technique is using anomaly detection algorithms that flag unusual or suspicious transactions for further investigation. These algorithms analyze various factors such as transaction amounts, frequency, location, device information, and user behavior patterns to identify potential fraudulent activity.
Another powerful method is network analysis, which looks at connections between different users or devices within a network. By mapping out relationships and interactions among users, it becomes easier to spot any irregularities or suspicious links that could indicate fraudulent behavior.
Additionally, predictive analytics can help create risk models that assign scores or ratings to each transaction based on its likelihood of being fraudulent. By setting appropriate thresholds for these scores, businesses can automatically block high-risk transactions or trigger additional verification steps before completing the purchase.
Furthermore, machine learning models can continuously learn from new data and adapt their fraud detection capabilities over time. This allows businesses to stay one step ahead of fraudsters who constantly evolve their tactics.
In conclusion (without using those words!), predictive analytics techniques play a vital role in detecting and preventing fraud in e-commerce by leveraging advanced algorithms to analyze vast amounts of data quickly and accurately. By implementing robust fraud prevention strategies powered by predictive analytics technologies like anomaly detection, network analysis, risk modeling,
and continuous learning through machine learning models — online retailers can protect themselves from financial losses while ensuring a secure shopping experience for their customers!
The role of artificial intelligence and machine learning in predictive analytics for e-commerce success
Artificial intelligence (AI) and machine learning have revolutionized the way businesses operate, and e-commerce is no exception. In fact, these technologies play a pivotal role in driving predictive analytics for e-commerce success.
By leveraging AI algorithms and machine learning models, retailers can analyze vast amounts of data to gain valuable insights into consumer behavior. This allows them to understand customers on a deeper level, anticipate their needs, and deliver personalized shopping experiences.
One application of AI in e-commerce is recommendation engines. These powerful tools use customer data and patterns to suggest products or services that are likely to be of interest to individual shoppers. By tailoring recommendations based on past purchases or browsing history, retailers can increase customer engagement and boost sales.
Moreover, AI-powered demand forecasting enables accurate inventory management. By analyzing historical sales data alongside external factors such as weather conditions or seasonal trends, retailers can optimize their stock levels to meet customer demands while minimizing costs associated with overstocking or out-of-stock situations.
Dynamic pricing strategies also benefit from predictive analytics powered by AI and machine learning. Retailers can adjust prices in real-time based on various factors like market demand, competitor pricing analysis, or even individual customer behavior. This approach helps maximize revenue by offering competitive prices while maintaining profitability.
Furthermore, fraud detection has become more efficient with the integration of AI algorithms into e-commerce systems. Machine learning models learn from previous fraudulent activities and identify suspicious transactions in real-time using pattern recognition techniques. This proactive approach not only protects businesses but also enhances trust among customers.
The future of shopping lies in embracing the power of predictive analytics driven by artificial intelligence and machine learning. As technology continues to advance rapidly, it’s crucial for e-commerce businesses to harness these capabilities for better decision-making processes that lead to increased efficiency and improved customer satisfaction.
Conclusion: Embracing the future of shopping with predictive analytics
Conclusion: Embracing the future of shopping with predictive analytics
The world of e-commerce is constantly evolving, and staying ahead of the game is crucial for success. Predictive analytics offers a powerful toolset that can revolutionize how businesses operate in this digital landscape. By harnessing the power of data analysis, personalized recommendations, demand forecasting, dynamic pricing strategies, fraud detection, and artificial intelligence, e-commerce retailers can unlock new levels of efficiency and profitability.
Understanding customer behavior through data analysis allows businesses to tailor their offerings to meet individual preferences and needs. This level of personalization not only enhances the overall shopping experience but also increases customer satisfaction and loyalty.
Recommendation engines powered by predictive analytics algorithms take personalization a step further. By analyzing vast amounts of data such as browsing history, purchase patterns, and demographic information, these engines provide customers with relevant product suggestions which significantly increase the likelihood of conversion.
Demand forecasting plays a critical role in inventory management for e-commerce businesses. By utilizing predictive analytics techniques like time series modeling or machine learning algorithms on historical sales data along with external factors like holidays or promotions, retailers can optimize their stock levels to meet customer demands while minimizing overstocking or stockouts.
Dynamic pricing strategies enabled by predictive analytics allow businesses to adjust prices dynamically based on real-time market conditions such as supply-demand dynamics or competitor pricing. This ensures optimal price points for maximizing revenue without sacrificing competitiveness.
Fraud detection has become increasingly important in an era where online transactions are prevalent. Predictive analytics leverages advanced algorithms to detect patterns indicative of fraudulent activities before they cause significant damage to both customers and merchants alike.
Artificial intelligence (AI) and machine learning (ML) play pivotal roles in driving predictive analytics forward in e-commerce. Through AI-powered chatbots or virtual assistants integrated into websites or mobile apps, retailers can offer personalized assistance 24/7 while gathering valuable insights about customer preferences and pain points.