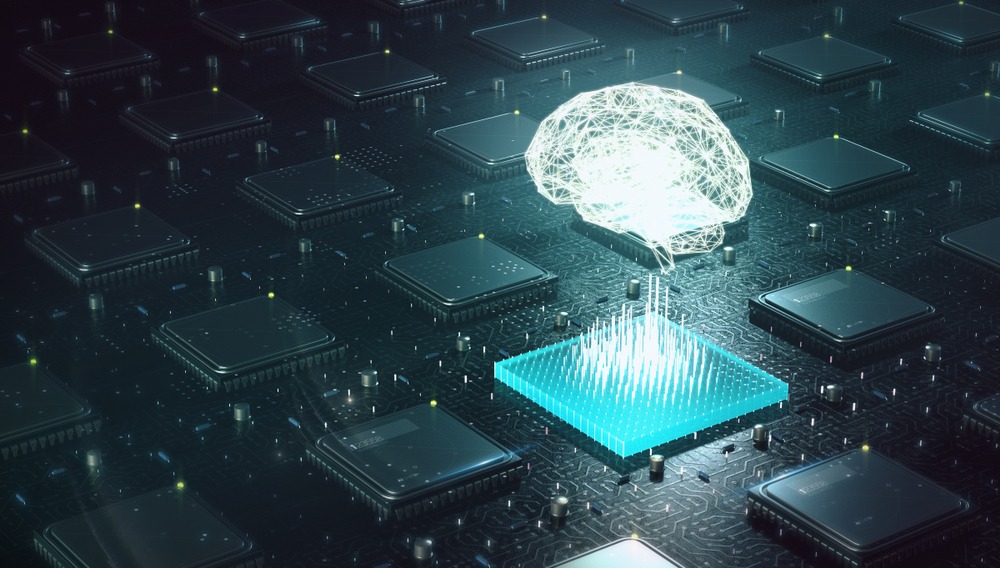
Welcome to the dawn of a new era – an era where machines are becoming smarter than humans. We’re not talking about mere sci-fi here, but rather the exciting and rapidly-evolving world of Artificial Intelligence (AI). Machine Learning is driving this revolution, enabling computers to learn from data without being explicitly programmed. In this blog post, we’ll explore how Machine Learning is shaping the future of AI and what it means for our society as a whole. So buckle up and get ready to dive into the fascinating realm of Beyond Human Intelligence!
Introduction: What is Machine Learning?
Machine learning is a subset of artificial intelligence (AI) that focuses on the ability of machines to learn from data and improve their performance over time. Machine learning algorithms are able to automatically detect patterns in data and use them to make predictions or recommendations.
Machine learning is a rapidly growing field with many applications in areas such as computer vision, natural language processing, and robotics. In recent years, machine learning has been used to develop self-driving cars, facial recognition systems, and spam filters.
There are three main types of machine learning: supervised learning, unsupervised learning, and reinforcement learning. Supervised learning algorithms are trained on a dataset that includes both input data and desired output labels. Unsupervised learning algorithms are not given any desired output labels; instead, they try to find structure in the data itself. Reinforcement learning algorithms interact with an environment in which they can receive feedback about their actions in order to learn what works and what doesn’t work.
Machine learning is a powerful tool that is shaping the future of artificial intelligence.
History of AI and Machine Learning
The history of AI and machine learning is full of milestones and breakthroughs that have led to the development of today’s sophisticated AI systems. The field of AI research was founded in 1956 at a now-famous conference at Dartmouth College, where participants laid out the ambitious goal of “devising methods for making computers think.”
In the years since, there have been many impressive achievements in AI, including early successes in artificial intelligence applications such as expert systems, computer chess playing, and natural language processing. However, it wasn’t until the late 1990s and early 2000s that machine learning began to gain real traction, thanks to advances in computation power and data storage capacity.
Today, machine learning is widely recognized as a key technology for powering artificial intelligence applications. Machine learning algorithms are used to automatically improve the performance of AI systems by increasing their accuracy and efficiency. And as machine learning techniques continue to evolve, so too does the potential for AI to transform our world in ways we can only imagine.
How Machine Learning is Changing the Game for Artificial Intelligence
Artificial intelligence (AI) is no longer a new concept. It’s been around for decades, with early successes in fields like chess and voice recognition. But AI is changing. Thanks to recent advances in machine learning, AI is becoming more powerful and flexible than ever before.
Machine learning is a type of AI that allows computers to learn from data, without being explicitly programmed. This means that machine learning can enable computers to improve their performance on tasks over time. For example, Google’s AlphaGo program used machine learning to teach itself how to play the ancient game of Go, and ultimately beat a world champion human player.
Machine learning is also playing a big role in the development of self-driving cars. By feeding data about traffic patterns and road conditions into a machine learning algorithm, autonomous vehicles can learn to navigate roads safely without human input.
The possibilities for machine learning are endless. And as machine learning techniques continue to improve, so too will the capabilities of artificial intelligence.
Benefits of Machine Learning for AI
Machine learning is a field of artificial intelligence (AI) that focuses on the development of computer programs that can access data and use it to learn for themselves. The ultimate goal of machine learning is to create algorithms that can automatically improve given more data.
Machine learning is closely related to and often overlaps with computational statistics; a discipline which also focuses on prediction-making through the use of computers. It has strong ties to mathematical optimization, which delivers methods, theory and application domains to the field. Machine learning is sometimes conflated with data mining, where the latter sub-field focuses more on exploratory data analysis and is known as unsupervised learning.
Statistical learning theory provides a mathematical framework for machine learning, with generalization error bounds for nonlinear functions achieved by using kernel methods. These kernel methods can be used to solve optimization problems in machine learning such as finding the maximum-margin hyperplane in support vector machines (SVMs). Other optimization techniques used in machine learning include decision trees, evolutionary programming, artificial neural networks and inductive logic programming.
Examples of AI & Machine Learning Projects
- Google’s AlphaGo: In 2016, Google’s AlphaGo made history by becoming the first AI system to beat a professional human player at the game of Go.
- IBM Watson: IBM’s Watson is a cognitive computing system that has been used in a variety of different applications, from healthcare to finance.
- Microsoft Azure: Microsoft Azure is a cloud computing platform that offers a variety of services, including machine learning and artificial intelligence.
- Amazon Machine Learning: Amazon Machine Learning is a service that allows developers to build predictive models using data from Amazon’s vast online ecosystem.
- TensorFlow: TensorFlow is an open source software library for machine learning that was developed by Google Brain.
Challenges and Limitations of Machine Learning
Despite its many successes, machine learning still faces a number of challenges and limitations. One challenge is that machine learning algorithms require a large amount of data in order to learn effectively. This can be a problem when trying to learn from small datasets, or when trying to learn from data that is non-uniform (e.g., images or text). Another challenge is that machine learning models can be difficult to interpret, making it hard to understand why the model made a particular prediction. This can be a problem when trying to diagnose errors or improve the model. Machine learning models are often highly sensitive to changes in the data (e.g., a slight change in the input data can cause a completely different output from the same model). This sensitivity can make it difficult to deploy machine learning models in real-world settings.
Conclusion
The future of AI is in the hands of machine learning and its ability to process vast volumes of data quickly and accurately. We have only scratched the surface on what can be achieved with this technology, but it promises to revolutionize our lives in ways we never thought possible. From faster decision-making processes, improved healthcare outcomes, safer autonomous vehicles, and more efficient production lines – machine learning has the potential to transform many aspects of our lives for the better. With continued improvements in algorithms and computer hardware, we may soon realize a world where AI is an integrated part of our daily lives.