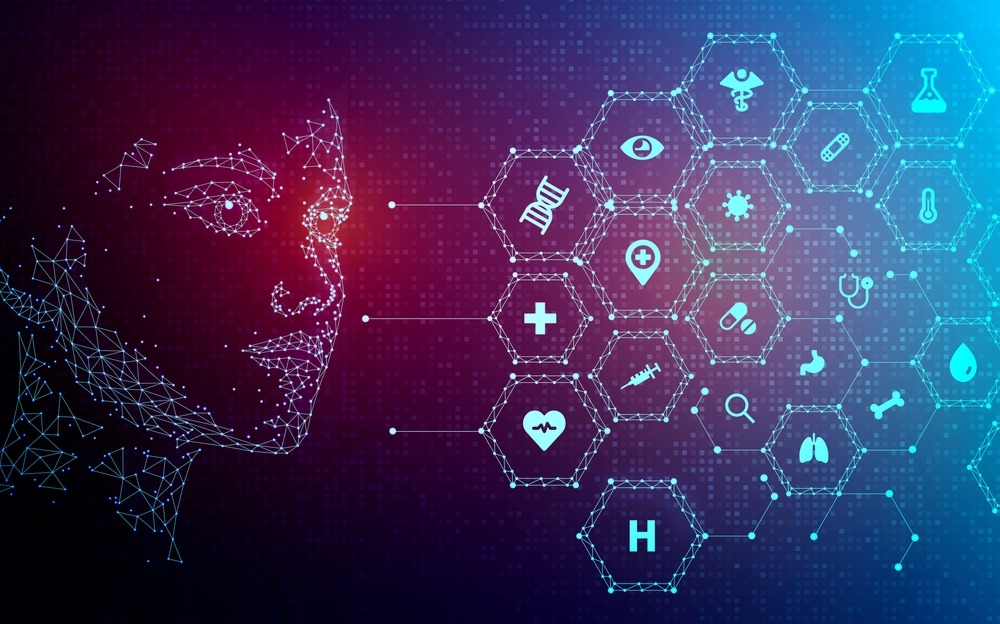
Machine learning has the potential to revolutionize the healthcare industry by improving patient outcomes, reducing costs, and accelerating medical research. The use of machine learning in healthcare can help in identifying patterns, predicting outcomes, and personalizing treatments. In this blog, we will discuss the applications of machine learning in healthcare and how it can be implemented to improve patient outcomes and medical research.
- Predictive Analytics for Early Disease Detection
Machine learning can help in early disease detection by analyzing patient data and identifying potential risk factors. It can analyze vast amounts of data such as medical records, genomics, and imaging to identify patterns and predict the likelihood of developing a disease. Early detection can help in developing personalized treatment plans, reducing healthcare costs, and improving patient outcomes.
- Personalized Treatment Plans
Machine learning algorithms can analyze patient data to create personalized treatment plans based on their individual characteristics. It can help in identifying the most effective treatment options and predicting the outcome of different treatment plans. Personalized treatment plans can improve patient outcomes and reduce the risk of adverse events.
- Drug Discovery and Development
Machine learning can help in drug discovery and development by analyzing vast amounts of data and identifying potential drug candidates. It can help in identifying molecular targets, predicting drug efficacy, and optimizing drug dosages. Machine learning can also help in reducing the time and cost of drug development, which can improve patient access to new treatments.
- Medical Image Analysis
Machine learning can help in medical image analysis by automating the process of image analysis and identifying patterns that are not visible to the human eye. It can help in detecting tumors, identifying abnormalities, and predicting disease progression. Medical image analysis can help in improving the accuracy of diagnoses and reducing the time required for manual image analysis.
- Clinical Decision Support Systems
Machine learning can help in clinical decision support systems by analyzing patient data and providing real-time recommendations to healthcare providers. It can help in identifying potential risks, predicting outcomes, and recommending the most effective treatments. Clinical decision support systems can help in reducing medical errors, improving patient outcomes, and reducing healthcare costs.
Implementing Machine Learning in Healthcare
- Implementing machine learning in healthcare requires collaboration between data scientists, healthcare providers, and patients. Here are some best practices for implementing machine learning in healthcare:
- Identify the Problem: Identify the specific problem that you want to solve with machine learning. It could be early disease detection, personalized treatment plans, or medical image analysis.
- Collect and Clean Data: Collect and clean data from various sources such as medical records, genomics, and imaging. The data should be of high quality and relevant to the problem you are trying to solve.
- Choose the Right Algorithm: Choose the right machine learning algorithm that is suited for the problem you are trying to solve. There are various types of algorithms such as supervised learning, unsupervised learning, and reinforcement learning.
- Train and Test the Model: Train and test the machine learning model using the collected data. The model should be trained using a sufficient amount of data to ensure accuracy.
- Monitor and Update the Model: Machine learning models should be monitored and updated regularly to ensure accuracy and relevance. The model should be updated with new data as it becomes available.
Conclusion
Machine learning has the potential to revolutionize the healthcare industry by improving patient outcomes, reducing costs, and accelerating medical research. The implementation of machine learning in healthcare requires collaboration between data scientists, healthcare providers, and patients. It is important to identify the specific problem that you want to solve, collect and clean data, choose the right algorithm, train and test the model, and monitor and update the model regularly. By implementing machine learning in healthcare, we can improve patient outcomes and advance medical research.