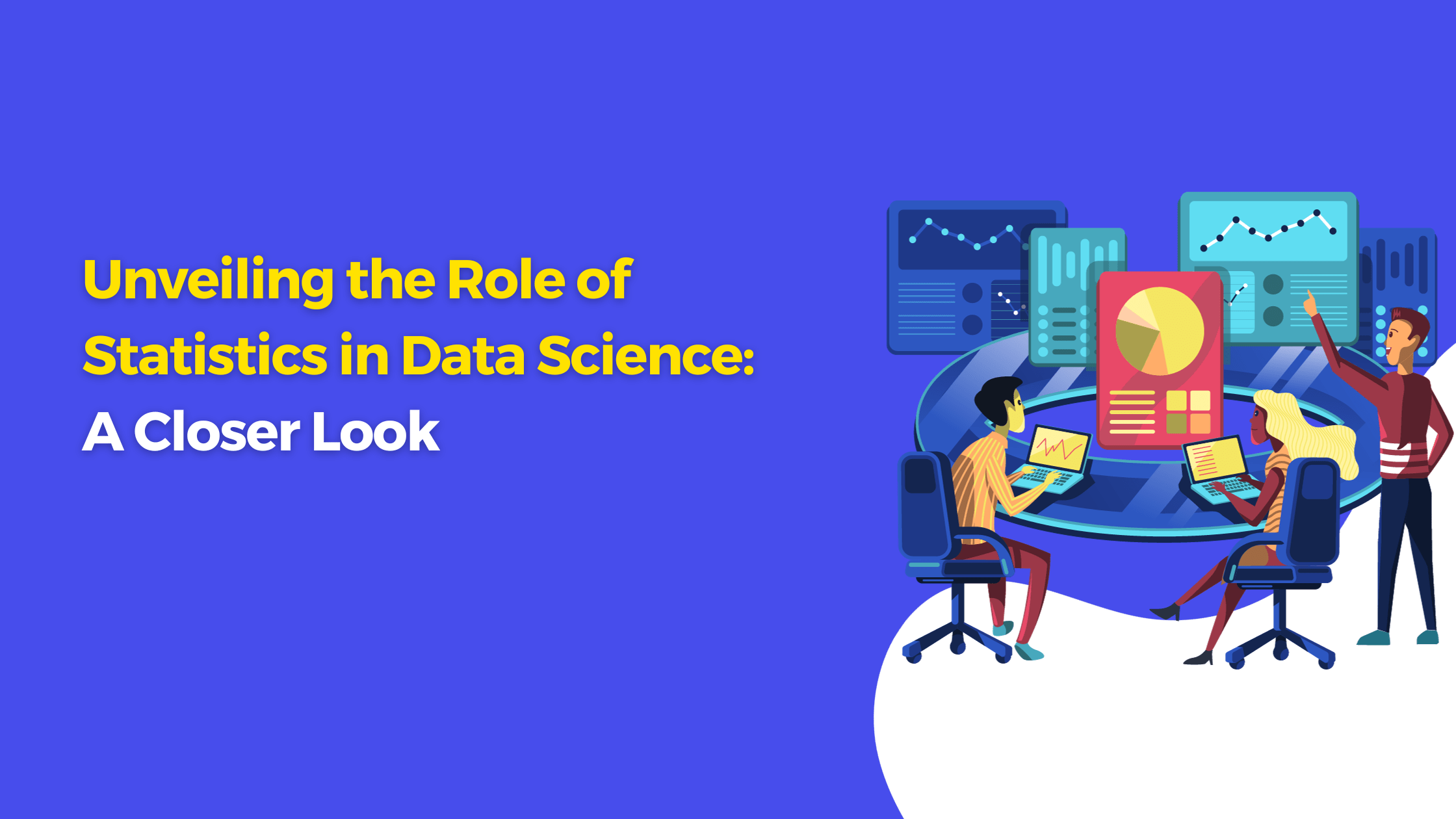
Introduction to Data Science
Welcome to the exciting world of data science, where numbers come alive and insights are unlocked! In today’s digital age, data has become a valuable asset that drives decision-making across industries. From predicting customer behavior to optimizing business operations, data science plays a pivotal role in transforming raw information into actionable knowledge.
But what exactly is data science? And why is it so important in our modern society? In this blog post, we will delve deep into the fascinating realm of statistics within data science and explore how it shapes our understanding of complex datasets. We’ll also compare data science with other related fields, discuss the challenges faced by data scientists, and highlight the numerous benefits it brings to businesses.
So buckle up as we embark on an illuminating journey through the intricate world of statistics within data science. Whether you’re a seasoned professional or just curious about this rapidly evolving field, there’s something here for everyone. Let’s begin unraveling the secrets behind statistical analysis in data science!
What is data science and why is it important?
Data science is the field that combines statistics, programming, and domain knowledge to extract valuable insights from data. It’s important because it helps businesses make informed decisions and drive innovation.
History and future of data science
Data science has come a long way since its inception, and its future looks promising. With advancements in technology and increasing reliance on data, the field is expected to continue growing and evolving.
Data science techniques and technologies
Data science employs a range of techniques and technologies to extract insights from data. These include machine learning, natural language processing, and big data analytics.
The Data Science Process
Obtaining and scrubbing data is the first step in the data science process. Exploring and modeling data helps uncover patterns, while interpreting and analyzing results provide valuable insights.
Obtaining and scrubbing data
Obtaining and scrubbing data is the crucial first step in the data science process. It involves collecting, cleaning, and organizing raw data to ensure accuracy and reliability for analysis.
Exploring and modeling data
Exploring and modeling data is a crucial step in the data science process. It involves digging deep into the dataset, identifying patterns, and building predictive models to extract valuable insights. The possibilities are endless!
Interpreting and analyzing results
Once the data has been explored and modeled, the next step is to interpret and analyze the results. This involves extracting insights and drawing conclusions from the data findings.
The Role of Statistics in Data Science
Data science relies on statistics to uncover patterns, make predictions, and provide insights. From descriptive to prescriptive analysis, statistics plays a crucial role in extracting meaning from data.
Descriptive analysis
Descriptive analysis is a crucial aspect of data science, providing insights into the characteristics and trends of data. It helps in summarizing and visualizing data to gain a better understanding.
Diagnostic analysis
Diagnostic analysis involves identifying patterns and relationships in data to understand the root causes of specific events or behaviors. It helps uncover insights and solutions for complex problems.
Predictive analysis
Predictive analysis plays a crucial role in data science, using historical data to make informed predictions about future outcomes. It helps businesses anticipate trends, optimize strategies, and make data-driven decisions for success.
Prescriptive analysis
Prescriptive analysis involves using data to make informed decisions and recommendations. It helps businesses optimize outcomes by providing actionable insights based on historical data and advanced algorithms.
Comparing Data Science with Other Fields
Data science is often compared to other fields like data analytics, business analytics, data engineering, machine learning, and statistics. Let’s explore the similarities and differences between these disciplines!
Data science vs. data analytics
Data science and data analytics are closely related but distinct fields. While data science focuses on extracting insights from data, data analytics is more focused on analyzing past trends and patterns.
Data science vs. business analytics
Data science and business analytics may seem similar, but they have distinct focuses. Data science emphasizes on algorithms and statistical models, whereas business analytics focuses on using data to make strategic decisions.
Data science vs. data engineering
Data science focuses on extracting insights from data, while data engineering is about building and maintaining the infrastructure to process and store large volumes of data. Both play crucial roles in the world of analytics.
Data science vs. machine learning
Data science and machine learning are closely related, but they have distinct differences. Data science encompasses a broader range of techniques, while machine learning focuses specifically on algorithms and models for making predictions or decisions.
Data science vs. statistics
While both fields deal with data, data science focuses on extracting insights and making predictions, while statistics primarily focuses on analyzing and interpreting data.
Challenges and Benefits of Data Science
Challenges faced by data scientists include handling large and complex datasets, ensuring data quality and privacy, and keeping up with the rapidly evolving technology. On the other hand, businesses can benefit from data science by gaining valuable insights for decision-making and improving operational efficiency.
Challenges faced by data scientists
Challenges faced by data scientists can range from managing large datasets to dealing with incomplete or inaccurate data. It requires constant learning and adapting to new techniques and technologies in this rapidly evolving field.
Benefits of data science for businesses
Data science offers numerous benefits for businesses, including improved decision-making, enhanced efficiency and productivity, better customer insights, and the ability to gain a competitive edge in the market.
Becoming a Data Scientist
Skills and qualifications required for becoming a data scientist include programming, statistics, machine learning, and problem-solving abilities. Learning resources such as online courses and tools like Python and R can help in acquiring these skills.
Skills and qualifications required
To become a data scientist, you need strong analytical skills, proficiency in programming languages like Python or R, and knowledge of statistics and machine learning algorithms.
Learning resources and tools
When it comes to learning data science, there are plenty of resources and tools available. From online courses to books and tutorials, you have many options to choose from.
Conclusion
In this fast-paced era of technology and information, data science has emerged as a powerful discipline that is transforming the way we analyze and interpret vast amounts of data. From businesses to healthcare, from finance to marketing, data science is playing a crucial role in driving decision-making processes and uncovering valuable insights.
Throughout this article, we have explored the intricacies of data science – its definition, techniques, and its relationship with statistics. We have delved into the various stages of the data science process, highlighting how statistics plays a pivotal role in each step – from obtaining and scrubbing data to interpreting and analyzing results. We have also compared data science with other related fields such as analytics, engineering, machine learning, and statistics itself.
Data scientists face numerous challenges on their journey towards extracting meaningful information from complex datasets. However, these challenges are outweighed by the tremendous benefits that businesses can derive from embracing data science. By leveraging statistical tools and techniques along with cutting-edge technologies like machine learning algorithms and artificial intelligence systems, organizations can gain actionable insights that drive growth and innovation.
If you aspire to become a successful data scientist yourself or wish to harness the power of this field for your business needs; acquiring relevant skills is paramount. Data scientists require a strong foundation in mathematics/statistics coupled with programming skills such as Python or R. Luckily there are plenty of online resources available today that provide comprehensive training programs aimed at equipping individuals with all necessary knowledge.